Understanding Confidence Intervals: Your Guide To Statistical Accuracy
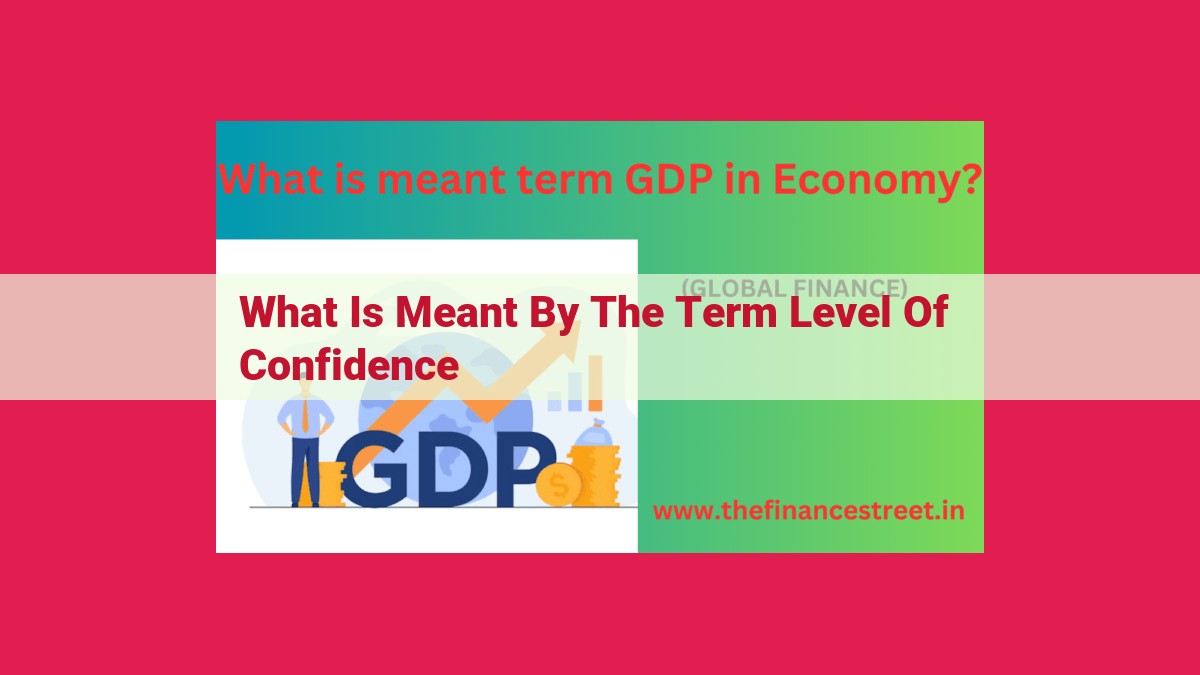
Level of confidence refers to the likelihood that a statistical inference is accurate, indicating the range within which the true value of a population parameter is expected to fall. It determines the width of the confidence interval, where a higher level of confidence results in a narrower interval, signifying greater certainty. Understanding the level of confidence is crucial for interpreting statistical results, as it influences the precision of estimates and the reliability of conclusions drawn from data.
Explanation of the concept of level of confidence and its significance in statistics.
What is Level of Confidence in Statistics?
Imagine you’re a detective trying to pinpoint the location of a hidden treasure. You start off with your best guess; a sprawling forest. As you gather more clues, you narrow down your search area. Level of confidence is like this narrowed-down area – it tells you how precise your guess is.
In statistics, level of confidence refers to the likelihood that your data accurately represents the actual population it’s drawn from. It’s expressed as a percentage, usually between 90% and 99%. A higher level of confidence means you’re more certain that your data is a true reflection of the population.
Why is it Vital?
Understanding level of confidence is essential for reliable data analysis. Without it, you can’t know how precise your estimates are and whether your conclusions are valid. Let’s say you find a box in the forest that you think contains the treasure. Without knowing the level of confidence, you don’t know if other boxes in the forest might also contain it.
Importance of Understanding the Level of Confidence for Reliable Data Analysis
Reliable data analysis is essential for drawing accurate conclusions from collected information. Understanding the level of confidence associated with your results is crucial to ensure the credibility of your findings.
