A Comprehensive Guide To Population Of Interest: Understanding Its Importance For Research Validity
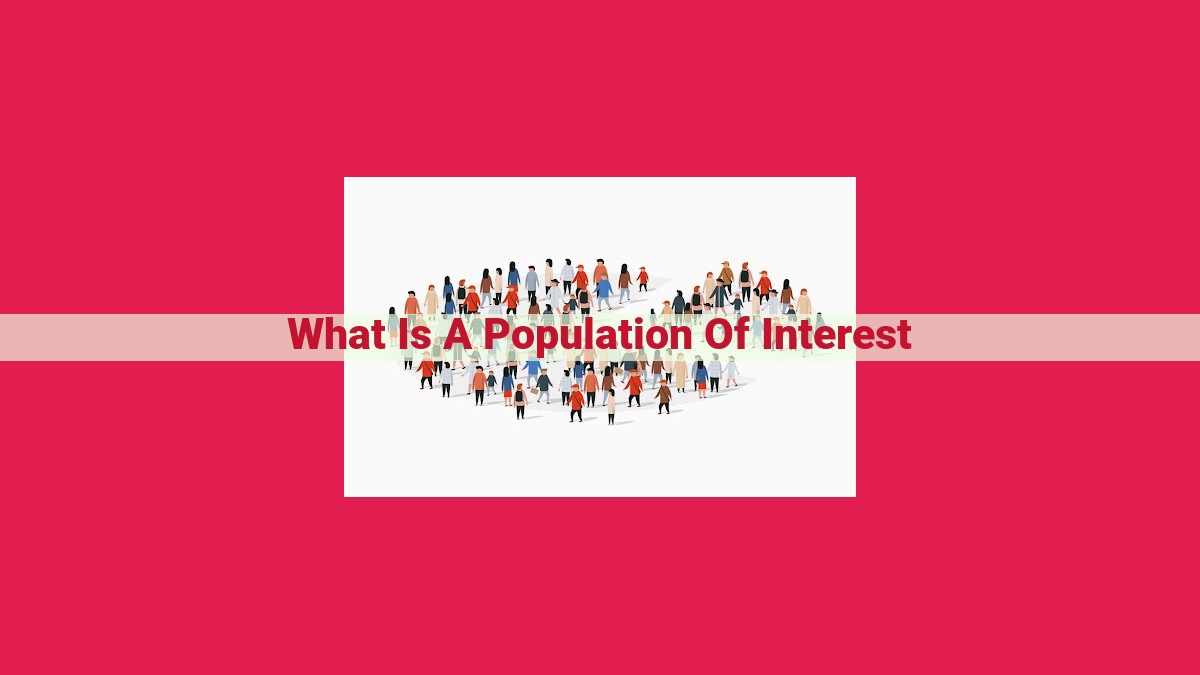
A population of interest refers to the entire group from which researchers seek to draw conclusions. It is the target population that the research aims to represent. By obtaining a representative sample that adequately captures the characteristics of the target population, researchers can make inferences about the larger group and generalize their findings. Understanding the concept of population of interest is crucial for the validity and generalizability of research.
In the vast landscape of research, understanding the population of interest is paramount to unlocking meaningful insights. This concept encompasses the entire group or phenomenon under investigation, representing a universe of potential subjects or observations. Its significance lies in guiding researchers to focus their inquiry on a specific subset of the population that is both manageable and relevant to their research question.
Defining the population of interest requires a clear understanding of its target population. This is the specific group or subset of the population that researchers intend to generalize their findings to. By establishing a target population, researchers can ensure that their findings are applicable to a broader group of individuals or phenomena.
Understanding the population of interest and its target population forms the foundation of any research inquiry. This knowledge enables researchers to design studies that accurately represent the target population and draw valid conclusions. It also helps researchers identify potential biases or limitations that may arise due to sampling or other factors.
The Target Population: The Cornerstone of Research
In the realm of research, the concept of population holds paramount importance. It encompasses the entire group of individuals, objects, or events under investigation. However, researchers often focus on a specific subset of the population, known as the target population. This group serves as the foundation upon which research is conducted and conclusions are drawn.
The target population is closely intertwined with the broader population of interest. The population of interest represents the entire group that the researcher ultimately wants to understand or generalize about. For instance, if a researcher is interested in studying the health habits of adults in a particular city, the population of interest would be all adults residing in that city.
