Unveiling The Secrets Of The Anova Table: A Comprehensive Guide To Data Analysis
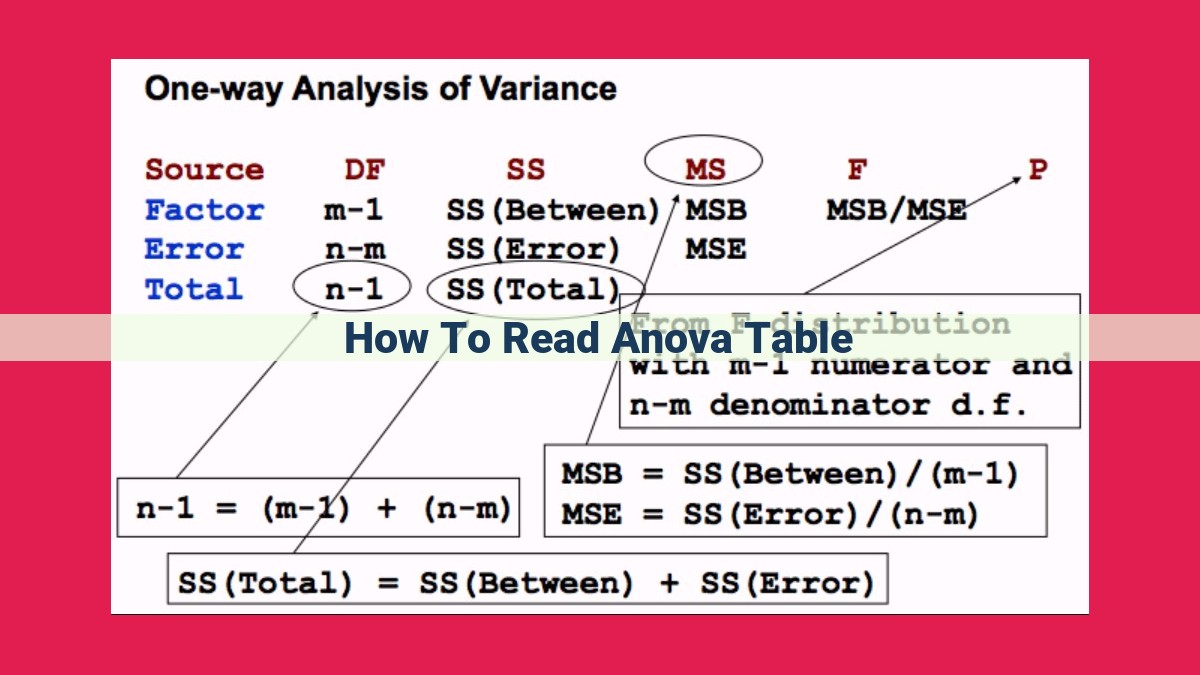
To read an ANOVA table, identify the source of variation (e.g., groups) and their degrees of freedom. Calculate the sum of squares within each group and the mean square between them. Calculate the F-statistic to assess group differences, comparing it to the F-critical value obtained from the F-distribution. The p-value indicates the probability of chance effects and determines statistical significance.
ANOVA Table: Unraveling Statistical Insights
In the realm of statistics, Analysis of Variance (ANOVA) stands tall as a statistical powerhouse, illuminating patterns and unveiling hidden insights from data. ANOVA Table, the cornerstone of ANOVA analysis, serves as a roadmap, guiding us through the complexities of statistical comparisons.
Understanding ANOVA’s Purpose
Imagine you’re conducting an experiment to test the effectiveness of different fertilizers on plant growth. ANOVA allows you to compare multiple groups (fertilizers) and determine whether their means are significantly different. By breaking down the variation in the data, ANOVA pinpoints sources of variation, identifying the experimental factors that contribute to the observed differences.
