Understanding Standard Error Of The Mean (Sx) For Accurate Statistical Analysis
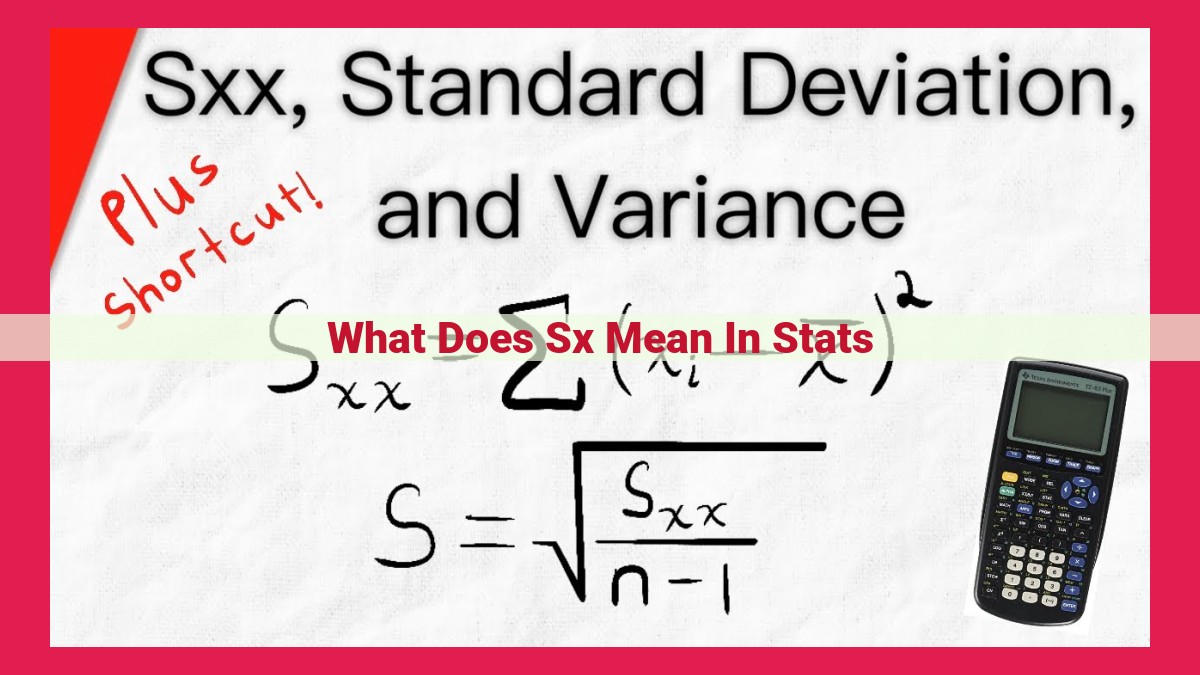
In statistics, SX (standard error of the mean) measures the variability of sample means from the true population mean. It represents the estimated accuracy of a sample and is crucial for determining confidence intervals and hypothesis testing. Factors like sample size, standard deviation, and variance influence SX, providing insights into the reliability of sample data. By understanding SX, researchers gain a deeper understanding of their results, reducing the risk of statistical errors and fostering accurate interpretations.
What Is Standard Error of the Mean (SX) and Why Does It Matter?
In the captivating world of statistics, the standard error of the mean (SX) reigns supreme as a beacon of accuracy. SX quantifies the reliability of sample means, guiding researchers toward confident estimations of population parameters.
Imagine yourself as an intrepid explorer, embarking on a mission to unveil the mysteries of a vast and hidden realm. As you venture forth, you encounter a perplexing obstacle: how can you know with certainty that your sample, a mere fraction of the entire population, truly reflects its characteristics?
Enter SX, the compass that illuminates your path. By factoring in the sample size and the inherent variability within the data, SX reveals the precision of your sample mean, painting a vivid picture of how closely your findings align with the true population.
In essence, SX represents the average distance between your sample mean and the true population mean. The smaller the SX, the more reliable your sample mean, and the greater your confidence in generalizing your findings to the entire population.
Your grasp of SX empowers you to:
- Determine the precision of your sample
- Establish confidence intervals, embracing uncertainty with clarity
- Test hypotheses and draw meaningful statistical inferences, unlocking the secrets of the hidden realm
Factors Affecting SX
- Sample Size: How sample size influences SX
- Standard Deviation: The relationship between standard deviation and SX
- Variance: The role of variance in calculating SX
Factors Affecting the Standard Error of the Mean (SX)
In the realm of statistics, understanding the standard error of the mean (SX) is crucial. It quantifies how much your sample mean might deviate from the actual population mean.
Sample Size
Imagine you’re conducting a survey on people’s favorite ice cream flavors. If you only ask a small group, say 20 people, your estimate of the most popular flavor might be heavily influenced by chance. However, if you surveyed a larger group of 200 people, your estimate would be more accurate and less prone to significant deviations from the true population. This is because a larger sample size reduces the SX.
Standard Deviation
The standard deviation measures the variability within your data. A higher standard deviation indicates greater variability, meaning your sample values are more spread out. Consequently, this increases the SX, making your estimate less precise. Conversely, a lower standard deviation means less variability, leading to a smaller SX and a more accurate estimate.
Variance
Variance is the square of the standard deviation and provides another perspective on data variability. It’s directly related to the SX, with a higher variance resulting in a larger SX and vice versa. By understanding the relationship between variance and SX, you can anticipate the precision of your sample estimates.
Applications of Standard Error of the Mean (SX)
In the realm of statistics, understanding SX is crucial, for it unveils the intricacies of sample accuracy. Just as a compass guides navigators, SX serves as a beacon for researchers, empowering them to draw meaningful conclusions from their data.
One significant application of SX lies in determining confidence intervals for population means. Imagine conducting a survey to estimate the average height of students in a particular school. The sample mean you obtain from the survey is an estimate of the true population mean, which is usually unknown. However, with SX, you can calculate confidence intervals that provide a range of plausible values for the population mean. This range gives you a sense of the accuracy of your estimate.
Another essential application involves hypothesis testing and making statistical inferences. Suppose you want to test whether a new teaching method improves student performance. By comparing the sample mean score of students using this method to a hypothesized population mean, you can calculate a test statistic using SX. This statistic helps you determine the probability of obtaining such a difference purely by chance. Based on this probability, you can make inferences about the effectiveness of the new method.
In essence, SX empowers researchers to quantify the uncertainty associated with their sample estimates and draw informed conclusions about the wider population. It is a fundamental tool in statistics, providing a solid foundation for robust and reliable research findings.