Level of confidence determines the likelihood that your sample accurately represents the entire population. It measures the uncertainty associated with your estimates and provides a range within which you can expect the true population value to fall.
Insufficient understanding of confidence levels can lead to misinterpretations and incorrect conclusions. For example, if you report a difference between two groups without specifying the level of confidence, readers may assume it’s statistically significant without knowing the precision of your estimate.
Understanding confidence levels empowers you to make informed decisions about the interpretation of your results. It provides a framework for evaluating the reliability of your data and communicating your findings effectively.
By carefully considering the level of confidence, you can ensure that your data analysis provides trustworthy insights that can be confidently used to inform decisions and advance knowledge.
**Level of Confidence: Understanding Its Significance in Statistics**
Picture this: You’re conducting a survey to determine the average height of a population. The results you gather will provide an estimate of the true average, but it’s unlikely to be exact. That’s where level of confidence comes in.
Level of confidence is like a margin of error around your estimate. It tells you how confident you can be that the true value falls within a specific range. The higher the level of confidence, the narrower the range of possible values and the more precise your estimate.
But here’s the twist: Increasing the level of confidence also increases the range of possible values, known as the confidence interval. It’s a balancing act between precision and confidence.
So, how are level of confidence and confidence interval related? They’re like best friends! The level of confidence determines the size of the confidence interval. A higher level of confidence means a larger confidence interval, while a lower level of confidence results in a smaller confidence interval.
Remember: The level of confidence is a crucial factor in determining the accuracy and reliability of your statistical analysis. It’s like a roadmap guiding you to a clear understanding of your data.
Understanding the Margin of Error: Your Compass in the Sea of Confidence
In the realm of statistics, the level of confidence serves as our beacon, guiding us through the uncertain waters of data. It’s the measure of our belief that the results we obtain accurately represent the true population characteristics. Hand in hand with confidence, the margin of error plays a crucial role in navigating these waters, acting as the compass that defines the range of possible values within our confidence interval.
The margin of error is the amount by which our sample estimate may differ from the true population value. It’s a measure of the precision of our estimate, with a smaller margin of error indicating a more precise estimate. The margin of error is directly proportional to the level of confidence, meaning that a higher level of confidence will result in a wider margin of error and vice versa.
Imagine you’re tossing a coin to estimate the probability of getting heads. If you want to be 90% confident in your estimate, the margin of error would be larger than if you were only 50% confident. This is because with a higher confidence level, we want to be more certain that our interval includes the true population value, which in turn requires a wider range of possible values.
The margin of error not only helps us determine the range of possible values but also influences the width of the confidence interval. A wider confidence interval indicates a greater degree of uncertainty in our estimate, while a narrower interval suggests a more precise estimate. The width of the confidence interval is determined by the margin of error and the sample size.
In essence, the margin of error is our guide in the sea of confidence. It provides us with an understanding of the precision of our estimates and the range of possible values within which the true population value may lie. By considering both the level of confidence and the margin of error, we can make informed decisions about the reliability of our statistical inferences.
Impact of Level of Confidence on Margin of Error
Understanding the Correlation
The level of confidence and margin of error are two intertwined concepts in statistics. Level of confidence refers to the probability of a confidence interval containing the true population parameter. On the other hand, margin of error represents the range of values within which the true parameter is likely to fall.
Inverse Relationship
A crucial aspect to grasp is the inverse relationship between level of confidence and margin of error. As you increase the level of confidence, the margin of error widens. This is because a higher level of confidence demands a larger range of values to ensure that the true parameter is captured with the desired probability.
Why it Matters
The impact of this relationship is significant. If you require a high level of confidence in your results, be prepared for a wider margin of error. This means that the range of possible values for the true parameter will be broader. Conversely, if you can tolerate a lower level of confidence, you will obtain a narrower margin of error, resulting in a more precise estimate.
Practical Example
To illustrate, consider a poll that aims to estimate the proportion of voters supporting a particular candidate. Suppose the poll has a 95% level of confidence. This means that there’s a 95% chance that the true proportion of voters supporting the candidate falls within the margin of error.
