Understand The Impact Of Outliers On Statistics: Maximizing Data Reliability
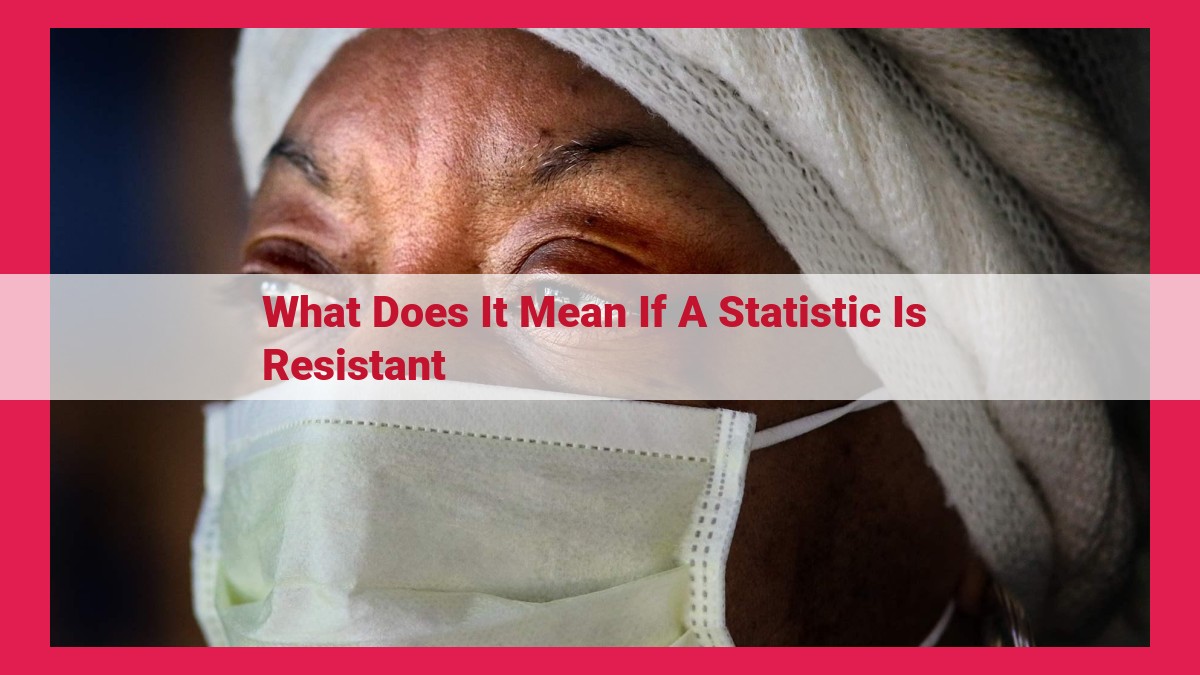
A statistic is resistant if it is not significantly affected by outliers or extreme values. Outliers are unusual data points that lie far from the majority of the data. Resistant statistics, such as the median and interquartile range, are not influenced by outliers, while non-resistant statistics, such as the mean and standard deviation, can be heavily skewed by outliers. It is important to choose appropriate statistics based on the expected level of outliers in the data to ensure reliable and accurate results.
Understanding Resistance in Statistics: A Guide to Robust Measures
In the realm of statistics, where data reigns supreme, resistance holds the key to resilience. Resistance, in this context, refers to the ability of statistical measures to withstand the impact of outliers, those extreme values that can significantly distort our understanding of data.
Outliers and Extreme Values: A Statistical Achilles Heel
Outliers are like mischievous imps in the world of data, capable of throwing off even the most seasoned statistician. These extreme values can arise from various sources, such as measurement errors, data entry mistakes, or simply the natural occurrence of rare events. Their presence can wreak havoc on traditional statistical measures, leading to biased and misleading conclusions.
Measures of Resistance: The Guardians of Stability
Fortunately, there are statistical measures that stand tall in the face of these outliers. These measures are known as resistant statistics, and they offer a much more stable representation of data in the presence of extreme values. Two key resistant measures are the median and the interquartile range (IQR).
