Understanding Outcome Variables, Independent Variables, And Control Factors For Effective Research
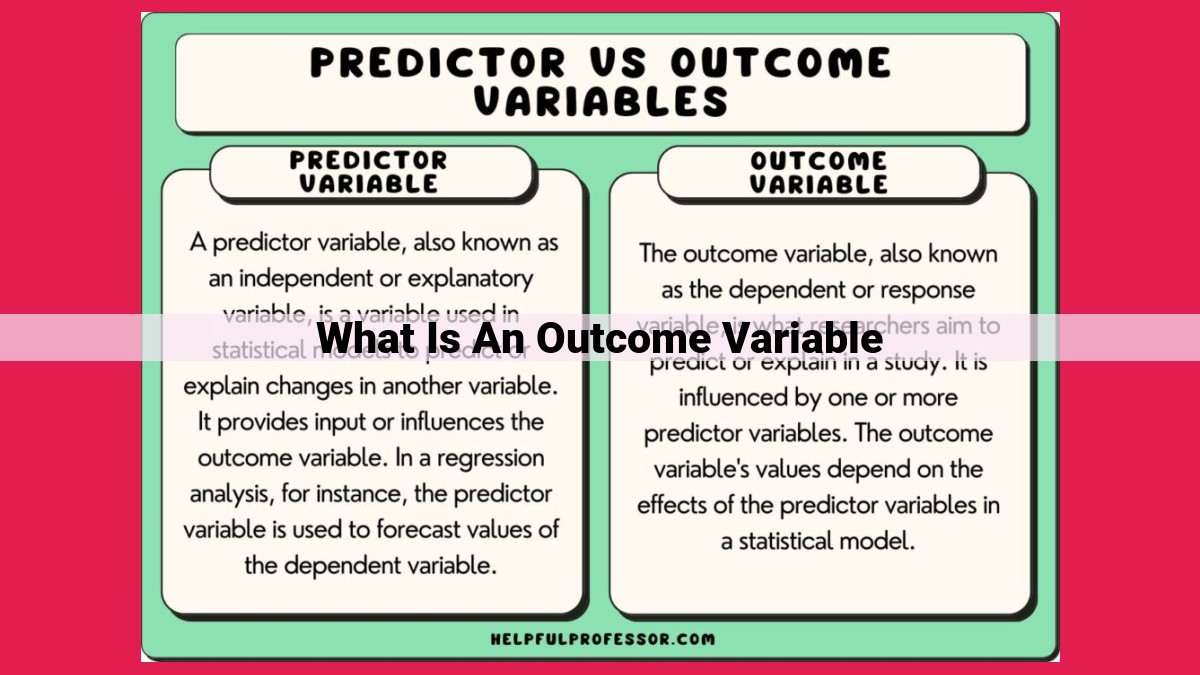
An outcome variable is a measurable characteristic that represents the effect of an independent variable on a research subject. It is used to assess the effectiveness of an intervention or treatment. Independent variables influence outcome variables by introducing a specific condition or manipulation. Control variables are used to eliminate the impact of confounding factors that may affect the outcome. Experimental and control groups allow for the comparison of outcome variables to determine the true effect of the independent variable. Understanding these concepts is crucial for effective research, as it enables researchers to isolate and measure the impact of specific variables and draw valid conclusions.
Outcome Variables: Understanding the Key Measure in Research
In the vast world of research, understanding cause-and-effect relationships is crucial. At the heart of this lies the outcome variable, a pivotal measure that reflects the impact of our actions or interventions.
An outcome variable is a quantifiable measure that captures the result of a study or experiment. It is the dependent variable, the one that changes in response to the manipulation of other variables. For instance, if you want to assess the effectiveness of a new study method, you might measure test scores as the outcome variable.
Understanding outcome variables is essential for drawing accurate conclusions from research. By measuring the changes in the outcome variable, we can determine the cause-and-effect relationship between the independent variables (the factors we manipulate) and the observed changes.
In our study method example, varying the amount of study time acts as the independent variable, while the resulting test scores serve as the outcome variable. By observing how changes in study time affect test scores, we can infer the relationship between these variables.
However, controlling for extraneous factors that could potentially influence the outcome is equally crucial. These control variables help us eliminate or minimize their impact. One way is to match participants on relevant characteristics like age or education level.
To strengthen the cause-and-effect inference, researchers often employ experimental and control groups. Participants are randomly assigned to these groups to ensure the equal distribution of potentially influential factors. By comparing the outcome variables between the two groups, researchers can isolate the effects of the independent variable.