Delving into ANOVA’s Components
The ANOVA Table is a veritable treasure trove of statistical information, composed of several key components:
- Source of Variation: This section identifies the independent (manipulated) variable (fertilizer types) and dependent (outcome) variable (plant height).
- Degrees of Freedom: These values represent the flexibility of the sample, indicating the number of independent data points used in each group.
- Sum of Squares: This measure quantifies the variability within each group, capturing the spread of data points around their respective means.
- Mean Square: Mean square assesses the variability between groups, revealing how much the groups differ from each other.
- F-statistic: This test statistic is calculated by dividing the mean square between groups by the mean square within groups, providing a measure of the significance of the group differences.
Interpreting the Results
The p-value plays a pivotal role in ANOVA interpretation. It represents the probability of obtaining the observed results if there were no real group differences. A low p-value (<0.05) suggests that the observed differences are unlikely to have occurred by chance alone, indicating statistically significant group differences.
The F-critical value serves as a benchmark for comparison. If the F-statistic exceeds the F-critical value, it further strengthens the evidence of significant group differences.
Assessing Significance and Error
ANOVA analysis is not without its limitations. It’s essential to acknowledge the potential for Type I error (incorrectly rejecting the null hypothesis) and Type II error (failing to reject a false null hypothesis). Confidence intervals provide a range of plausible values for the true group differences, offering a more nuanced interpretation.
The ANOVA Table is an invaluable tool, empowering researchers to delve into the intricacies of statistical comparisons. By understanding its components and interpreting the results, we can unlock valuable insights from our data, informing decision-making and advancing our understanding of complex phenomena.
Unveiling the Source of Variation: Setting the Stage for ANOVA
In the realm of statistical analysis, ANOVA (Analysis of Variance) emerges as a powerful tool to uncover meaningful patterns and differences within datasets. Embarking on this statistical journey, we begin by identifying the crucial components that form the foundation of ANOVA: the independent and dependent variables.
The independent variable, also known as the manipulated variable, represents the factor that we intentionally control or manipulate in an experiment or study. It is the variable that we believe has an influence or effect on the outcome of interest.
The dependent variable, or outcome variable, is the variable that we measure or observe as a result of changes in the independent variable. It is the variable that we aim to predict or understand based on the values of the independent variable.
In experimental design, the different levels or categories of the independent variable define the groups that are being compared. For instance, in an experiment examining the effects of fertilizer on plant growth, the fertilizer type could be the independent variable, with different fertilizer treatments representing the groups.
Understanding the experimental design and group assignments is paramount for interpreting ANOVA results. By clearly defining these elements, we establish a framework for assessing the variability within and between groups, which forms the cornerstone of ANOVA analysis.
Degrees of Freedom: Defining the Sample’s Flexibility
Imagine yourself at a party filled with laughter and lively conversations. As you mingle with different groups, you notice that some ignite more engaging discussions than others. This phenomenon, in statistical terms, is reflected in the concept of degrees of freedom.
In the realm of statistics, degrees of freedom (df) measure the number of independent pieces of information available in a sample. Just as a lively party conversation thrives on the contributions of diverse perspectives, statistical analyses rely on the availability of independent data points to draw meaningful conclusions.
Calculating Degrees of Freedom
For a single-sample t-test, df is calculated as the sample size (n) minus 1:
df = n - 1
In a two-sample t-test, df is calculated as the sum of the degrees of freedom of each sample minus 2:
df = (n1 - 1) + (n2 - 1) = n1 + n2 - 2
Importance for Statistical Significance
Degrees of freedom play a crucial role in interpreting statistical significance. A small df increases the likelihood of obtaining a significant result even when there is little or no actual difference between groups. Conversely, a large df makes it harder to achieve statistical significance, ensuring that significant results are less likely to be false positives.
Example
Suppose you conduct an experiment with two groups, each containing 10 participants. The df for this experiment is 18 (10+10-2). If you obtain an F-statistic of 3.5, the corresponding p-value with df = 18 is 0.08. This result is not statistically significant, suggesting that the observed difference between groups could be due to chance.