The Median: A Rock-Solid Anchor in the Midst of Turbulence
The median is a true beacon of stability. It represents the middle value in a data set when arranged in ascending order. Unlike the mean (average), the median remains unfazed by outliers. No matter how extreme the outliers, the median remains firmly rooted in its position, providing a reliable indicator of the true center of the data.
The Interquartile Range: A Window into Data Variability
The interquartile range (IQR) is another resistant measure that focuses on the variability or spread of the data. It encompasses the middle 50% of the data, calculated as the difference between the upper and lower quartiles (the 25th and 75th percentiles). Like the median, the IQR is unaffected by outliers, offering a clear window into the true distribution of the data.
The Median: A Resilient Measure in the Face of Outliers
In the realm of statistics, navigating data anomalies can be a tricky task. Some statistical measures, like the mean and standard deviation, are highly susceptible to the influence of outliers – extreme values that can skew results and distort interpretation. Enter the median, a robust measure that stands firm against the impact of outliers, offering a more reliable representation of central tendency.
The median, simply put, is the middle value of a dataset when arranged in ascending or descending order. To calculate it, we follow this procedure:
- Sort the data: Arrange the values from smallest to largest.
- Identify the middle value: If there’s an odd number of values, the median is the middle one (e.g., in the set {1, 3, 5}, the median is 3). If there’s an even number, the median is the average of the two middle values (e.g., in {1, 2, 3, 4}, the median is (2 + 3) / 2 = 2.5).
The median’s superpower lies in its exceptional resistance to outliers. This means that even if extreme values are present in a dataset, they won’t significantly alter the median. Unlike the mean, which can be easily swayed by outliers, the median remains stable and unaffected.
To illustrate this resilience, let’s consider an example. Imagine a dataset of house prices: {100, 200, 300, 400, 1000}. The mean price is 340 – a decent representation. However, if we add an outlier of 10,000, the mean jumps to 2280, no longer reflective of the typical house price. The median, on the other hand, remains at 300, providing a more accurate picture of the central tendency.
Interquartile Range (IQR): A Resistant Measure of Data Spread
As we embark on our statistical journey, we often encounter data that exhibits varying degrees of variability. To accurately assess the central tendency and data dispersion, it is essential to select statistical measures that are not easily swayed by extreme values or outliers. One such resistant measure is the Interquartile Range (IQR).
The IQR provides a robust measure of data spread, unaffected by the presence of outliers. It is calculated as the difference between the upper quartile (Q3) and the lower quartile (Q1). Q3 represents the value at which 75% of the data lies below it, while Q1 represents the value at which 25% of the data lies below it.
Unlike the standard deviation, which can be heavily influenced by outliers, the IQR remains stable even in the presence of extreme values. This is because the quartiles are not affected by the values that lie beyond them. As a result, the IQR provides a more accurate representation of the variability within the main body of the data, excluding any potential distortions caused by outliers.
To illustrate the IQR’s resistance to outliers, consider the following data set:
10, 12, 15, 17, 20, 25, 30, 50, 100
The mean of this data set is 25.33, and the standard deviation is 18.67. However, the presence of the extreme value 100 significantly skews both these measures. The median, at 18, provides a more stable measure of central tendency, as it is not affected by the outlier.
The IQR, calculated as the difference between Q3 (25) and Q1 (12), is 13. This value remains unchanged even with the presence of the outlier 100, demonstrating the IQR’s resistance to extreme values.
In conclusion, the IQR is a powerful resistant measure of data spread that is not easily influenced by outliers. By focusing on the values within the main body of the data, the IQR provides a reliable assessment of data variability, unaffected by the presence of distortions caused by extreme values.
Mean: A Sensitive Measure of Central Tendency
When it comes to describing the center of a dataset, the mean is a widely used measure of central tendency. It’s calculated by adding up all the values in the dataset and dividing by the number of values. While the mean provides a meaningful representation for many datasets, it’s important to note its susceptibility to outliers – extreme values that lie far from the majority of the data.