If the poll results in a margin of error of 3%, it implies that the true proportion of voters supporting the candidate is likely between 47% and 53% (assuming the poll result is 50%). This range represents the potential values within which the true parameter might lie.
Understanding the inverse relationship between level of confidence and margin of error is paramount for reliable statistical analysis. This knowledge enables researchers and analysts to make informed decisions about the desired level of confidence and the corresponding margin of error based on the context and precision requirements of their research.
Understanding the Relationship Between Margin of Error and Confidence Interval Width
In statistics, a confidence interval provides a range of values within which we can be reasonably confident that the true population parameter lies. The margin of error determines the width of this interval, while the confidence level indicates how confident we are that the interval contains the true value.
The margin of error is directly proportional to the confidence level. As the confidence level increases, the margin of error also increases, resulting in a wider confidence interval. This relationship is intuitive: the higher the level of confidence we demand, the more conservative our estimate must be to ensure that it includes the true value with the specified probability.
For instance, consider a poll that estimates the percentage of voters who support a particular candidate. A margin of error of ±5% with a 95% confidence level意味着 that if we repeated the poll multiple times, 95% of those polls would produce results within 5% of the true percentage of voters who support the candidate.
In summary, the width of the confidence interval is determined by the margin of error, which is directly proportional to the confidence level. A higher confidence level leads to a wider confidence interval, while a lower confidence level results in a narrower interval. Understanding this relationship is crucial for interpreting and using confidence intervals effectively in statistical analysis and research.
Understanding the Role of Standard Deviation in Determining Confidence Intervals
The Tale of Data Variability
Imagine a group of friends who all love to play basketball. Each friend has their own unique shooting style, some more consistent than others. If we were to measure the distance of each friend’s shots from the basket, we would notice that some shots land closer to the hoop than others. This variability in shooting distances is what statisticians measure using standard deviation.
Standard Deviation: Measuring Scatter
Standard deviation is a statistical measure that quantifies how spread out a data set is. It measures the average distance of individual values from the mean (average) value of the data set. A small standard deviation indicates that the data points are clustered closely around the mean, while a large standard deviation suggests that the data is more spread out.
The Impact on Confidence Intervals
When determining the confidence interval for a population parameter (such as a mean or proportion), the standard deviation of the sample plays a crucial role. A larger standard deviation will result in a wider confidence interval, meaning that we are less certain about the true value of the parameter. Conversely, a smaller standard deviation will produce a narrower confidence interval, giving us greater confidence in our estimate.
The Balancing Act of Confidence
The level of confidence we desire in our estimates affects the width of the confidence interval. A higher level of confidence leads to a wider interval, while a lower level of confidence results in a narrower interval. It’s a balancing act between precision and confidence.
Practical Applications
Understanding standard deviation and its impact on confidence intervals is essential in research and data analysis. For example, if we are conducting a survey to estimate the average weight of adult men in a certain city, a higher standard deviation in the sample weights would indicate a wider range of possible values for the true average weight. Conversely, if the standard deviation were smaller, we could be more confident in our estimate.
Influence of standard deviation on the width of the confidence interval.
The Role of Standard Deviation in Determining Confidence Interval Width
In the realm of statistics, confidence intervals are like safety nets that protect us from uncertainty. They provide us with a range of values that have a high probability of containing the true population parameter we’re interested in.
But just as safety nets vary in their tightness, confidence intervals also have varying widths. And one of the key factors that influences their width is the standard deviation.
Imagine you’re trying to estimate the average height of students in your university. You randomly select a sample of 100 students and measure their heights. The standard deviation of their heights tells you how spread out their measurements are.
Now, let’s say you want to be 95% confident that the true average height falls within a certain range. The higher the standard deviation, the wider the confidence interval you’ll need. This is because a large standard deviation indicates significant variability in the data.
In other words, the standard deviation is like a measure of how predictable your data is. The less predictable, the wider the confidence interval. And the wider the confidence interval, the less precise your estimate of the true population parameter.
So, understanding the role of standard deviation is crucial for designing effective studies and interpreting results. It helps you determine the appropriate sample size and confidence level to achieve the desired level of precision in your data analysis. By considering the standard deviation, you can ensure that your confidence intervals provide a reliable range for your population estimates.
