Understand Predictor Variables: Unveiling The Factors Driving Outcomes
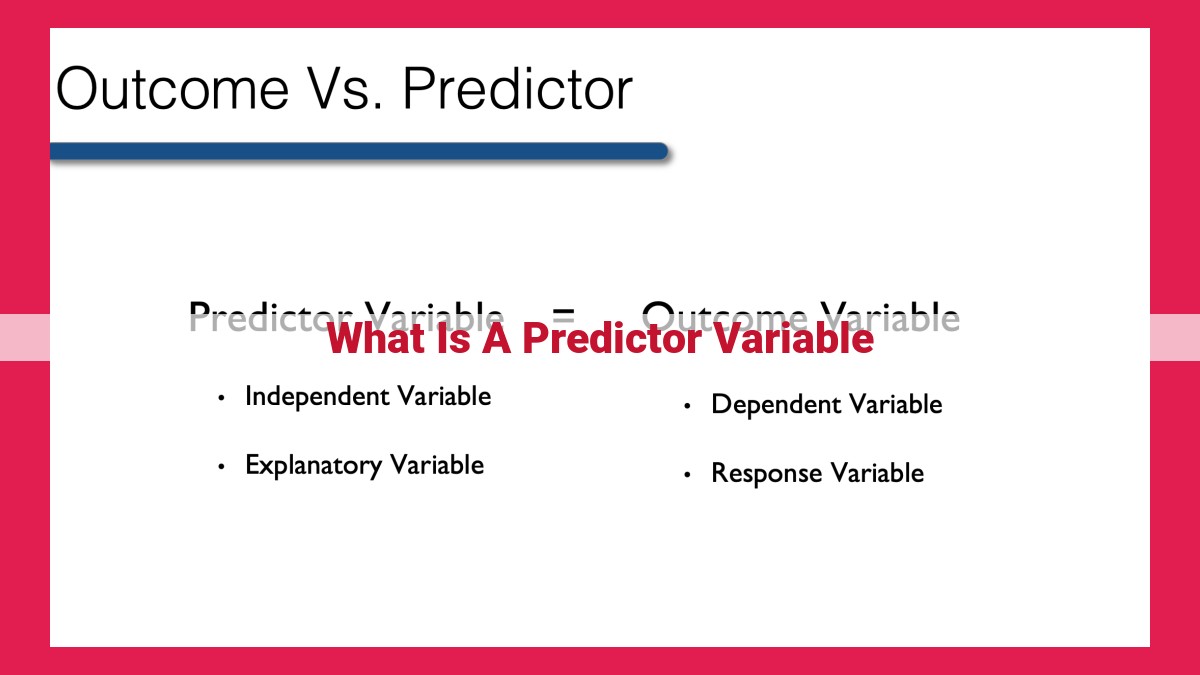
A predictor variable, also known as an independent or explanatory variable, is a factor that influences or explains the variation in a specific outcome or dependent variable. In statistical analysis, predictor variables are used to understand the relationships between different variables and to make predictions or inferences. They help identify the factors that contribute to an outcome and can be used to develop models or forecasts based on their influence on the dependent variable.
- Definition of a predictor variable and its role in statistical analysis
- Importance of predictor variables in understanding relationships
Predictor Variables: Unveiling the Hidden Determinants
In the realm of statistics, unraveling the complexities of relationships is crucial, and at the heart of this endeavor lie predictor variables. These essential elements hold the key to deciphering the intricate web of cause and effect, shedding light on the underlying factors that shape our world.
A predictor variable, also known as an explanatory variable, is a characteristic or measurement used to predict the value of another variable, termed the dependent variable. It’s like a compass guiding us through the labyrinth of data, pointing us towards the factors that influence the outcomes we observe.
Understanding the significance of predictor variables is paramount. They provide the answers to essential questions: Why does one event occur more frequently than another? What combination of factors contributes to a particular outcome? By identifying these variables, we gain invaluable insights into the dynamics that govern our lives and environments.
