Unlock Data Insights: Uncover Variance And Its Role In Decision-Making
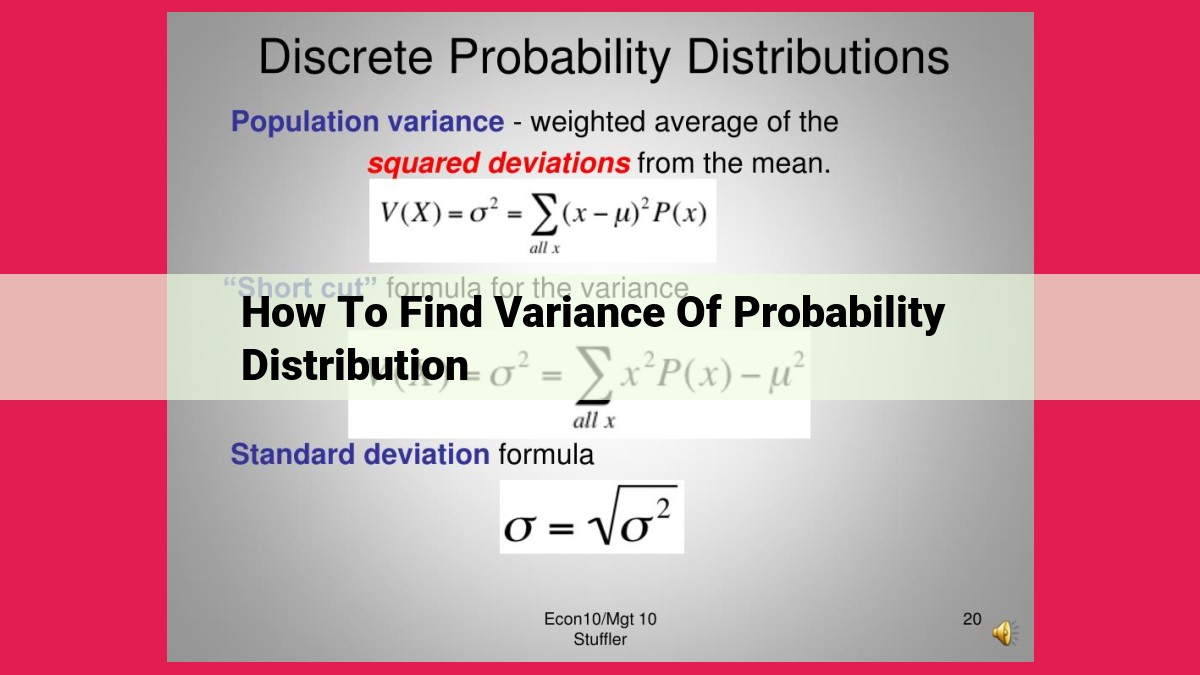
To find the variance of a probability distribution, first calculate the expected value (mean). Then, calculate the variance by finding the average of the squared deviations from the mean. This measures the spread or variability of the distribution. Variance provides insights into the consistency and reliability of outcomes, helping in decision-making, risk assessment, and statistical analysis.
Unlocking the Enigma of Variance: A Comprehensive Guide to Probability
Variance, a pivotal concept in probability theory, is a measure of how data is distributed around the central tendency or mean. Understanding variance is crucial for unlocking the complexities of probability and its myriad applications.
In the realm of statistics, the mean serves as the balance point of a distribution, a gravitational center around which the data revolves. Variance, on the other hand, is a measure of how far data points deviate from this central point. A low variance indicates that data is clustered closely around the mean, while a high variance signifies a wider spread of values.
Variance is a vital tool in probability theory, providing a quantifiable measure of the spread or dispersion of a distribution. It allows us to compare the variability of different distributions and make informed decisions about the underlying phenomena.
Understanding Variance: A Journey into the Expected and the Unexpected
In the realm of probability, variance holds a pivotal role, quantifying the deviation from the expected. Let’s embark on a conceptual journey to grasp this fundamental concept.
Expected Value: The Center of Gravity
The expected value (mean) represents the average outcome of a random event. Like the center of gravity, it balances out the distribution of possible outcomes. Consider a coin toss: the expected value is 0.5, reflecting the equal chance of landing heads or tails.
Variance: Measuring the Spread
Variance measures the dispersion of a distribution around the expected value. A high variance indicates a wide spread of outcomes, while a low variance suggests a more concentrated distribution. The formula for variance is the average of squared deviations from the expected value.
Variance and Expected Value in Tandem
Variance and expected value work hand in hand. The variance of a distribution cannot be lower than the square of the expected value. This fundamental relationship unveils that as the expected value increases, the distribution becomes less spread out.
Real-World Applications of Variance
Variance finds practical applications across disciplines:
- Finance: Assessing investment risk by measuring the volatility of stock prices.
- Manufacturing: Optimizing production processes by reducing variability in product quality.
- Psychology: Analyzing the spread of personality traits within a population.
Understanding variance empowers us to make informed decisions in the face of uncertainty. It’s the cornerstone of many statistical techniques, enabling us to quantify the predictable and the unpredictable in the world of probability.
Concept 2: Variance (Standard Deviation)
- Define variance and explain its mathematical formula.
- Interpret variance as a measure of variability in a distribution.
- Relate variance to expected value and provide real-life examples.
Concept 2: Variance (Standard Deviation)
Defining Variance: The Measure of Variability
Variance stands as a cornerstone concept in probability theory, quantifying the spread or variability within a distribution. Mathematically, it is defined as the average of the squared differences between each data point and the expected value (mean) of the distribution.