Definition of confidence level and its role in determining the range of values included in the confidence interval.
Level of Confidence: A Key Concept in Statistics
In the realm of statistics, we often encounter uncertainties and strive to make inferences about populations based on limited data. Level of confidence is a crucial concept that helps us navigate these uncertainties by establishing a range of plausible values for our estimates.
Confidence Interval and Level of Confidence
A confidence interval is a range of values within which we are confident that the true value of a population parameter lies. The level of confidence indicates the probability that the true value falls within this interval. For instance, a 95% confidence interval suggests that we are 95% sure that the true value is within the specified range.
Understanding the Confidence Level
The choice of confidence level depends on the balance between precision and the associated margin of error. A higher confidence level leads to a wider interval, while a lower confidence level results in a narrower interval. This is because as we increase the confidence level, we require a greater margin of error to account for the increased certainty.
Implications for Research and Data Analysis
Level of confidence plays a vital role in research and data analysis. By understanding the relationship between confidence level and margin of error, researchers can make informed decisions about the precision of their estimates. For example, in a study on customer satisfaction, a higher confidence level may be desirable to ensure greater certainty in the findings, even if it means a broader range of possible values.
Factors to Consider
When choosing the appropriate confidence level, researchers consider factors such as the sample size, the variability of the data, and the desired level of precision. The sample size has a direct impact on the width of the confidence interval. Larger sample sizes generally lead to narrower intervals and higher levels of confidence.
In conclusion, level of confidence is a fundamental concept in statistics that helps us quantify the uncertainty associated with our estimates. Understanding the relationship between confidence level, confidence interval, and margin of error is essential for making informed decisions and ensuring the reliability of our statistical analyses.
Understanding Level of Confidence: A Guide to Reliable Data Analysis
In the realm of statistics, the concept of level of confidence plays a crucial role in ensuring the reliability of data analysis. It represents the probability that confidence intervals, which estimate the range of possible values for a population parameter, contain the true value.
Level of Confidence and Associated Concepts
A confidence interval is a statistical tool that consists of a range of values within which the true population parameter is believed to lie with a specified probability. The level of confidence is the percentage of times that the true value is expected to fall within the confidence interval.
The margin of error is another key concept related to level of confidence. It represents the distance between the sample estimate and the population parameter, and it is inversely proportional to the level of confidence. A narrower margin of error indicates a higher level of confidence.
The Role of Standard Deviation
The standard deviation measures the variability or spread of the data. A higher standard deviation indicates greater variability, which in turn leads to a wider confidence interval.
Confidence Level and Level of Significance
The confidence level is closely related to the level of significance in hypothesis testing. The level of significance is the probability of rejecting the null hypothesis when it is actually true. A higher level of confidence corresponds to a lower level of significance.
Sample Size and Its Impact
The sample size has a significant impact on the width of the confidence interval. A larger sample size tends to produce narrower confidence intervals, resulting in a higher level of confidence.
Choosing the Appropriate Level of Confidence
The appropriate level of confidence depends on the specific research question and the desired level of precision. A higher level of confidence leads to wider confidence intervals and vice versa. Researchers must balance precision with confidence to make informed decisions.
Understanding level of confidence is essential for conducting reliable data analysis and drawing accurate conclusions. It allows researchers to determine the probability that their confidence intervals contain the true population parameter. By carefully considering level of confidence, sample size, and other relevant factors, researchers can ensure the validity and credibility of their research findings.
Importance of Sample Size in Determining Accuracy of Population Estimates
When we want to make inferences about a large population based on a smaller sample, the size of the sample plays a crucial role in determining the accuracy of our estimates. A larger sample size generally leads to more accurate results.
Imagine you want to know the average height of all adults in your city. Instead of measuring everyone, you decide to select a random sample of 100 individuals and measure their heights. The average height of your sample will give you an estimate of the true average height in the population.
Now, consider if you only selected a sample of 10 individuals instead of 100. The average height of this smaller sample is less likely to be close to the true population average because it’s less representative of the entire population.
