Unveiling The Pitfalls Of Statistical Manipulation: A Guide To Critical Data Interpretation
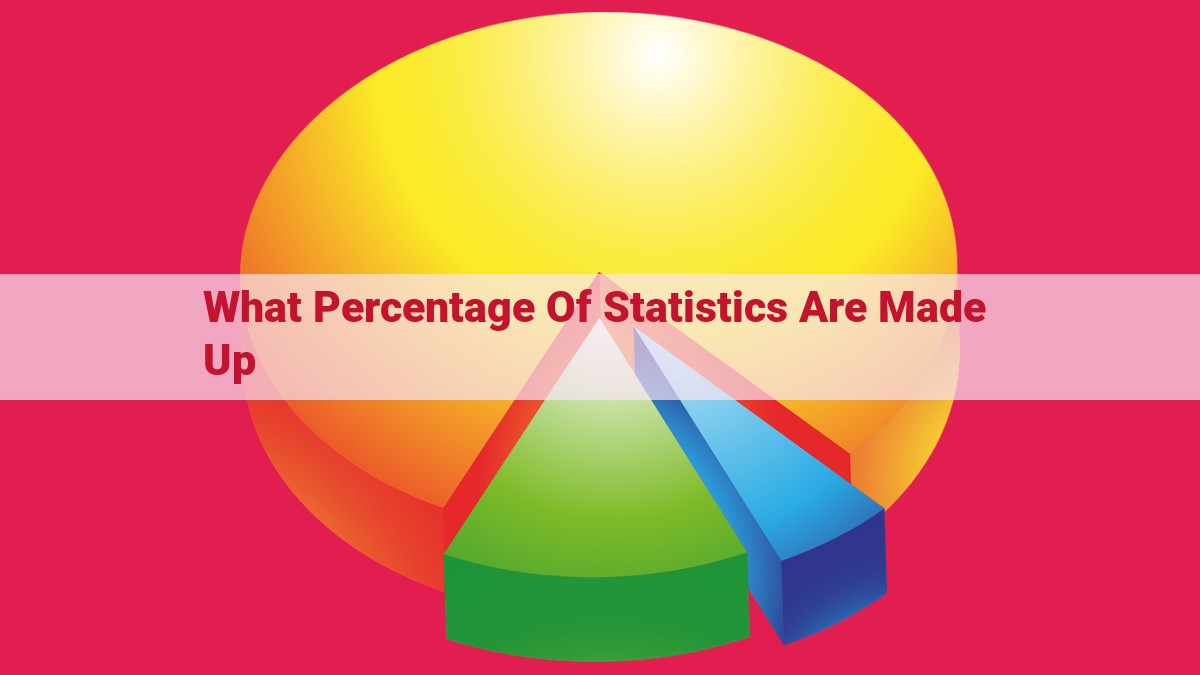
Statistics are crucial in today’s world, but their accuracy can be compromised by manipulation. Biased sampling, cherry-picking, and data dredging can lead to misleading results. Understanding the nature of statistical significance, context, and effect size is crucial for accurate interpretations. Data manipulation and biased sampling can influence interpretations, highlighting the need for critical evaluation and consideration of multiple perspectives. Consequently, it is essential to approach statistical information with caution, recognizing its limitations and nuances.
The Nature of Statistics: Unraveling the Truth Amidst Numbers
In the realm of numbers, where data reigns supreme, Mark Twain once quipped, “There are three kinds of lies: lies, damned lies, and statistics.” This witty observation encapsulates the complex and often deceptive nature of statistics, a field that plays an increasingly significant role in shaping our understanding of the world around us.
Today, statistics are ubiquitous, permeating every aspect of our lives. From the headlines we read to the decisions we make, statistics have the power to inform, persuade, and even manipulate our perceptions. It is essential to approach this numerical landscape with both skepticism and understanding, as the proper interpretation of statistics can mean the difference between informed decision-making and falling prey to deception.
Data Manipulation: The Art of Deception
In the realm of statistics, as the legendary Mark Twain once quipped, “There are three kinds of lies: lies, damn lies, and statistics.” This enigmatic quote succinctly captures the potential for data manipulation to distort the truth and mislead audiences.
