Unlocking Relationships: The Importance Of Correlation In Data Analysis
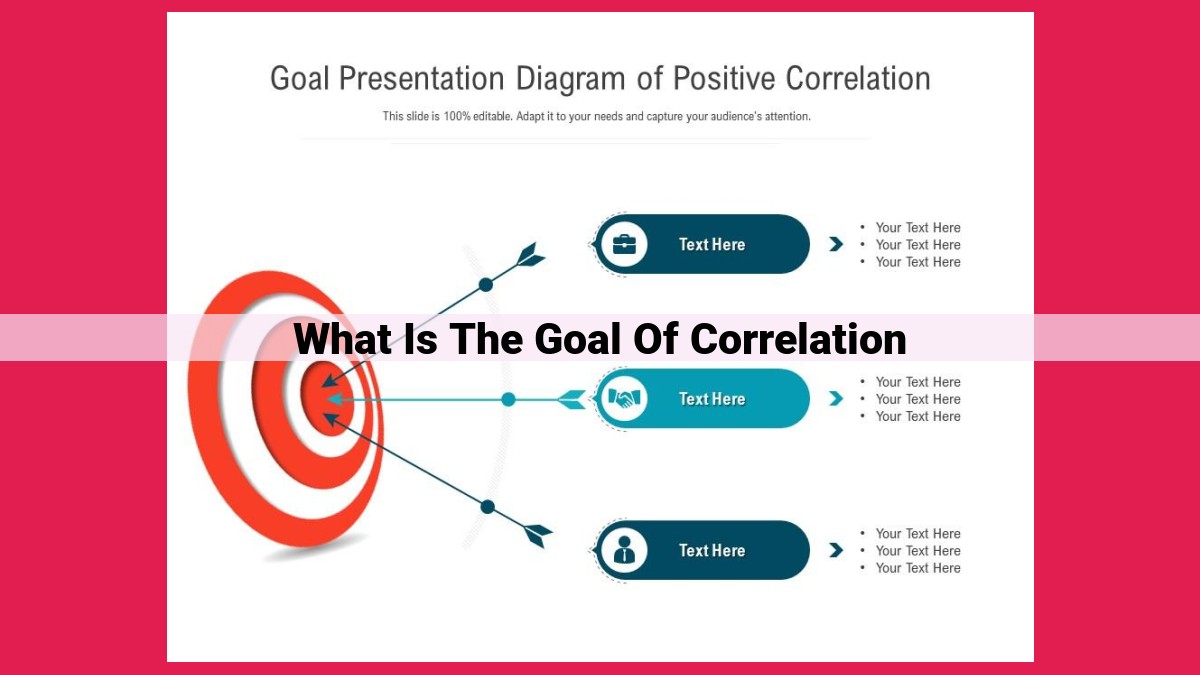
Correlation’s goal is to measure the strength and direction of relationships between variables. It aids in identifying significant relationships and determining the presence of positive or negative associations. Correlation allows for predictions of one variable based on another, aiding in regression analysis and forecasting. While it cannot establish causality alone, it provides initial evidence of potential causal relationships. Statistical significance and hypothesis testing help distinguish meaningful relationships from random occurrences, while controlling for confounding variables prevents biased correlations. Correlation plays a crucial role in data structure analysis, uncovering patterns and relationships within complex data sets.
The Essence of Correlation
- Define correlation and its purpose in statistical analysis
- Explain how it measures the strength and direction of relationships between variables
Unveiling the Essence of Correlation: A Comprehensive Guide
In the world of data analysis, correlation plays a pivotal role, unveiling hidden relationships and providing insights into complex phenomena. It’s a statistical tool that measures the strength and direction of associations between variables. By examining correlations, we gain a deeper understanding of how different factors interact and influence each other.
Imagine you’re analyzing sales data for a retail company. You notice a positive correlation between sales revenue and marketing expenses. This suggests that as the company spends more on marketing, sales revenue tends to increase. By quantifying this relationship, you can predict future sales revenue based on planned marketing investments.
Conversely, a negative correlation might indicate an inverse relationship. For instance, if you observe a negative correlation between customer satisfaction and product price, it implies that as product prices go up, customer satisfaction tends to decline. This information can guide pricing strategies to balance profit margins with customer loyalty.