However, if you increase the sample size to 50 participants per group, the df becomes 98. The same F-statistic of 3.5 now yields a p-value of 0.0001, indicating a highly significant result. The larger df has reduced the likelihood of a false positive conclusion.
Understanding degrees of freedom is essential for correctly interpreting statistical results. A clear grasp of df helps researchers assess whether their findings represent genuine differences or are merely statistical noise. By carefully considering degrees of freedom, you can make informed decisions about the significance of your experimental outcomes.
Unveiling Variability Within Groups: The Sum of Squares
In the realm of statistical analysis, the ANOVA table serves as a window into the intricate world of statistical insight. Among its key components is the sum of squares, a measure that unveils the variability within groups. By delving into the sum of squares, we gain a deeper understanding of data dispersion and the spread of values within each group in our analysis.
The sum of squares is essentially a measure of how much the data points within a group deviate from the group’s mean. This deviation is known as variance, which is a crucial aspect in understanding the spread of data. To calculate the sum of squares, we square the differences between each data point and the group mean and then add up these squared differences. The resulting value provides a quantitative measure of variability within that particular group.
The standard deviation, closely related to the sum of squares, is a statistical tool that helps us make meaningful interpretations of variability. It measures the spread of data around the mean and is calculated by taking the square root of the variance. A smaller standard deviation indicates that the data points are closely clustered around the mean, while a larger standard deviation suggests more dispersion.
Understanding the sum of squares and standard deviation is essential for assessing the homogeneity of groups in our analysis. If the sum of squares and standard deviation are small for a particular group, it indicates that the data points are tightly clustered around the group mean, suggesting little variability. Conversely, larger values of these measures indicate greater spread and variability within the group.
By examining the sum of squares and standard deviation for each group, we gain valuable insights into the internal dynamics of our data. These measures provide a foundation for further statistical comparisons and help us make informed conclusions about the relationships and differences between groups in our study.
Mean Square: Unveiling the Variation Between Groups
In the realm of statistics, the ANOVA table provides a window into the intricate dance of data, revealing the variability within and between experimental groups. Mean square is a crucial component of this statistical tapestry, shedding light on the differences that truly matter.
Picture this: you’re conducting an experiment to test the effects of different fertilizers on plant growth. After meticulous measurements, you have a table filled with data representing the heights of plants in each treatment group. To unravel the story behind these numbers, you embark on the ANOVA journey.
The sum of squares captures the total variability within each group. However, to compare the variability between groups, we need a more nuanced measure. Enter mean square, which is calculated by dividing the sum of squares by the corresponding degrees of freedom.
Degrees of freedom represent the flexibility within the data, taking into account the sample size and the number of groups. By factoring in this flexibility, mean square provides a more precise estimate of the variability due to the experimental treatments.
Now, the fun begins! By comparing the mean squares between groups, we can see if there are significant differences in variability. A large mean square indicates more variability between groups, suggesting that the treatments are having an effect. On the other hand, a small mean square suggests that the treatments are not creating much of a stir.
Interpreting mean square is like deciphering a code, revealing the true nature of the group differences. It allows us to determine which treatments are truly driving the observed variation and which ones are simply noise.
So, the next time you find yourself gazing at an ANOVA table, remember the power of mean square. It’s the key to unlocking the secrets of variability between groups, paving the way for meaningful conclusions and data-driven insights.
F-statistic: Putting Variability to the Test
- Introducing the F-distribution and its role in hypothesis testing.
- Calculating the F-statistic to test for significant group differences.
**F-statistic: Putting Variability to the Test**
Imagine you’re hosting a cooking competition between three chefs, each with a unique recipe. After the dishes are tasted and judged, you’re curious to know if there are significant differences in their culinary skills. Enter the mighty F-statistic, a statistical tool that can help you uncover the hidden truths about these chefs’ performances.