The standard deviation of your sample, which measures the spread of data, also influences the accuracy of your estimate. A larger standard deviation indicates greater variability in the data, making it harder to draw precise conclusions from a smaller sample size.
In general, a larger sample size reduces the margin of error and increases the confidence interval. The confidence interval is a range of values within which we believe the true population parameter lies. A narrower confidence interval indicates higher confidence in our estimate.
For example, if we want to estimate the proportion of people who prefer a certain brand of coffee, a sample size of 1,000 will likely give us a more accurate estimate and a narrower confidence interval than a sample size of 200.
In conclusion, sample size is paramount in determining the accuracy of population estimates. A larger sample size generally leads to more precise estimates and narrower confidence intervals. When planning a study, selecting an appropriate sample size is essential to ensure reliable and insightful results.
Understanding Level of Confidence: A Guide for Statistical Analysis
The Role of Standard Deviation
Standard deviation is a statistical measure that describes how spread out a set of data is. It plays a crucial role in determining the width of the confidence interval. The wider the standard deviation, the more variable the data is, and the wider the confidence interval will be. This is because there is more uncertainty in the underlying population, leading to a greater range of possible values within the confidence interval.
Sample Size and Its Impact
The sample size is the number of observations in a given dataset. A larger sample size tends to produce a narrower confidence interval. This is because with more data points, we have a better representation of the population, leading to a more precise estimate. Conversely, a smaller sample size results in a wider confidence interval, as there is more uncertainty in the data.
The relationship between sample size and confidence interval width is inversely proportional. As the sample size increases, the width of the confidence interval decreases. This is because a larger sample size reduces sampling error, which is the difference between the population parameter and the sample estimate.
Choosing the Appropriate Level of Confidence
When selecting the desired level of confidence, several factors should be considered:
- Precision: A higher level of confidence results in a narrower confidence interval, providing a more precise estimate.
- Sample size: A larger sample size allows for a higher level of confidence without sacrificing precision.
- Available resources: Time and budget constraints may influence the achievable sample size and confidence level.
Balancing precision and confidence is essential. A higher level of confidence comes at a cost of decreased precision, potentially leading to overly narrow confidence intervals that may not accurately reflect the population. Conversely, a lower level of confidence may result in wider intervals, making it difficult to draw meaningful conclusions.
Impact of Sampling Error on Confidence Intervals
Imagine you’re a consumer researcher trying to estimate the average satisfaction rating of a new product. You conduct a survey of 500 randomly selected customers. Your survey yields an average satisfaction rating of 4.2.
However, because you only surveyed a sample of the population, your estimate may not be 100% accurate. This difference between the true population average and your sample estimate is known as sampling error.
The higher the level of confidence you set for your interval estimate, the wider the range around your sample average that will be included in the confidence interval. This means that higher confidence levels lead to more conservative estimates, as they account for a greater potential margin of error due to sampling.
Conversely, lower levels of confidence result in narrower confidence intervals. While these estimates are more precise, they may also be less reliable, as they are more susceptible to sampling error.
Therefore, it’s crucial to carefully consider the trade-off between precision and confidence. A higher level of confidence may yield a wider interval but provide greater assurance that the true population average falls within that range. Conversely, a lower level of confidence may result in a narrower interval but with a higher risk that the true average lies outside of it.
In the case of your survey, if you set a 95% confidence level, your confidence interval would likely be wider than if you had set a 90% confidence level. This is because a 95% confidence level accounts for a greater potential margin of error due to sampling.
Understanding Level of Confidence: A Guide to Reliable Data Analysis
When delving into the realm of statistics, one concept that often arises is the level of confidence. This enigmatic term holds significant sway over the interpretation of data and the conclusions we draw from it. As we embark on this blog post, we’ll unravel the complexities of level of confidence, exploring its significance, its relationship with associated concepts, and the factors that help us choose the most appropriate level of confidence for our analytical endeavors.
Level of Confidence and Its Significance
In the world of statistics, level of confidence refers to the likelihood that the results we obtain from a sample accurately represent the characteristics of the larger population from which that sample was drawn. Essentially, it’s a measure of how much we trust our data. A higher level of confidence indicates that we are more certain that our sample is representative of the population, while a lower level of confidence suggests a greater degree of uncertainty.