The beauty of correlation lies in its ability to reveal significant relationships. Statistical significance ensures that observed correlations are not merely random occurrences but have a meaningful underlying connection. By testing hypotheses and examining statistical significance, we can distinguish between genuine relationships and those that may be influenced by chance factors.
Correlation is a powerful tool, but it’s essential to approach it with caution. While it can identify relationships, it cannot establish causality. Just because two variables are correlated doesn’t mean one causes the other. Additional analysis and experimentation are necessary to determine whether a causal relationship exists.
In conclusion, correlation is a fundamental statistical concept that unveils relationships between variables, aiding in prediction, understanding complex data, and testing hypotheses. However, it’s crucial to interpret correlations with caution, recognizing their limitations and the need for further investigation to establish causality.
Unveiling Relationships: The Fundamental Role of Correlation
Correlation, a statistical tool akin to a skilled detective, wields the remarkable ability to uncover hidden connections and unravel the intricacies of relationships between variables. Its keen eye scrutinizes data, seeking out patterns that reveal the strength and direction of these relationships.
Imagine two variables, like shoe size and running speed. Correlation steps into the scene, measuring the extent to which these two variables dance together. A positive correlation suggests that as shoe size increases, running speed tends to increase as well. Like two friends who love to explore, they move in the same direction.
On the other hand, a negative correlation paints a different picture. Consider age and memory. As age increases, memory may tend to fade, like a fading photograph. These variables move in opposite directions, indicating an inverse relationship.
Correlation’s superpower lies in its ability to quantify these relationships using a correlation coefficient, a number that ranges from -1 to 1. A coefficient close to 1 indicates a strong positive correlation, while a value near -1 suggests a strong negative correlation. Values closer to 0 indicate a weaker relationship.
So, next time you encounter data, remember correlation, the detective of relationships. It will guide you through the maze of variables, helping you understand how they interact and illuminating the hidden connections that shape our world.
Predictive Potential: Harnessing Correlation for Forecasting
Correlation, a cornerstone of statistical analysis, not only reveals relationships but also empowers us with the ability to predict future outcomes. Its predictive prowess shines brightly in the realm of forecasting, where businesses and researchers leverage correlation’s power to make informed decisions.
One such application is in regression analysis. This statistical technique uses correlation to establish a mathematical equation that predicts the value of one variable (dependent variable) based on another (independent variable). By identifying the strength and direction of their relationship, we can forecast future values of the dependent variable with remarkable accuracy.
For instance, in the retail industry, correlation can help predict customer demand based on factors like seasonality, marketing campaigns, and economic conditions. This knowledge enables businesses to optimize inventory levels, allocate resources effectively, and drive revenue growth.
Furthermore, correlation plays a pivotal role in forecasting techniques such as time series analysis. By examining the correlation between past data points, analysts can identify patterns and trends that can be extrapolated into the future. This predictive capability is essential for businesses to plan for seasonal fluctuations, manage supply chains, and forecast market trends.
Correlation and Causality: Exploring the Subtle Interplay
In the realm of statistical analysis, correlation reigns supreme as a tool to identify relationships between variables. It’s a dance of numbers, revealing the extent to which one variable sways in sync with another. While correlation can paint a vivid picture of these intertwined variables, it’s crucial to remember that it alone cannot establish causality.
Causality is the holy grail of statistical inference, the elusive link between cause and effect. Correlation provides a tantalizing glimpse into this hidden world, but it’s merely a catalyst, a gentle nudge that suggests a deeper connection may exist.
Imagine a study that uncovers a strong positive correlation between ice cream sales and drowning deaths. Does this mean that indulging in cold treats somehow leads to a watery end? Of course not! This apparent relationship is likely driven by a confounding variable, a hidden player in the mix: summer. As the sun beats down, people flock to the beach, seeking relief from the heat with ice cream and, unfortunately, sometimes falling victim to accidents.
Correlation, while illuminating, is a double-edged sword. It can hint at causality, but it can also be misleading. That’s why it’s essential to tread cautiously and dig deeper into the data, employing other statistical techniques to establish the true nature of the relationship.
Remember, correlation is a crucial step in the scientific exploration of causality, a beacon that guides us towards potential connections. But it’s only a step, not the final destination. With careful analysis and a healthy dose of skepticism, we can navigate the labyrinth of statistical data, uncovering the hidden truth that lies beneath the numbers.
