Understanding Variable Relationships: Control Confounders And Model Interactions
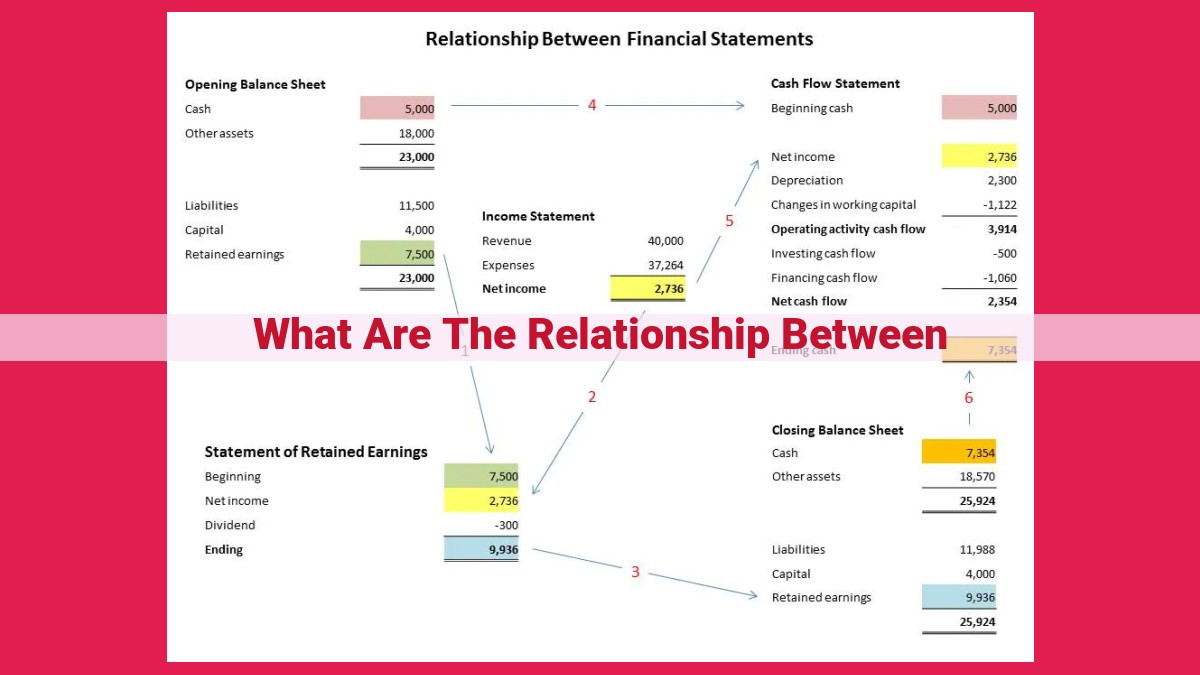
Understanding relationships between variables involves defining dependent and independent ones, exploring correlation, establishing causality, and recognizing spurious relationships. Lurking variables can create spurious relationships, highlighting the importance of controlling for confounders using experimental control or statistical methods. Statistical tools like regression analysis and path analysis aid in modeling and analyzing these relationships.
Defining Dependent and Independent Variables:
- Explanation of the concepts and their relationship in a research context.
Understanding the Interplay of Variables: Dependent and Independent Variables in Research
In the fascinating world of research, understanding the interplay between variables is crucial for unraveling the mysteries that surround us. Among the most fundamental concepts in research are dependent and independent variables.
Defining Dependent Variables
Imagine you’re conducting an experiment to explore the effects of fertilizer on plant growth. The variable you’re interested in measuring, the plant’s growth, is your dependent variable. It’s called “dependent” because its value depends on the manipulation of the independent variable.
Defining Independent Variables
The independent variable, on the other hand, is the one you manipulate or control to observe its impact on the dependent variable. In our plant growth experiment, fertilizer is the independent variable. It’s independent because its value is not influenced by the dependent variable.
The Dance of Dependency
The relationship between dependent and independent variables is a dance of cause and effect. The independent variable sets the stage, influencing the dependent variable. Just like in our plant growth experiment, the fertilizer we introduce influences the plant’s growth.
Remember, establishing a direct, causal relationship between variables is key in research. It’s not enough to simply observe a correlation between two variables; you need to demonstrate that one causes the other to change.
This intricate interplay of variables is fundamental to understanding the complexities of the world around us. By skillfully identifying and manipulating independent variables, researchers can unlock new insights and contribute to our ever-expanding knowledge base.
Exploring Correlation: The Dance Between Variables
In the realm of research and data analysis, correlation holds a crucial role in unraveling the hidden connections between different variables. It’s a measure that quantifies the strength and direction of the relationship between two or more variables.