The distinction between the target population and the population of interest lies in accessibility. While the researcher may have an interest in understanding the entire population, it may not be feasible or practical to study every single individual. Therefore, they select a target population that is representative of the population of interest and allows for efficient data collection.
For example, in the aforementioned study on health habits, the researcher may not have the resources to survey every single adult in the city. Instead, they may choose a target population of 1,000 randomly selected adults, ensuring that the sample is diverse enough to reflect the characteristics and demographics of the population of interest.
By carefully defining the target population and ensuring its representativeness, researchers can increase the generalizability of their findings. Generalizability refers to the extent to which the results of the study can be applied to the population of interest. The more representative the target population is, the more confident the researcher can be that the findings are applicable to the wider group.
Representativeness: Bridging the Gap
In research, representativeness is crucial as it ensures that the sample accurately reflects the larger population of interest. A representative sample is a microcosm of the population, capturing its diversity and characteristics.
Factors Influencing Representativeness
Multiple factors contribute to representativeness, including:
- Sampling Method: Simple random sampling and stratified sampling promote representativeness by ensuring every individual has an equal chance of being selected and by preserving the population’s subgroups.
- Sample Size: A larger sample size generally yields a more representative sample, as it increases the likelihood of capturing the population’s variability.
Importance of Representative Sampling
Obtaining a representative sample is essential for accurate inferences. It helps researchers:
- Generalize Findings: A representative sample allows researchers to generalize their findings to the larger target population. This is critical for making informed decisions and creating policies that impact wider society.
- Avoid Bias: A representative sample controls for potential biases that may arise from sampling particular groups or individuals. This enhances the validity and credibility of research findings.
- Increase Confidence: A representative sample increases confidence in the research results, as researchers can be more certain that their findings reflect the population of interest.
Generalizability: Extending Findings
When we obtain a representative sample from the population of interest, we can make generalizations about the larger population based on the findings from our sample. This process of generalizing from a sample to a population is known as generalizability.
The extent to which findings can be generalized depends on the sampling error involved. Sampling error refers to the difference between the characteristics of the sample and the actual characteristics of the population. The smaller the sampling error, the more accurate the generalizations will be.
However, it is important to recognize that generalizability implied by research findings has certain limitations. Firstly, generalization can only be made to the specific population that was sampled. Secondly, generalizations may be limited by biases introduced during the sampling process.
For example:, if we conduct a survey to understand the voting preferences of registered voters, our findings can only be generalized to the population of registered voters. It cannot be generalized to the entire population of adults, as non-registered voters are not represented in our sample.
Sampling Error: The Variability Factor
Understanding Sampling Error
When conducting research, we often rely on samples to represent a larger population. However, samples are inherently imperfect, and the difference between the sample and the population is known as sampling error. This error can affect the accuracy and reliability of our research findings.
Types of Sampling Error
There are two main types of sampling error:
- Random sampling error: This occurs simply because the sample is not identical to the population. It is always present to some extent and is the main source of sampling variability.
- Non-random sampling error: This occurs when the sample is not representative of the population due to factors such as biased sampling methods or small sample sizes.
Effects of Sampling Error
Sampling error can impact research outcomes in several ways:
- Underestimation or overestimation of population parameters: A sample may not accurately reflect the true population mean, variance, or other characteristics. This can lead to erroneous conclusions.
- Reduced confidence in results: High levels of sampling error increase the uncertainty of our findings, making it difficult to generalize to the population.
- Bias: Non-random sampling errors can introduce bias into the sample, potentially leading to inaccurate or distorted results.
Minimizing Sampling Error
Fortunately, there are strategies to minimize sampling error and enhance the generalizability of our findings:
- Increase sample size: The larger the sample, the more likely it is to be representative of the population.
- Use random sampling methods: Random sampling ensures that all members of the population have an equal chance of being selected.
- Stratify the sample: Dividing the population into homogeneous subgroups and sampling from each subgroup can reduce bias.
- Weighting: Adjusting the data to account for potential biases can help improve representativeness.
- Consider the population size: For small populations, sampling error is likely to be more pronounced.
By understanding sampling error and implementing appropriate strategies to minimize it, researchers can enhance the validity and reliability of their findings. This helps ensure that research conclusions are accurate and meaningful in the context of the broader population.