Outliers and the Mean
Outliers, like a stray bullet in a firing range, can have a significant impact on the mean. They pull the mean away from its intended target – the true center of the data. This happens because the mean takes into account the value of every single data point, giving equal weight to both normal values and outliers.
For instance, consider a dataset representing the salaries of employees in a company. If there’s a single employee with an exceptionally high salary, the mean salary will be significantly inflated, creating a distorted view of the average salary in the company. In such cases, the mean fails to accurately represent the typical salary earned by the majority of employees.
Example: Outliers Skewing the Mean
Let’s say we have a dataset of test scores: {80, 85, 90, 95, 100}. The mean of this dataset is 90, which fairly represents the central tendency. Now, if we add an outlier – say, a student who scored an exceptionally high 150 – the mean jumps to 98. This drastic change in the mean is solely due to the presence of the outlier, which skews the average upwards.
Standard Deviation: A Sensitive Measure of Data Variability
In the realm of statistics, the standard deviation emerges as a crucial metric for gauging the extent to which data values deviate from their central tendency. It’s an indispensable tool for understanding the spread or dispersion of a dataset.
Mathematically, the standard deviation is calculated by first finding the mean or average of the data. Then, each data point’s difference from the mean is squared. The resulting squared differences are summed up and divided by the number of data points minus one (the sample size). Finally, the square root of this average squared difference yields the standard deviation.
While the standard deviation provides valuable insights into data variability, it possesses a critical vulnerability: sensitivity to outliers. Outliers are extreme values that lie far from the rest of the data. Even a single outlier can disproportionately inflate the standard deviation, making it appear that the data is more spread out than it actually is.
To illustrate this, consider a dataset of income values: [10, 12, 15, 18, 20, 25, 1000]. The mean of this dataset is roughly 143, and the standard deviation is approximately 259. However, if we remove the outlier (1000), the mean changes only slightly to 15.7, while the standard deviation drops significantly to 4.9. This dramatic reduction demonstrates the sensitivity of the standard deviation to even a single outlier.
Therefore, when dealing with datasets that may contain outliers, it’s crucial to use caution when interpreting the standard deviation. It’s always advisable to inspect the data for outliers and consider using alternative measures of variability, such as the interquartile range (IQR), which is more resistant to extreme values.
Outliers: The Unwanted Guests in Your Data
Imagine a dinner party where one guest arrives dressed in a flamboyant clown suit, while everyone else is in formal attire. This guest, with their eccentric behavior and unconventional appearance, is an outlier – a data point that stands out from the rest of the group.
In statistics, outliers are extreme values that deviate significantly from the majority of the data. They can be caused by measurement errors, sampling fluctuations, or simply the presence of unusual observations.
Outliers can have a profound impact on data analysis. They can distort the mean, which is a common measure of central tendency (the average value of a data set). Outliers can also inflate the standard deviation, which is a measure of data dispersion (how spread out the data is).
Consider this example: A data set contains the test scores of 100 students. The mean score is 80. However, one student scored 100, an outlying value that is 20 points higher than the next highest score. This outlier pulls the mean up to 81, which is not an accurate representation of the typical student’s performance.
Similarly, outliers can artificially increase the standard deviation. If the data set in the previous example contained only 99 students, the standard deviation would be 10. However, the inclusion of the outlier increases the standard deviation to 12. This suggests that the data is more spread out than it actually is.
In contrast to the mean and standard deviation, the median and interquartile range (IQR) are resistant statistics. They are not affected by outliers, making them more reliable measures of central tendency and data dispersion when dealing with data that may contain extreme values.
The median is the middle value of a data set when arranged in ascending order. It is not influenced by outliers because they fall either above or below the median. The IQR is the difference between the third quartile (Q3) and the first quartile (Q1). It is a measure of the spread of the middle 50% of the data, ignoring the extremes.
Outliers can be valuable in certain situations, such as identifying fraudulent transactions or detecting rare events. However, when it comes to drawing general conclusions about a data set, it is crucial to be aware of their potential impact and to use resistant statistics that are not easily swayed by extreme values.