Observable Unit And Unit Of Analysis In Observational Studies: Optimizing Research For Accuracy
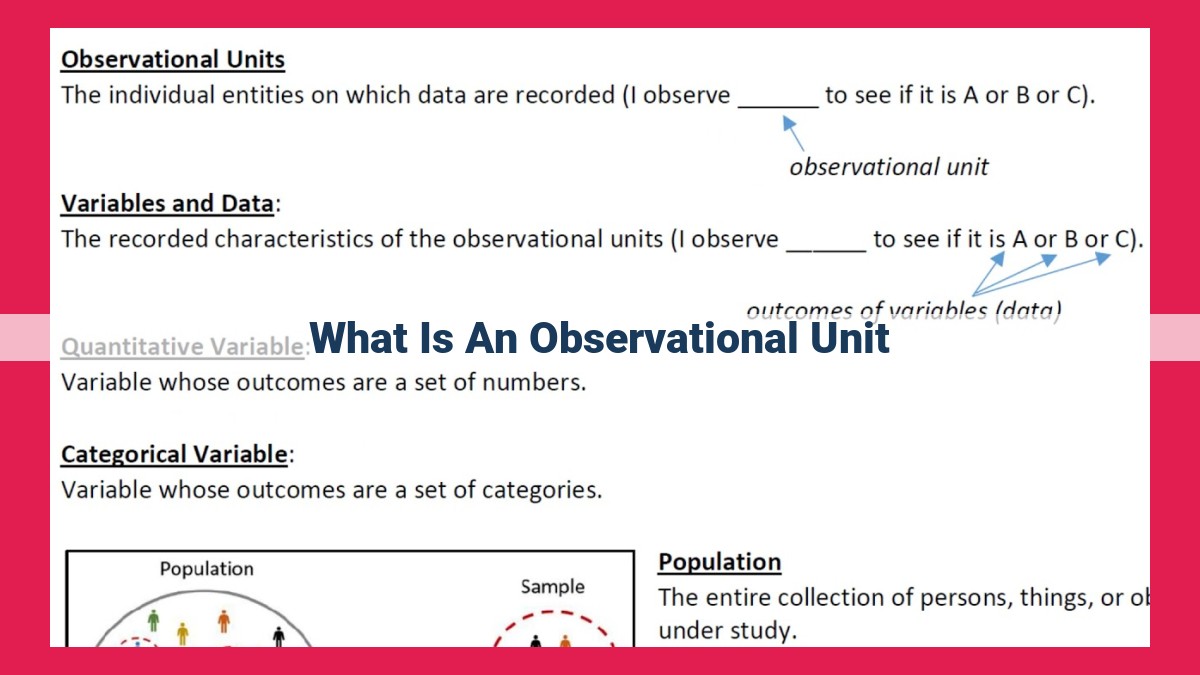
An observational unit is the fundamental subject of an observational study. It can be an individual entity (e.g., person, animal, object) or a group (e.g., team, community). The choice of observational unit depends on the research question and determines the level of data collection (unit of analysis). Incorrectly choosing the observational unit or unit of analysis can lead to biases, such as aggregation bias (distortion of individual-level data when aggregated to group level) or ecological fallacy (inferring individual characteristics from group-level data). Understanding these concepts is crucial in observational study design to ensure valid and meaningful results.
Understanding Observational Units: The Foundation of Observational Studies
Imagine you’re conducting a study to determine the relationship between smoking and lung cancer. What would be the observational unit in this scenario? That’s right, it’s the individual smoker or non-smoker. They are the primary subjects of your observation.
Observational units are the cornerstone of data collection and analysis in any observational study. Understanding them thoroughly is vital for ensuring the accuracy and validity of your findings. They represent the entities you observe and collect data from to draw inferences about a larger population.
In the example above, the observational unit is an individual person. However, observational units can also be groups, such as teams, organizations, or communities. The choice of observational unit depends on the research question and the data available.
Types of Observational Units: Exploring the Focus of Observation
In the realm of observational studies, identifying the primary subject of observation is crucial. These subjects, known as observational units, come in diverse forms, including individuals and groups. Understanding these observational units is essential for accurate data collection and valid analysis.
Individual Units: The Foundation of Observation
Individual observational units are the fundamental building blocks of many observational studies. These units can be individuals, animals, or even objects. For instance, a researcher studying the effects of a new exercise program may observe individual participants’ fitness levels over time.
Group Units: Collective Entities under Scrutiny
Observational units can also encompass larger groups, such as teams, organizations, or communities. These units represent collective entities with distinct characteristics and behaviors. By studying such groups, researchers can gain insights into organizational dynamics, social trends, or population-level outcomes.
Implications of Observational Unit Choice
The choice of observational unit profoundly influences the nature and interpretation of the study. For example, focusing on individual units allows for a fine-grained analysis of individual-level characteristics and experiences. On the other hand, group-level observational units provide aggregate data that can reveal patterns and trends within the group as a whole.
Understanding the implications of observational unit choice is paramount to ensure that the study design aligns with the research questions and that the findings accurately reflect the phenomena under investigation.
Unit of Analysis: The Foundation of Observational Study Interpretation
Imagine yourself embarking on an exciting journey of data exploration, where the unit of analysis serves as your guiding compass. It’s the level at which you gather and interpret your data, shaping the very essence of your findings.
Let’s unravel the mysteries of this crucial concept. The unit of analysis can be an individual, a group, or even a population. Each holds unique significance, like pieces of a puzzle fitting together to paint a complete picture.