Associated Concepts: Confidence Interval and Margin of Error
The confidence interval is closely intertwined with the level of confidence. It represents the range of values within which we believe the true population parameter lies. The margin of error is the half-width of the confidence interval and provides a measure of the precision of our estimate. A smaller margin of error indicates a more precise estimate, while a larger margin of error suggests a more imprecise one.
Choosing the Appropriate Level of Confidence
Selecting the right level of confidence is crucial in statistical analysis. Several factors should be considered when making this decision, including:
- Desired level of precision: Consider the degree of accuracy you need in your estimate. A higher level of confidence results in a narrower confidence interval, increasing precision.
- Available sample size: The size of the sample you have collected can influence the level of confidence you can achieve. Smaller sample sizes generally yield lower levels of confidence.
- Cost and resources: Obtaining a higher level of confidence may require a larger sample size, which can increase the cost and time required for data collection.
- Consequence of error: Evaluate the potential consequences of making an incorrect decision based on your data. A higher level of confidence reduces the risk of such errors but may come at a cost.
Balancing Precision and Confidence
The choice between precision and confidence is often a delicate balancing act. A higher level of confidence leads to a narrower confidence interval and greater precision. However, this comes at the cost of increased sample size and resources. Conversely, a lower level of confidence allows for a larger margin of error but may be more economical and feasible in certain situations.
Understanding the level of confidence is paramount for reliable data analysis. By considering its significance, associated concepts, and the factors involved in selecting the appropriate level, we can ensure that our inferences and conclusions are well-founded. Remember, the level of confidence serves as a guidepost, helping us navigate the uncertainties inherent in statistical analysis and equipping us with the confidence to make informed decisions based on our data.
Understanding Level of Confidence: A Beginner’s Guide
Imagine you’re conducting a survey to gauge customer satisfaction. You collect 200 responses and find that 70% of respondents are satisfied. Is this a reliable estimate? How confident can you be in this result? That’s where level of confidence comes in.
Level of confidence is a crucial concept in statistics that helps us assess the reliability of our data. It represents the probability that the true value (in this case, the percentage of satisfied customers) falls within a confidence interval. The confidence interval is a range of values that reflects the possible range of outcomes.
The margin of error, which is a percentage or number, determines the width of the confidence interval. It’s crucial to understand that a higher level of confidence leads to a wider margin of error, and vice versa. This is because a wider margin of error encompasses a larger range of possible values, increasing our confidence that the true value is included.
However, this comes at a cost. A wider margin of error also means less precision. In our survey example, a 99% level of confidence with a 5% margin of error gives us a wider confidence interval than a 95% level of confidence with a 3% margin of error. While the higher confidence level provides more certainty, it also makes the range of possible values broader, reducing the precision of our estimate.
Balancing precision and confidence is essential. For example, in medical research, a high level of confidence (e.g., 99%) is often required to support significant claims. However, in marketing research, where speed and flexibility are often more critical, a lower level of confidence (e.g., 95%) may be acceptable, allowing for quicker decision-making.
Ultimately, the choice of level of confidence depends on the specific research question, the available data, and the desired level of certainty. By understanding the relationship between level of confidence and margin of error, we can make informed decisions about the reliability and precision of our statistical conclusions.
Recapitulation of the key concepts related to level of confidence.
Level of Confidence: Unraveling the Key Concepts
Imagine yourself as a detective on a mission to uncover the truth. To reach a solid conclusion, you need to be confident in your findings. Similarly, in statistics, the level of confidence plays a crucial role in determining the reliability of your data analysis.
The level of confidence is the probability that a true population parameter lies within a specific range, known as the confidence interval. Essentially, it tells you how likely your results are to be accurate. A higher level of confidence means a narrower confidence interval, resulting in a more precise estimate of the population.
Another key concept is the margin of error, which represents the half-width of the confidence interval. It’s like the radius around your target: the smaller the margin of error, the tighter the interval and the more confident you can be in your results.
The standard deviation measures the variability of your data. A higher standard deviation indicates more spread, which in turn widens the confidence interval. So, if your data is more variable, it becomes more challenging to make precise estimates.