For instance, in our study method example, we might assign participants to an experimental group that receives a study guide and a control group that does not. The difference in test scores between these groups would then provide a more precise estimate of the study guide’s effectiveness.
In conclusion, understanding outcome variables is paramount for effective research. They help us measure the impact of our manipulations, identify cause-and-effect relationships, and draw meaningful conclusions. By carefully controlling variables and employing experimental and control groups, we can enhance the reliability and validity of our research findings.
Independent Variables: Unveiling Their Influence on Outcomes
In the realm of research, understanding the intricate relationship between variables is paramount. Among them, independent variables play a pivotal role in shaping outcome variables. Grasping their essence and interplay is crucial for conducting rigorous and meaningful investigations.
An independent variable, as the name implies, is a variable manipulated by the researcher to observe its effect on the outcome variable. It represents a presumed cause or factor that may influence the result of the study. For instance, in an examination of the effectiveness of a new study method, the independent variable could be the amount of study time, while the outcome variable would be the test scores.
The manipulation of the independent variable allows researchers to isolate its influence and gauge its impact on the outcome. By systematically varying the independent variable, researchers can observe the corresponding changes in the outcome variable. In our study method example, different groups of participants could be assigned varying study times ranging from minimal to extensive, and their subsequent test scores would be compared.
The relationship between independent and outcome variables is not always straightforward. Some independent variables may have a linear effect, meaning that as the independent variable increases, the outcome variable increases proportionally. Others may have a curvilinear effect, where the outcome variable initially increases with the independent variable but eventually plateaus or declines. Additionally, the strength and direction of the relationship can vary depending on the context and other factors involved in the study.
Understanding the role of independent variables is essential for conducting research that can establish cause-and-effect relationships. By carefully controlling the independent variable and observing its impact on the outcome variable, researchers can draw meaningful conclusions about the effectiveness of interventions, the influence of environmental factors, or the relationships between different variables. This knowledge is invaluable for informing policy decisions, developing effective treatments, and advancing our understanding of the world around us.
Controlling Extraneous Factors: The Role of Control Variables
When conducting research, it’s crucial to control for extraneous factors that might influence your outcome variable, leading to biased or inaccurate results. This is where control variables come into play.
Control variables are characteristics or variables that affect the outcome variable but are unrelated to the independent variable being studied. They are included in the research design to minimize their confounding effect on the relationship between the independent and outcome variables.
There are several ways to control for confounding variables. One common method is matching. This involves selecting participants who are similar on the control variables. For example, if you’re studying the effect of a study method on test scores, you might match participants based on age and education level. This ensures that any differences in test scores are less likely due to these extraneous factors and more likely due to the study method itself.
Another way to control for confounding variables is blocking. This involves dividing participants into groups based on the control variables. By randomly assigning participants to groups, you can ensure that the groups are similar on the control variables and that any differences in the outcome variable are truly due to the independent variable.
Controlling extraneous factors through control variables is essential for ensuring the accuracy and validity of your research. By minimizing the impact of confounding variables, you can confidently determine the relationship between the independent and outcome variables and avoid misinterpreting your results.
Experimental and Control Groups: Unraveling Treatment Effects
When conducting research, we often aim to determine cause-and-effect relationships. One crucial way to achieve this is by employing experimental and control groups. These groups play a pivotal role in isolating the effects of specific treatments.
Random Assignment: The Foundation of Fairness
The key to unbiased results lies in random assignment. Participants are randomly allocated to either the experimental group, which receives the treatment, or the control group, which does not. This randomization ensures that both groups are comparable in terms of baseline characteristics, eliminating potential confounding factors.
Outcome Variable Comparison: Revealing Differences
Once participants have undergone the treatment or control condition, their outcome variables are compared. Outcome variables are the measurable results that indicate a change or effect due to the treatment. By comparing the outcome variables between the two groups, researchers can determine if the treatment had a significant impact.
Example: The Study Guide Efficacy
Imagine a study that aims to evaluate the effectiveness of a study guide. Participants are randomly assigned to either an experimental group that receives the study guide or a control group that does not. After a period of study, both groups take a test. If the experimental group performs significantly better on the test, it suggests that the study guide had a positive effect.
Experimental and control groups are indispensable tools for isolating and measuring treatment effects. Random assignment ensures fairness, while outcome variable comparison reveals differences between groups. By carefully controlling for extraneous factors, researchers can draw valid conclusions about the impact of specific treatments.
Understanding these concepts is paramount for effective research. It empowers researchers to discern cause-and-effect relationships, leading to evidence-based decisions that improve our understanding of the world and, ultimately, benefit society.