Correlation can be positive, indicating that as one variable increases, the other variable also tends to increase. For instance, a positive correlation exists between ice cream sales and temperature. Conversely, a negative correlation suggests that as one variable increases, the other variable tends to decrease. An example is the negative correlation between exercise frequency and body fat percentage.
Types of Correlation
There are three main types of correlation:
- Perfect Positive Correlation (r = 1): Indicates a perfect linear relationship where one variable increases by a fixed amount for each unit increase in the other variable.
- Perfect Negative Correlation (r = -1): Indicates a perfect linear relationship where one variable decreases by a fixed amount for each unit increase in the other variable.
- Zero Correlation (r = 0): Indicates no relationship between the variables. The changes in one variable have no predictable effect on the other variable.
Statistical Measures of Correlation
The Pearson correlation coefficient (r) is the most common statistical measure used to calculate correlation. It ranges from -1 to 1, where:
- -1 indicates a perfect negative correlation
- 0 indicates no correlation
- 1 indicates a perfect positive correlation
Another measure is the Spearman’s rank correlation coefficient (rho), which is used to assess monotonic relationships that may not be linear.
Correlation is an indispensable tool for researchers and analysts. By exploring the relationships between variables, we gain insights into the intricate patterns that shape our world. However, it’s essential to interpret correlation with caution, as it does not imply causation. Correlation is a dance between variables, revealing their connection but not necessarily the underlying mechanism that drives their relationship.
Establishing Causation in Research: Unraveling the Cause-and-Effect Relationship
When seeking answers in research, we often strive to understand not only how variables are related, but also if one variable causes another. This pursuit of causation lies at the heart of scientific inquiry. However, establishing causation is a more intricate task than simply observing a correlation between two variables.
Criteria for Determining Causation
To establish causation, researchers rely on three key criteria:
- Temporality: The cause must precede the effect. In other words, the independent variable must occur before the dependent variable.
- Consistency: The cause-and-effect relationship must be repeatable across different studies and under various conditions.
- Elimination of other factors: The cause must be the only plausible explanation for the effect. Other potential factors that could influence the outcome must be ruled out.
Common Pitfalls in Establishing Causation
Although these criteria provide a framework for establishing causation, several common pitfalls can hinder researchers:
- Reverse causality: Sometimes, the effect appears to cause the cause. This can occur when two variables are mutually influential.
- Confounding variables: Uncontrolled variables that influence both the independent and dependent variables can create a spurious relationship, making it difficult to determine the true cause.
Avoiding Pitfalls and Ensuring Validity
To avoid these pitfalls and ensure the validity of causal claims, researchers employ various methods:
- Experimental control: Randomly assigning participants to different treatment groups allows researchers to rule out the influence of confounding variables.
- Matching: Matching participants on relevant characteristics can also help control for potential confounding factors.
- Longitudinal studies: Observing the development of a relationship over time can help establish temporality.
Establishing causation is a complex but crucial aspect of research. By adhering to the criteria of temporality, consistency, and elimination of other factors, and by avoiding common pitfalls, researchers can confidently draw causal inferences. Understanding the principles of causation allows us to unravel the intricate relationships between variables and gain deeper insights into the world around us.
Understanding Spurious Relationships: Unveiling the Illusion
In the realm of research, it’s essential to distinguish between genuine relationships and misleading coincidences known as spurious relationships. These deceptive connections arise when two variables appear to be linked, but in reality, the relationship is caused by a third, lurking variable that influences both variables.
Imagine the classic example of ice cream sales and drowning deaths. A researcher might observe a positive correlation between the two, concluding that eating ice cream leads to increased drowning risk. However, this apparent relationship is spurious. The lurking variable in this scenario is hot weather. As temperatures rise, people flock to the beach, resulting in both higher ice cream sales and a greater likelihood of drowning.
Spurious relationships can lead researchers astray, influencing conclusions and misdirecting policies. To avoid falling into this trap, researchers must be vigilant in identifying lurking variables and properly controlling for their influence.
Controlling for Confounding Variables: Unveiling the Hidden Ties
In the realm of research, understanding the relationships between variables is crucial. However, these relationships can be distorted by confounding variables—hidden factors that can influence both the dependent (outcome) and independent (predictor) variables, leading to erroneous conclusions.
The Sneaky Nature of Confounding Variables
Imagine studying the impact of exercise on weight loss. You might find a strong correlation between regular exercise and lower body weight. However, what if another factor, such as diet, is also influencing weight loss? In this case, diet would be a confounding variable. It affects both exercise (people who exercise tend to eat healthier) and weight loss, creating a spurious relationship between exercise and weight loss.
