Unlock The Power Of Nlp: Enhancing Human-Computer Communication And Driving Business Insights
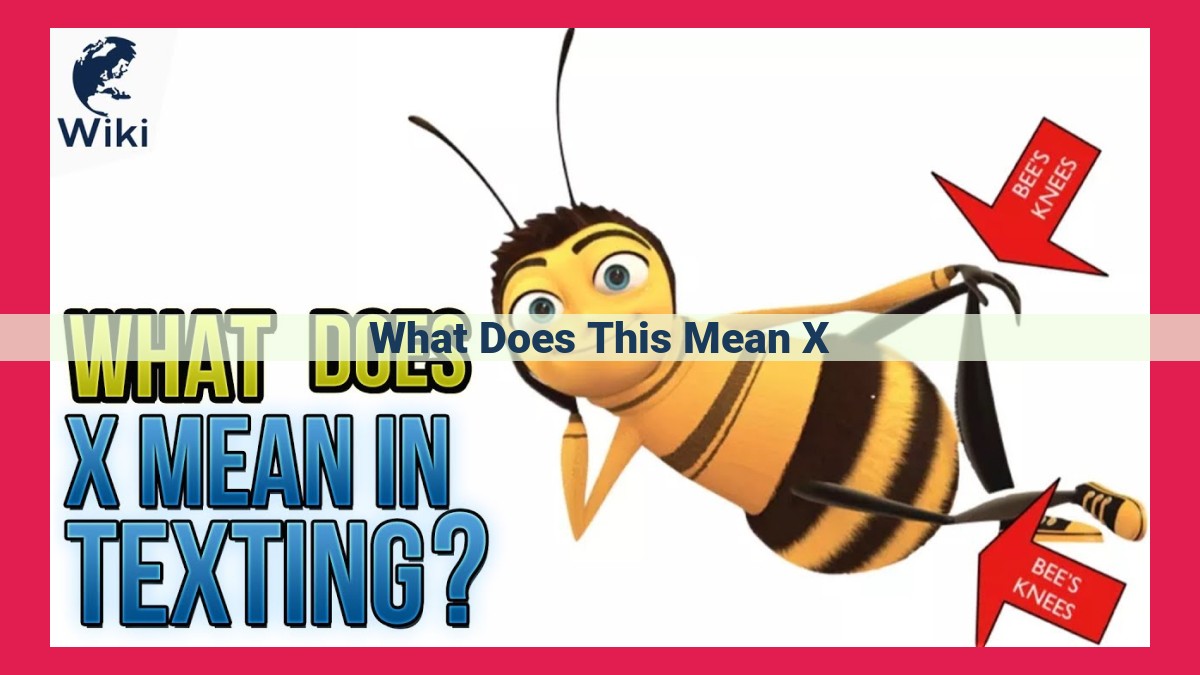
NLP enables computers to understand human language by exploring contextual understanding, machine learning, and related concepts like data mining and machine translation. Machine learning algorithms contribute to NLP tasks like language understanding and generation. NLP finds practical applications in fields such as customer service and sentiment analysis, and advancements continue to expand its capabilities and potential.
Understanding Natural Language Processing (NLP)
In the realm of communication, humans have an extraordinary ability to comprehend the nuances and complexities of language. But what if we could empower computers with the same level of linguistic understanding? That’s where Natural Language Processing (NLP) comes into play.
NLP is the bridge between human language and the digital world. It’s the process of enabling computers to understand, interpret, and interact in a way that mirrors human-to-human communication. By analyzing the structure, semantics, and context of text and speech, NLP unlocks a new level of interaction between humans and machines.
NLP is the key to creating applications that can understand customer inquiries, extract meaningful insights from unstructured data, and generate coherent, human-like text. Its versatility extends across industries, from healthcare and finance to social media and e-commerce.
Imagine a world where computers can comprehend our language, the same way we understand each other.
With NLP, this vision becomes a reality. From chatbots that answer questions and provide support to self-driving cars that interpret road signs and commands, NLP is revolutionizing the way we interact with technology. As we delve deeper into this fascinating field, let’s explore how NLP makes it possible for computers to understand the intricate world of human language.
Understanding Contextual Understanding in NLP
Contextual understanding lies at the heart of Natural Language Processing (NLP), empowering computers to decipher the nuances and meanings of human language. Unlike simple keyword matching, NLP seeks to comprehend the context in which words are used, considering the surrounding text, the relationships between words, and the broader discourse.