Understanding the Formula
The formula for variance is given as:
Variance = Σ((x - μ)^2 * P(x))
where:
- x represents each data point in the distribution
- μ is the expected value
- P(x) is the probability of occurrence for each data point
Interpreting Variance: A Measure of Consistency
Variance provides valuable insights into the behavior of a distribution. A smaller variance indicates that the data points tend to cluster closer to the mean, resulting in a more consistent distribution. Conversely, a larger variance suggests a wider distribution with data points more spread out from the mean.
Relating Variance to Expected Value
Variance is closely intertwined with expected value. A distribution with a higher expected value does not necessarily have a higher variance. For instance, consider two distributions: one with a high mean and low variance (tightly clustered data) and another with a high mean and high variance (widely scattered data).
Real-Life Applications of Variance
In practice, variance has numerous applications across various fields:
- Finance: Measuring the risk of investments or the volatility of stock prices.
- Quality Control: Assessing the consistency of manufacturing processes or the reliability of products.
- Population Genetics: Studying the genetic diversity within a population.
- Survey Research: Determining the representativeness of a sample by comparing the variance of sample responses to the population variance.
Concept 3: Covariance (Correlation Dependence)
- Define covariance and explain its mathematical formula.
- Interpret covariance as a measure of co-occurrence in a distribution.
- Discuss its relationship with correlation and applications.
Concept 3: Covariance – Unveiling the Secrets of Co-Occurrence in Probability
In the realm of probability, we encounter a fascinating concept known as covariance, a measure that quantifies the extent to which two random variables tend to vary together. It provides valuable insights into the underlying relationship between these variables, shedding light on their co-occurrence within a probability distribution.
Covariance is calculated using a mathematical formula that involves the expected value of each variable and the joint probability distribution of the variables. This calculation yields a numerical value that indicates the degree to which the variables fluctuate in tandem. A positive covariance suggests that the variables tend to move in the same direction, while a negative covariance indicates an opposite trend.
Interpreting covariance is crucial to understanding its significance. A high positive covariance implies that an increase in one variable is likely accompanied by an increase in the other. Conversely, a high negative covariance indicates that an increase in one variable is associated with a decrease in the other. A covariance close to zero suggests that there is little or no relationship between the variables.
Covariance finds widespread applications in various fields, including finance, economics, and data science. In finance, it helps assess the relationship between the returns of different assets, enabling investors to make informed diversification decisions. In economics, covariance plays a role in understanding the co-movement of economic variables, such as inflation and unemployment. Data scientists leverage covariance to identify patterns and relationships in large datasets, enhancing the accuracy of predictive models.
Understanding covariance is essential for gaining a deeper comprehension of probability theory. It provides a powerful tool for analyzing the co-occurrence of random variables, uncovering hidden relationships and making informed decisions based on probabilistic data.
Concept 4: Correlation (Relationship)
Picture this: you’re at a carnival, playing a ring toss game. As you toss rings towards a pyramid of cans, you notice a pattern. The closer you get to the top, the more likely you are to knock a can down. This pattern demonstrates a relationship between the distance from the pyramid and the success rate of the toss. In probability theory, we call this relationship correlation.
Correlation measures the strength and direction of the linear relationship between two random variables. It’s calculated using a mathematical formula that considers the covariances of the variables.
Interpreting Correlation
A correlation coefficient can range from -1 to 1. A correlation of 1 indicates a perfect positive relationship, meaning that as one variable increases, the other increases proportionally. A correlation of -1 indicates a perfect negative relationship, meaning that as one variable increases, the other decreases proportionally. A correlation of 0 indicates no linear relationship between the variables.
Correlation and Causality
It’s important to remember that correlation does not imply causation. Just because two variables are correlated does not mean that one causes the other. For example, the sales of ice cream and shark attacks may be positively correlated, but there’s no logical connection between them.
However, correlation can be a useful tool for inferring causality. If two variables are strongly correlated and there is a plausible mechanism that explains the relationship, it’s possible that one variable causes the other. To establish causality, further research and analysis are typically needed.
Concept 5: Joint Probability Distribution and Variance
In the realm of probability theory, the joint probability distribution holds great significance. It’s a map that charts the likelihood of two or more random events occurring together. This map paints a clear picture of the relationship between variables, revealing how they dance and interact within a distribution.
The bond between joint probability distribution and variance is unbreakable. Variance measures the spread or deviation of a distribution around its mean. By delving into the joint probability distribution, we can harness its power to calculate variance, providing valuable insights into the variability of variables.
Practical Applications of Variance in Joint Probability Distributions
This treasure trove of information has far-reaching applications in diverse fields:
- Finance: Assessing the risk associated with financial portfolios.
- Epidemiology: Understanding the spread of infectious diseases.
- Engineering: Predicting the reliability of complex systems.
Calculating Variance Using Joint Probability Distribution
Embark on the mathematical journey to calculate variance using joint probability distribution. Imagine a joint probability distribution table, a grid where each cell represents the likelihood of a particular combination of events. The formula for variance is a dance of probabilities, where each cell contributes its share:
Variance(X, Y) = ΣΣ (x - E(X))² (y - E(Y))² p(x, y)
Where:
- X and Y are random variables
- E(X) and E(Y) are their respective expected values
- p(x, y) is the joint probability of x and y
Variance is a cornerstone of probability theory, revealing the heartbeat of distributions. Its relationship with joint probability distribution empowers us to delve deeper into the interplay of variables, uncover patterns, and make informed decisions. From risk assessment in finance to unraveling the mysteries of disease spread, the applications of variance are endless. Embrace its power to unlock the secrets of probability and navigate the uncertainties of our world.