Key Concepts Related to Predictor Variables
Independent Variable: The Driving Force
Think of an independent variable as the trigger that sets the chain reaction in motion. It’s the cause that influences the outcome. In a classic experiment, we might investigate how different watering schedules affect plant growth. The amount of water (independent variable) directly influences the plant’s height (dependent variable).
Explanatory Variable: Unraveling the Reasons
Predictor variables serve as explanations for the observed outcome, shedding light on the underlying mechanisms. In the plant growth experiment, factors like sunlight and soil quality can act as explanatory variables. By examining their effects on plant growth, we gain insights into the processes involved.
Dependent Variable: The Resulting Effect
The dependent variable is the consequence, the outcome that we’re ultimately interested in. In our plant growth example, it’s the height of the plant. It’s important to note that the dependent variable is influenced by the independent variable, but not the other way around.
Additional Considerations for Predictor Variables
When delving into the world of statistical analysis, it’s imperative to consider covariates and confounding variables to ensure the accuracy and validity of your findings.
Covariates: The Unsung Heroes of Relationships
Covariates are additional predictor variables that influence the relationship between the primary predictor variables and the dependent variable. Think of them as the sidekicks that add context to the main story. They can strengthen or weaken the observed relationships, providing a more nuanced understanding of the underlying dynamics.
For instance, consider a study exploring the relationship between education level and income. Age is a relevant covariate in this scenario since it can affect income independently of education level. By including age as a covariate, the researchers can isolate the true impact of education on income, accounting for the potential confounding influence of age.
Confounding Variables: The Tricksters of Relationships
Confounding variables are lurking variables that can distort the observed relationship between predictor variables and the dependent variable. They act like sneaky imposters, pretending to be influential when they’re not.
Imagine a study investigating the effectiveness of a new drug for treating a particular disease. Unbeknownst to the researchers, the participants in the study also received a different medication that might have influenced the observed outcomes. In this case, the additional medication is a confounding variable, potentially masking or exaggerating the true effect of the new drug.
To tackle confounding variables, researchers employ various strategies, such as randomized controlled trials, where participants are randomly assigned to different treatment groups, minimizing the potential influence of confounding variables.
By considering covariates and confounding variables, researchers can unravel the complexities of relationships, ensuring that their findings are reliable and insightful.
Unlocking the Power of Predictor Variables: Unveiling Causal Relationships, Future Predictions, and Informed Decision-Making
In the realm of statistical analysis, predictor variables hold the key to unraveling the intricate relationships between different variables. These variables, often known as independent or explanatory variables, provide valuable insights into the factors that shape outcomes.
Understanding Causal Relationships
Predictor variables enable us to establish causal relationships between variables. By carefully controlling and manipulating predictor variables, researchers can determine their direct impact on the dependent variable (the outcome being measured). This knowledge empowers us to discern cause-and-effect relationships, providing a deeper understanding of the underlying mechanisms influencing phenomena.
Predicting Future Outcomes and Making Inferences
The predictive power of predictor variables is another invaluable asset. By analyzing historical data and identifying the relationships between predictor variables and outcomes, we can develop models to predict future outcomes. These models serve as powerful tools for making educated guesses about events that have yet to unfold, allowing us to make informed decisions based on data-driven insights.
Developing Models for Forecasting and Decision-Making
The significance of predictor variables extends to the development of models for forecasting and decision-making. In fields such as finance, marketing, and healthcare, accurate forecasting is crucial for strategic planning and risk mitigation. By incorporating predictor variables into predictive models, organizations can gain a competitive advantage by better anticipating market trends, optimizing customer engagement, and improving patient outcomes.
In conclusion, predictor variables play a pivotal role in statistical analysis by facilitating the understanding of causal relationships, predicting future outcomes, and developing models for forecasting and decision-making. Their significance lies in empowering us to make informed decisions based on data, unlocking new possibilities for innovation, and driving progress across various fields.