The F-statistic is a magical number that measures the ratio of the variability between groups (the chefs) to the variability within groups (the individual dishes created by each chef). It’s akin to a sensitive scale that tips in favor of groups that stand out in terms of their average performance.
To calculate the F-statistic, we delve into the F-distribution, a statistical bell curve that describes the expected distribution of F-values under the assumption of no real differences between groups. We then compare our calculated F-statistic to the critical value from the F-distribution, which tells us the threshold beyond which we can reject the null hypothesis of no differences.
If the F-statistic surpasses the critical value, like a superhero leaping over a hurdle, it signals that the variability between groups is significantly greater than the variability within groups. This suggests that our chefs are not equally skilled, supporting our hypothesis that they possess distinct culinary abilities.
Conversely, if the F-statistic falls short of the critical value, it’s akin to a detective failing to find any solid evidence. In this case, the variability between groups is not significantly higher than the variability within groups, indicating that our chefs’ performances are relatively comparable.
By leveraging the F-statistic, we can make informed decisions about our chefs’ cooking abilities. It’s an invaluable tool in the world of statistics, helping us understand the nuances of data and draw meaningful conclusions. So, next time you’re faced with a statistical puzzle, don’t hesitate to call upon the F-statistic; it will guide you towards the truth about variability, one dish at a time.
p-value: The Probability of Chance
- Defining the p-value and its significance in determining statistical significance.
- Setting a significance level and interpreting p-values accordingly.
p-value: Unveiling the Probability of Chance
In the realm of statistics, the p-value holds a pivotal role in determining statistical significance. It represents the probability of obtaining results as extreme as those observed, assuming the null hypothesis is true. Imagine rolling a fair six-sided die. The probability of rolling a particular number is 1/6. However, if you rolled a six five times in a row, you might question whether the die is fair.
Setting a Significance Level: The Threshold of Doubt
Scientists establish a significance level (usually 0.05 or 0.01) before conducting a statistical test. This level represents the probability threshold below which they reject the null hypothesis. In other words, if the p-value is lower than the significance level, it suggests that the observed results are unlikely to have occurred by chance.
Interpreting p-values: Signaling Statistical Significance
A low p-value (less than the significance level) indicates that the observed differences are unlikely to have been caused by random chance. This allows researchers to reject the null hypothesis and conclude that there is a statistically significant difference between groups. A p-value above the significance level, on the other hand, fails to provide sufficient evidence to reject the null hypothesis.
The Perils of Error: Type I and Type II Missteps
Statistical inference always carries the risk of error. Type I error occurs when researchers reject the null hypothesis when it is actually true (false positive). Type II error occurs when researchers fail to reject the null hypothesis when it is false (false negative). P-values help researchers minimize the risk of Type I error by setting a strict significance level.
Confidence Intervals: Estimating the True Difference
Confidence intervals provide a range of values within which the true population difference is likely to fall. Researchers can calculate confidence intervals based on the p-value and the sample size. A narrow confidence interval indicates a more precise estimate, while a wider interval suggests greater uncertainty.
By understanding the p-value and its role in statistical significance, researchers can make more informed decisions about their findings. It helps them avoid exaggerated claims and ensures that their conclusions are supported by credible evidence.
F-Critical Value: The Gateway to Statistical Thresholds
In the realm of statistical hypothesis testing, the ANOVA table illuminates a path towards understanding group differences. One crucial aspect of this analysis is the F-critical value, a threshold that separates statistical significance from chance.
Just as a lock requires a key to open, hypothesis testing requires a critical value to determine if the observed differences between groups are mere fluctuations or indicate meaningful disparities. The F-critical value is that key, derived from the significance level and the F-distribution.
The significance level, typically set at 0.05, represents the maximum probability of rejecting the null hypothesis (assuming no group differences) when it is actually true. In other words, it’s the risk we’re willing to take of making a false positive conclusion.