Rigorous Assessment: Uncovering Meaningful Relationships
In our quest to understand the intricate relationships woven within data, determining the statistical significance of a correlation is paramount. It serves as a compass, guiding us towards discerning whether the observed relationship is a mere mirage or a robust reflection of an underlying connection.
Hypothesis testing emerges as our trusty ally in this endeavor. It challenges our hypothesis, scrutinizing it with an air of skepticism. A null hypothesis is postulated, asserting the absence of any correlation. The data is then subjected to statistical tests that calculate the p-value. This enigmatic value quantifies the likelihood that the observed correlation could have arisen by chance alone.
A low p-value (typically below 0.05) casts doubt on the null hypothesis, hinting that the correlation may not be a statistical fluke. It suggests that the observed relationship is unlikely to have occurred randomly, implying a meaningful connection between the variables.
Determining statistical significance is crucial as it helps us distinguish between true correlations and those that are simply the product of sampling error or chance occurrences. It ensures that our conclusions are grounded in reliable evidence, safeguarding us against erroneous interpretations.
By embracing hypothesis testing and determining statistical significance, we embark on a path of rigorous assessment, ensuring that the relationships we uncover are not mere mirages but genuine reflections of the underlying data structure.
Unveiling Hidden Influences: Controlling for Confounding Variables
In the realm of statistical analysis, correlation plays a pivotal role in unraveling relationships between variables. However, this journey can be marred by the presence of confounding variables, enigmatic factors that lurk in the shadows, obscuring true connections and swaying outcomes.
Confounding variables are like mischievous puppeteers, tugging on the strings of our data, distorting the relationships we observe. They are variables that are associated with both the independent and dependent variables in our analysis, acting as hidden influencers that can muddy the waters of correlation.
Imagine, for instance, that we’re investigating the correlation between coffee consumption and heart rate. We may observe a positive correlation, suggesting that people who drink more coffee have higher heart rates. However, what if we fail to account for the confounding variable of age? Older individuals tend to both drink less coffee and have higher heart rates. If we don’t control for age, our correlation will be inflated, falsely attributing the relationship between coffee and heart rate to the lurking influence of age.
To unveil these hidden influences, we employ statistical techniques that shine a light on confounding variables, revealing their true impact. One such technique is stratification, which involves dividing our data into subgroups based on the confounding variable. In our coffee-heart rate example, we could create subgroups for different age groups, analyzing the correlation within each group separately.
Another powerful weapon in our arsenal is regression analysis, a statistical method that allows us to control for the effects of confounding variables. Regression analysis effectively isolates the relationship between our independent and dependent variables, neutralizing the influence of confounders.
By controlling for confounding variables, we can ensure that our correlations reflect genuine relationships, not the illusions cast by hidden influencers. This rigorous approach allows us to draw more accurate conclusions from our data, uncovering the true nature of the connections that shape our world.
Unraveling Data Complexity: Correlation’s Role in Data Structure Analysis
In the world of data analysis, correlation plays a pivotal role in uncovering hidden patterns and relationships that lie within complex data sets. Statistical techniques like factor analysis, principal component analysis, and cluster analysis rely heavily on correlation to reveal the intrinsic structure and underlying dimensions within data.
Factor analysis, often used in market research and behavioral studies, leverages correlation to group variables that exhibit similar patterns into clusters known as factors. These factors represent the underlying latent dimensions that drive the observed variables, providing valuable insights into data complexity.
Principal component analysis, a widely utilized technique in data compression and dimensionality reduction, employs correlation to identify the most important directions of variability in a data set. By projecting data onto these principal components, analysts can gain a concise and meaningful representation of the data’s inherent structure.
Cluster analysis, on the other hand, utilizes correlation to partition data points into clusters based on their similarity. By grouping data points with similar characteristics, cluster analysis helps identify distinct subpopulations within a data set, allowing for targeted and effective data analysis.
In essence, correlation serves as the guiding force in these statistical techniques, enabling analysts to disentangle, understand, and interpret the complex relationships and patterns hidden within data. By unraveling data complexity, correlation empowers analysts to extract valuable insights that inform decision-making, facilitate prediction, and drive innovation.