Unveiling the Hidden Influence
To avoid such pitfalls, researchers employ various methods to control for confounding variables. One powerful approach is randomization. By randomly assigning participants to different groups, such as an exercise group and a control group, researchers can reduce the influence of confounding variables. This ensures that the groups are similar in other relevant characteristics, such as diet, minimizing its impact on the results.
Another method is matching. Researchers match participants in the exercise group with similar participants in the control group based on potential confounding variables, such as age, gender, and initial weight. By doing this, they create groups that are comparable in key aspects, reducing the likelihood that confounding variables will skew the results.
The Importance of Control
Controlling for confounding variables is essential for uncovering true relationships between variables. By eliminating or minimizing their influence, researchers can increase the validity and reliability of their findings. This allows them to draw more accurate conclusions about the effects of independent variables on dependent variables.
Real-World Applications
In the field of medicine, controlling for confounding variables is particularly crucial. For example, researchers studying the effectiveness of a new drug might consider factors such as age, lifestyle, and other medications that could influence the drug’s effects. By controlling for these variables, they can gain a clearer understanding of the drug’s true efficacy.
By embracing these methods, researchers can navigate the complex web of relationships between variables, unveiling the hidden ties that may confound their results and ultimately leading to more nuanced and accurate insights.
Delving into Statistical Tools for Unveiling Relationships
Understanding the complex interplay between variables is crucial in research, and statistical tools play a pivotal role in uncovering these relationships. From regression analysis to path analysis and structural equation modeling, these techniques empower researchers to model and analyze the intricate connections between variables.
Regression Analysis: Unveiling Linear Relationships
Regression analysis is a ubiquitous statistical technique that allows researchers to investigate the relationship between a dependent variable and one or more independent variables. This powerful tool enables researchers to determine not only the direction and strength of the relationship but also to predict the value of the dependent variable based on the values of the independent variables.
Path Analysis: Deciphering Pathways
Path analysis expands upon regression analysis by allowing researchers to examine the causal relationships between multiple variables. By creating a graphical representation of the hypothesized relationships, path analysis helps identify direct and indirect effects, as well as the overall causal structure of the system under investigation.
Structural Equation Modeling: A Comprehensive Framework
Structural equation modeling is an advanced statistical technique that integrates regression analysis and path analysis into a comprehensive framework. This versatile tool allows researchers to simultaneously model complex relationships between multiple variables, incorporating both observed and latent (unobserved) variables. By testing hypotheses about the relationships between these variables, structural equation modeling provides valuable insights into the underlying dynamics of the system being studied.
Applying the Concepts: Unraveling Real-Life Relationships
Identifying Relationships in Our Daily Lives
In the tapestry of real-life events, understanding relationships between variables is crucial. Consider the correlation between study habits and exam scores. By observing students’ study time and their subsequent performance, we can identify a positive correlation. The more time students spend studying, the higher their scores tend to be.
Establishing Causation: Beyond Correlation
However, establishing causation requires more than just correlation. We need to demonstrate that studying (independent variable) directly causes higher exam scores (dependent variable). This involves meeting criteria like temporality, where studying precedes the exam, and eliminating other factors, such as prior knowledge or inherent intelligence.
Avoiding Pitfalls: Unveiling Spurious Connections
Beware, not all correlations imply causation! Spurious relationships can arise due to hidden variables influencing both the dependent and independent variables. For example, a correlation between ice cream sales and drowning incidents could be misleading. A lurking variable like hot weather may cause an increase in both ice cream consumption and swimming activities, creating the false impression of causation.
Controlling for Confounding Variables: Isolating the Truth
To accurately establish causation, it’s essential to control for confounding variables. Researchers use techniques like randomization (assigning participants randomly to different groups) and matching (equating groups based on relevant characteristics) to eliminate their influence. This helps isolate the effect of the independent variable on the dependent variable.
Statistical Tools: Unlocking the Complexity
Beyond simple correlations, statistical tools like regression analysis, path analysis, and structural equation modeling provide powerful means to model and analyze complex relationships between variables. These techniques can help researchers predict outcomes, identify intervening variables, and test causal hypotheses.
Practical Examples: Navigating the Real World
Let’s explore some practical applications. A marketing campaign might investigate the relationship between advertising spending and product sales. By controlling for factors like brand reputation and economic conditions, researchers can better determine if the advertising campaign caused the increase in sales.
In healthcare, a study might examine the correlation between smoking and lung cancer. However, by accounting for other factors like age, genetics, and occupational hazards, researchers can establish the causal link between smoking and this deadly disease.
Avoiding Common Pitfalls: A Cautionary Tale
Remember, research design and interpretation are fraught with potential pitfalls. Selection bias (when participants are not representative of the population) and confounding variables can lead to erroneous conclusions. By adhering to rigorous scientific methods and critical thinking, researchers can avoid these biases and uncover the true relationships between variables that shape our world.