Armed with the significance level, we delve into the F-distribution, a bell-shaped curve that describes the distribution of F-statistics when the null hypothesis is true. The F-statistic, calculated from the ANOVA table, represents the ratio of variability between groups to variability within groups. If this ratio is sufficiently large, it suggests that the observed differences are unlikely to have occurred by chance alone.
To establish the threshold for rejection, we locate the F-critical value on the F-distribution, where the probability of obtaining a larger F-statistic under the null hypothesis is equal to the significance level. For instance, if the significance level is 0.05 and the degrees of freedom are known, we can consult an F-distribution table or use software to find the corresponding F-critical value.
Comparing the F-statistic to the F-critical value is like weighing two values on a balance scale. If the F-statistic exceeds the F-critical value, it indicates that the observed differences between groups are statistically significant, meaning it is unlikely that they occurred by chance. In this case, we reject the null hypothesis and conclude that the groups differ meaningfully.
On the other hand, if the F-statistic falls below the F-critical value, it suggests that the observed differences could reasonably have occurred by chance. In this scenario, we fail to reject the null hypothesis and conclude that there is no statistically significant difference between the groups.
Thus, the F-critical value serves as a gatekeeper, allowing us to interpret statistical results with confidence. It ensures that our conclusions are grounded in empirical evidence and minimizes the risk of making false judgments about group differences.
Significance and Error: Unveiling the Truth
Understanding the results of ANOVA is crucial in drawing meaningful conclusions. Statistical significance emerges from comparing the F-statistic to a critical value, revealing whether group differences are due to chance or meaningful variations. However, acknowledging potential errors is equally important.
Demystifying Statistical Errors
Two types of errors can arise in statistical inference:
- Type I error (false positive): Rejecting a true null hypothesis (concluding a difference when none exists).
- Type II error (false negative): Failing to reject a false null hypothesis (missing a real difference).
Maintaining a balance between these errors is essential. By setting an appropriate significance level (α), we control the risk of Type I errors. Typically, a level of 0.05 (5%) is used, meaning a 5% chance of finding a significant result when no actual difference exists.
Unveiling True Differences: Confidence Intervals
Beyond statistical significance, confidence intervals provide a range of plausible values for true group differences. These intervals are calculated based on the sample data and the chosen significance level. They indicate the likelihood that the true difference falls within a specific range.
Narrower confidence intervals suggest more precise estimates, while wider intervals indicate greater uncertainty. Interpreting confidence intervals helps us better understand the practical significance of group differences and their variability.
In summary, ANOVA provides valuable insights into the presence of significant group differences. However, acknowledging potential statistical errors and utilizing confidence intervals ensures that our conclusions are both accurate and meaningful. Embracing this approach empowers us to make informed decisions based on solid statistical evidence.
Error Term: Accounting for Uncertainty
In the realm of statistics, the ANOVA table provides a comprehensive snapshot of data variability and significance. However, it’s essential to acknowledge that even the most meticulously collected data can harbor unexpected fluctuations and discrepancies. This is where the concept of error term comes into play.
The error term, also known as residual variance, captures the portion of variability in the data that cannot be attributed to the independent variables. It represents the random error inherent in any measurement process. This error can stem from various sources, such as individual differences, measurement noise, or uncontrollable environmental factors.
Understanding the error term is crucial because it estimates the uncertainty associated with statistical inferences. When we calculate the p-value and confidence intervals, we are essentially assessing the probability of making incorrect conclusions due to random error. By incorporating the error term into our statistical models, we can account for this uncertainty and make more accurate predictions and inferences.
The error term serves as a reminder that even in carefully controlled experiments, there will always be some degree of variability that cannot be explained by the independent variables. It is this unexplained variability that the error term attempts to capture. By understanding and accounting for the error term, we can draw more reliable conclusions from our statistical analyses.