For instance, if you’re studying voting patterns, you might choose individuals as your unit of analysis. This allows you to examine individual voting behaviors and identify trends among different demographic groups. On the other hand, if you’re analyzing the performance of a company, you might select groups (such as departments) as your unit of analysis. By comparing group performance, you can pinpoint areas for improvement and make informed decisions.
The choice of unit of analysis has a profound impact on your results. Consider this: if you investigate the educational attainment of a population by analyzing group data (e.g., average education levels of different cities), you might overestimate the proportion of individuals with high education. This phenomenon is known as aggregation bias, where group-level data can lead to distorted conclusions.
Similarly, the ecological fallacy occurs when you draw conclusions about individuals based on group-level data. For example, assuming that all residents of a wealthy neighborhood are highly educated can lead to erroneous inferences.
Understanding the unit of analysis is paramount in any observational study. It’s the lens through which you view your data, ensuring that your findings accurately reflect the phenomena you’re investigating. By carefully considering the appropriate unit of analysis, you lay the foundation for valid and insightful conclusions, paving the path to knowledge discovery.
Aggregation Bias: Unveiling the Pitfalls of Group-Level Data
Have you ever wondered if the data you’re analyzing paints an accurate picture of the individuals it represents? When you group data from individuals into categories or groups, you may inadvertently introduce distortions known as aggregation bias. Let’s delve into this often-overlooked phenomenon to understand its impact on data analysis.
Types of Aggregation Bias
Aggregation bias occurs when individual-level data is aggregated into group-level data. This can lead to two distinct types of bias:
-
Upward bias (overestimation): This occurs when the group-level data overestimates the true average of the individual-level data. Imagine you’re studying the average income of a city. If you have individual income data, you’ll find that some people earn more than the average while others earn less. However, when you aggregate this data into groups (such as income brackets), the average income for each group will likely be higher than the true average for all individuals.
-
Downward bias (underestimation): Conversely, downward bias occurs when the group-level data underestimates the true average of the individual-level data. This can happen when the variability within groups is ignored. For example, if you’re analyzing the average happiness of employees in a company, you may find that some departments are happier than others. However, if you only look at the company-wide average, you may underestimate the true happiness of the individuals.
Causes of Aggregation Bias
Aggregation bias arises when:
- Variability within groups is not accounted for: When aggregating data, it’s crucial to consider the differences between individuals within groups. Overlooking this variability can lead to misleading conclusions.
- Group-level characteristics are incorrectly assumed to represent individuals: Assuming that all members of a group are identical can lead to erroneous inferences. Individuals within groups may have diverse experiences and characteristics that are not captured by the group-level data.
Consequences of Aggregation Bias
Aggregation bias can have significant consequences for data analysis and decision-making:
- Inaccurate conclusions: Biased data can lead to incorrect interpretations and inaccurate conclusions.
- Policy decisions based on flawed evidence: When policymakers rely on biased data, they may make decisions that do not accurately reflect the needs of individuals.
- Wasted resources: Time and resources invested in research based on biased data are largely ineffective.
Avoiding Aggregation Bias
To avoid aggregation bias, consider the following strategies:
- Use disaggregated data when possible: Analyzing individual-level data can help mitigate the effects of aggregation bias.
- Control for group-level characteristics: When analyzing group-level data, account for the variability within groups by controlling for relevant group characteristics.
- Be aware of the limitations of group-level data: Understand that group-level data may not fully represent the experiences and characteristics of individuals.
Ecological Fallacy: Unveiling the Pitfalls of Group-Level Data
Imagine you’re crafting a study to analyze the relationship between education and income. You gather data from counties across the nation and discover a fascinating correlation: counties with higher average education levels tend to boast higher average incomes. However, when you delve into individual data, you’re met with a puzzling paradox. Individuals within these high-income counties often have lower education levels than individuals in counties with lower average incomes.
Introducing the Ecological Fallacy
This puzzling discrepancy is a classic example of the ecological fallacy. It’s a misleading inference that assumes individual characteristics mirror group characteristics. Simply put, the ecological fallacy occurs when you draw conclusions about individuals based solely on group-level data.
Downward Bias and Upward Bias: Distorting the Truth
Within the realm of the ecological fallacy lie two distinct forms of bias:
- Downward Bias (Overgeneralization): This occurs when the group-level data suggests individuals fare better than they truly do.
- Upward Bias (Undergeneralization): Conversely, this arises when group data portrays individuals as faring worse than they actually do.
Downward Bias Unraveled
Consider the jarring example of a study suggesting that countries with higher average happiness levels report lower crime rates. While this may hold true at the national level, it could mask a sinister truth: within those happy countries, certain marginalized groups might endure disproportionately high crime rates.
Upward Bias Exposed
Now, envision a study claiming that states with stricter gun control laws have higher murder rates. Again, while this may be accurate at the state level, it could conceal a different reality: individuals residing in neighborhoods with feeble gun control might experience lower murder rates than those in areas with stringent laws.
Preventing the Ecological Trap
To avoid the pitfalls of the ecological fallacy, it’s imperative to recognize its presence and employ appropriate remedies:
- Consider individual data: Supplement group-level data with individual-level data to provide a comprehensive understanding.
- Examine within-group variability: Acknowledge that groups are not monolithic—individuals within them may exhibit diverse characteristics.
- Use appropriate statistical methods: Utilize techniques such as multilevel modeling to account for the hierarchical nature of data and minimize biases.
The ecological fallacy is a treacherous pitfall that can lead to erroneous conclusions. By understanding its mechanisms and implementing safeguards, researchers can avoid these biases and uncover the true relationships between group-level and individual characteristics. This knowledge empowers us to make more informed decisions and shape policies that effectively address societal issues.