Semantics, the study of word meanings, plays a pivotal role in contextual understanding. NLP algorithms analyze the denotations (literal meanings) and connotations (implied meanings) of words to derive their intended interpretations. Pragmatics delves into the use of language in specific situations, examining how context influences word choice, tone, and interpretation.
Discourse analysis uncovers the structure and coherence of text, identifying relationships between sentences and paragraphs. It reveals how writers organize their ideas, establish connections, and maintain the flow of information. Cohesion refers to the linguistic devices that tie text together, such as pronouns, conjunctions, and lexical chains, ensuring that the reader can follow the narrative seamlessly.
By integrating semantics, pragmatics, discourse analysis, and cohesion, NLP algorithms can grasp the intricate tapestry of human language, unraveling its underlying meanings and making sense of the world around us.
Machine Learning and Natural Language Processing (NLP)
Natural Language Processing (NLP) is a fascinating field that enables computers to comprehend human language like never before. Machine learning plays a pivotal role in this revolutionary technology, opening up new possibilities for interaction between people and machines.
The Power of Machine Learning in NLP
Machine learning, a subset of artificial intelligence, empowers NLP algorithms to learn from data and uncover intricate patterns within language. These algorithms can process vast amounts of text, identifying statistical correlations and extracting meaningful insights that would be impossible for humans to decipher manualmente.
Pattern Recognition and Prediction
One key application of machine learning in NLP is pattern recognition. By analyzing large datasets of human language, algorithms can learn to recognize specific linguistic patterns, such as grammatical structures and word sequences. This knowledge allows them to make informed predictions about the meaning and context of new text, aiding tasks like text classification, information retrieval, and machine translation.
For example, a machine learning algorithm trained on a corpus of customer service transcripts can recognize patterns associated with positive or negative customer feedback. This enables the algorithm to predict the sentiment of new customer reviews, providing businesses with valuable insights into customer satisfaction.
Expanding the Scope of NLP: Related Concepts and Their Significance
While Natural Language Processing (NLP) empowers computers to unravel the complexities of human language, a host of related concepts play pivotal roles in enhancing its capabilities. These concepts encompass a wide range of disciplines, extending NLP’s reach beyond its core functionality.
Data Mining and Text Analysis: Treasure Troves of Insights
Data mining and text analysis serve as essential tools for extracting meaningful patterns and knowledge from vast amounts of textual data. These techniques unlock the potential of NLP by enabling the identification of hidden trends, patterns, and relationships within text, empowering machines to uncover insights that would otherwise remain concealed.
Machine Translation: Bridging Language Barriers
Machine translation empowers NLP to transcend language barriers, enabling the seamless conversion of text from one language to another. This capability opens up a world of possibilities for cross-cultural communication, allowing individuals to access information and connect with others regardless of linguistic differences.
Text Classification: Categorizing Data with Precision
Text classification empowers NLP to categorize and organize text data into predefined categories. This process enhances NLP’s ability to filter, prioritize, and analyze text, enabling a diverse range of applications, from spam filtering to sentiment analysis.
Information Retrieval: Navigating the Sea of Knowledge
Information retrieval empowers NLP to locate and retrieve specific information from vast collections of text. This capability forms the foundation of search engines, allowing users to quickly and efficiently access the information they seek. NLP’s ability to understand the context and relevance of text enables highly accurate and personalized search results.
Question Answering: Unlocking Knowledge on Demand
Question answering systems leverage NLP’s capabilities to provide direct answers to specific questions posed in natural language. These systems tap into vast knowledge bases and employ advanced NLP techniques to extract relevant information and generate concise and informative responses, empowering users to access knowledge instantly.
Machine Learning Techniques in NLP: Empowering Computers to Understand and Generate Language
Supervised Learning: Guiding Algorithms with Labeled Data
In supervised learning, NLP algorithms are trained on labeled data, where each data point is paired with a corresponding label. This labeled data allows the algorithm to learn the relationship between input text and desired output, such as classifying text into specific categories or translating text from one language to another.
Unsupervised Learning: Unraveling Patterns without Labels
Unsupervised learning differs from supervised learning in that it operates on unlabeled data. The algorithm must identify patterns and structures within the data on its own, without explicit guidance. This approach is often used for tasks like clustering similar text documents or identifying hidden topics within large text collections.