Defining Data Manipulation
Data manipulation refers to any alteration or fabrication of data with the intent to influence the outcome of a statistical analysis. It can take various forms, each with its own nefarious purpose.
Examples of Data Manipulation Techniques
- Biased Sampling: This occurs when researchers select a sample that is not representative of the population they intend to study. By excluding or overrepresenting certain groups, they can skew the results in their favor.
- Cherry-Picking: When researchers selectively present only the data that supports their desired conclusion while omitting or downplaying data that contradicts it, they are engaging in cherry-picking. This practice creates a distorted and incomplete picture.
- Data Dredging: This involves repeatedly analyzing a dataset in different ways until they find results that support their hypothesis. By sheer chance, they may eventually stumble upon statistically significant findings, even if there is no real relationship between the variables.
Impact on Statistical Results
Data manipulation can have a profound impact on statistical results. By altering the underlying data, researchers can create the illusion of significance where none exists or hide the true relationships between variables. This undermines the integrity of the research and leads to misleading conclusions.
Data manipulation is a serious threat to the credibility of statistical analysis. It is essential for researchers and consumers of statistical information to be aware of these deceptive techniques and to approach statistical claims with a critical eye. By understanding the potential for data manipulation, we can safeguard ourselves against being misled and ensure that our decisions are based on accurate and unbiased information.
Interpretation: Making Sense of the Numbers
When it comes to statistics, the numbers themselves are just the beginning. The true value lies in the interpretation and understanding of those numbers.
Bias and Limitations: Color the Picture
Like a prism that bends light, bias and limitations can shape the way we interpret statistical results. Bias, whether conscious or unconscious, can creep into data collection and interpretation, skewing our conclusions. Similarly, limitations in the study design or sample size can impact the generalizability of the findings.
The Dark Art of Data Manipulation
Just as a magician uses misdirection to create illusions, data manipulation can make statistical results seem more impressive or convincing than they are. Cherry-picking favorable data points, discarding inconvenient ones, or using misleading statistical techniques can all lead to distorted interpretations.
Unveiling the True Meaning
To navigate the statistical landscape effectively, we must be critical thinkers and scrutinize the numbers with a keen eye. Consider the following:
- Context is Key: Statistical results don’t exist in a vacuum. They must be interpreted in the context of the study’s objectives, target population, and limitations.
- Effect Size Matters: Statistical significance is important, but it’s not the whole story. Effect size measures the magnitude of an effect, providing a sense of its practical importance.
- Multiple Perspectives: No single statistical result is absolute truth. Seek out multiple perspectives and alternative explanations to gain a more comprehensive understanding.
Statistics can be a powerful tool for understanding the world around us, but they must be used and interpreted with discernment. By recognizing the potential for bias, limitations, and data manipulation, and by carefully considering the context and implications of statistical results, we can navigate the statistical landscape with confidence and uncover the true meaning behind the numbers.
Nuance: Embracing the Complexity of Statistics
Statistics often provides us with clear-cut answers, but even the most rigorous statistical methods are subject to nuances that can influence the interpretation of results. Acknowledging these nuances is crucial for navigating the statistical landscape with confidence and avoiding common pitfalls.
One significant factor to consider is bias, which can creep into statistical analysis at various stages. Bias in data collection, for example, can skew the results if the sample is not representative of the population being studied. Similarly, bias in data interpretation can arise from preconceived notions or selective reporting of findings.
To unveil the true nature of statistical results, it is essential to consider multiple perspectives and explore alternative explanations. Every set of data can be interpreted in different ways, and without embracing these nuances, we risk oversimplifying complex relationships. For instance, a study might show a correlation between ice cream sales and drowning rates, but it would be naïve to assume a causal relationship without considering other factors such as weather and proximity to water.
Ultimately, true understanding comes from recognizing that statistics is not merely a series of numbers, but a tapestry of different perspectives and potential interpretations. Embracing the complexity of statistical analysis allows us to make informed decisions, avoid misinterpretations, and navigate the statistical landscape with a healthy dose of skepticism and critical thinking.