The confidence level is closely related to the level of confidence. It represents the percentage of times that the true population parameter will fall within the confidence interval. A 95% confidence level means that you are 95% sure that the actual parameter lies within the interval.
Sample size also significantly impacts the accuracy of your estimates. A larger sample size leads to a narrower confidence interval, making your results more reliable. However, it’s important to strike a balance between precision and confidence. Choosing a very high level of confidence can result in an extremely narrow interval that may not be practical or necessary for your research.
Recapitulating the Key Concepts
In summary, the level of confidence is a fundamental statistic that helps us assess the reliability of our data analysis. It’s intertwined with concepts like confidence interval, margin of error, standard deviation, confidence level, and sample size. By understanding these concepts, you can make informed decisions about the level of confidence you need for your research and interpret your results with greater assurance.
Understanding Level of Confidence: A Key to Reliable Statistical Analysis
Imagine a researcher conducting a survey to estimate the average income of a particular population. After collecting the data, the researcher is faced with a crucial question: How much confidence can we have in the accuracy of our estimate?
Enter level of confidence, a fundamental concept in statistics. It reflects the researcher’s belief in the likelihood that the true population parameter (in this case, average income) lies within a specific range, known as the confidence interval.
The Significance of Level of Confidence
Consider two researchers conducting similar surveys with different levels of confidence. The researcher with a higher level of confidence is more certain that the true population parameter falls within a narrower range. This is analogous to placing a wider net (lower confidence) or a narrower net (higher confidence).
A higher level of confidence provides a more precise estimate, but it comes at the cost of a narrower confidence interval. Conversely, a lower level of confidence results in a broader confidence interval, indicating less certainty in the estimate.
Factors to Consider When Choosing Level of Confidence
The appropriate level of confidence depends on several factors:
- Precision Level: The desired level of accuracy in the estimate. Higher precision requires a higher level of confidence.
- Risk Tolerance: The willingness to accept some level of uncertainty in the estimate. A lower risk tolerance warrants a higher level of confidence.
- Resources: The time and resources available for data collection. Larger sample sizes generally allow for lower levels of confidence.
Balancing between precision and confidence is crucial. While a high level of confidence provides more certainty, it may not be practical or necessary in all scenarios. Researchers must carefully consider these factors to optimize their statistical analysis.
Level of Confidence: A Guide to Reliable Data Analysis
In the realm of statistics, level of confidence plays a pivotal role in ensuring the accuracy and reliability of data analysis. It represents the researcher’s belief in the likelihood that their results represent the true population values. Understanding this concept is essential for drawing meaningful conclusions from statistical data.
Confidence Interval and Margin of Error
Confidence interval is the range of values within which the true population value is likely to lie. The margin of error is half the width of the confidence interval and indicates the precision of the estimate. A higher level of confidence corresponds to a wider confidence interval and larger margin of error. Conversely, a lower level of confidence results in a narrower confidence interval and smaller margin of error.
Standard Deviation and Confidence Interval
Standard deviation measures data variability. A higher standard deviation leads to a wider confidence interval because there is more uncertainty regarding the true population value.
Confidence Level and Level of Significance
Confidence level is the probability that the true population value falls within the confidence interval. It is closely related to the level of significance used in hypothesis testing. A higher confidence level corresponds to a lower level of significance and vice versa.
Sample Size and Confidence Interval
Sample size significantly impacts the accuracy of confidence intervals. A larger sample size generally leads to a narrower confidence interval, while a smaller sample size results in a wider confidence interval.
Applications of Level of Confidence
Level of confidence has wide-ranging applications in research and data analysis. For instance, in medical research, it is used to determine the efficacy and safety of new treatments. In consumer research, it is utilized to estimate market share and customer satisfaction. In social science research, it is employed to understand population attitudes and behaviors.
Level of confidence is a fundamental concept in statistics that guides researchers in making informed decisions about data analysis. By considering the interplay between confidence level, margin of error, and sample size, researchers can construct accurate and reliable confidence intervals. This knowledge empowers them to interpret results confidently and draw meaningful conclusions from their data.