Reinforcement Learning: Fine-tuning Algorithms through Trial and Error
Reinforcement learning leverages a feedback loop to improve an algorithm’s performance over time. The algorithm interacts with its environment, observes the consequences of its actions, and adjusts its behavior based on rewards or penalties it receives. This iterative process enables NLP algorithms to adapt and learn from their interactions with language data.
Contributions to NLP Tasks: A Game-Changing Collaboration
These machine learning algorithms play vital roles in various NLP tasks:
- Language Understanding: Algorithms analyze text to extract meaning, identify entities, and perform sentiment analysis.
- Language Generation: Algorithms create new text, summarize documents, and translate languages.
Implementation and Applications of Natural Language Processing (NLP)
NLP has found its way into various domains, significantly transforming business processes and redefining customer interactions. Let’s delve into some of its practical applications:
Customer Service
NLP-powered chatbots have become ubiquitous in customer service, offering 24/7 assistance and resolving queries efficiently. Their ability to understand natural language enables them to engage in human-like conversations, providing personalized responses and streamlining the entire support experience.
Information Extraction
NLP algorithms can automatically extract structured data from unstructured text. This has revolutionized the way businesses process unstructured information, such as news articles, customer reviews, and social media posts. The extracted data can be used for market research, sentiment analysis, and knowledge graph construction.
Sentiment Analysis
NLP techniques are employed to analyze the emotional undertones of text. This enables businesses to understand customer feedback, monitor brand reputation, and gauge public opinion. Sentiment analysis aids in identifying dissatisfied customers, detecting brand mentions, and gaining valuable insights into customer sentiment.
Text Summarization
NLP algorithms can condense lengthy text into concise summaries, capturing the key points and preserving the context. This is particularly beneficial for busy professionals who need to quickly grasp the gist of emails, documents, and reports. Text summarization also helps improve search results, making it easier to locate relevant information.
Beyond Industry Applications
NLP is revolutionizing various industries by automating tasks, enhancing decision-making, and improving customer experiences. In healthcare, NLP is used for patient record analysis and drug discovery. In finance, it enables fraud detection and risk assessment. In education, NLP powers personalized learning and content creation. The applications of NLP are vast and continue to expand as the field evolves.
Challenges and Future of NLP
Despite remarkable advancements, Natural Language Processing (NLP) faces ongoing challenges that hinder its full potential and drive ongoing research efforts.
Addressing Ambiguity and Context Dependence
NLP systems often struggle to resolve ambiguity, where words or phrases can have multiple meanings depending on context. This challenge arises due to the context dependence of language, where the intended meaning can vary based on the surrounding words and discourse. To overcome this, researchers are exploring techniques like coreference resolution and discourse analysis to capture the relationships between words and phrases within a context.
Handling Diverse Language Styles
NLP models are typically trained on large datasets that represent a specific language style or register. However, language can vary significantly across domains and genres, posing challenges for NLP systems to handle diverse styles effectively. This issue is particularly evident in tasks like sentiment analysis and text classification, where models may perform differently on datasets with different linguistic characteristics. Researchers are working on transfer learning and domain adaptation techniques to enable NLP models to adapt to new styles and enhance their robustness.
Advancements and Future Directions
Despite these challenges, NLP is rapidly evolving, driven by advancements in machine learning and computational linguistics. Researchers are exploring novel approaches to enhance NLP capabilities, including:
-
****Large Language Models (LLMs)**: LLMs, such as GPT-3 and LaMDA, demonstrate impressive language understanding and generation capabilities. These models are trained on vast text datasets and can handle a wide range of NLP tasks.
-
****Explainable AI (XAI)**: XAI techniques provide insights into the decision-making processes of NLP models, helping practitioners understand how these models arrive at their predictions. This transparency is crucial for building trust and enabling the adoption of NLP in critical applications.
-
****Multilingual NLP**: NLP research is expanding beyond English, focusing on developing models that can process and understand multiple languages. This advancement will empower NLP to cater to a global audience and facilitate cross-cultural communication.
NLP is a rapidly growing field with immense potential to transform our interactions with technology and unlock new frontiers in language understanding and communication. By addressing the challenges of ambiguity and context dependence, handling diverse language styles, and embracing advancements like LLMs, XAI, and multilingual NLP, we can expect even greater strides in the future of NLP.