Understanding The Significance Of Negative T-Values In Hypothesis Testing: A Guide For Statistical Analysis
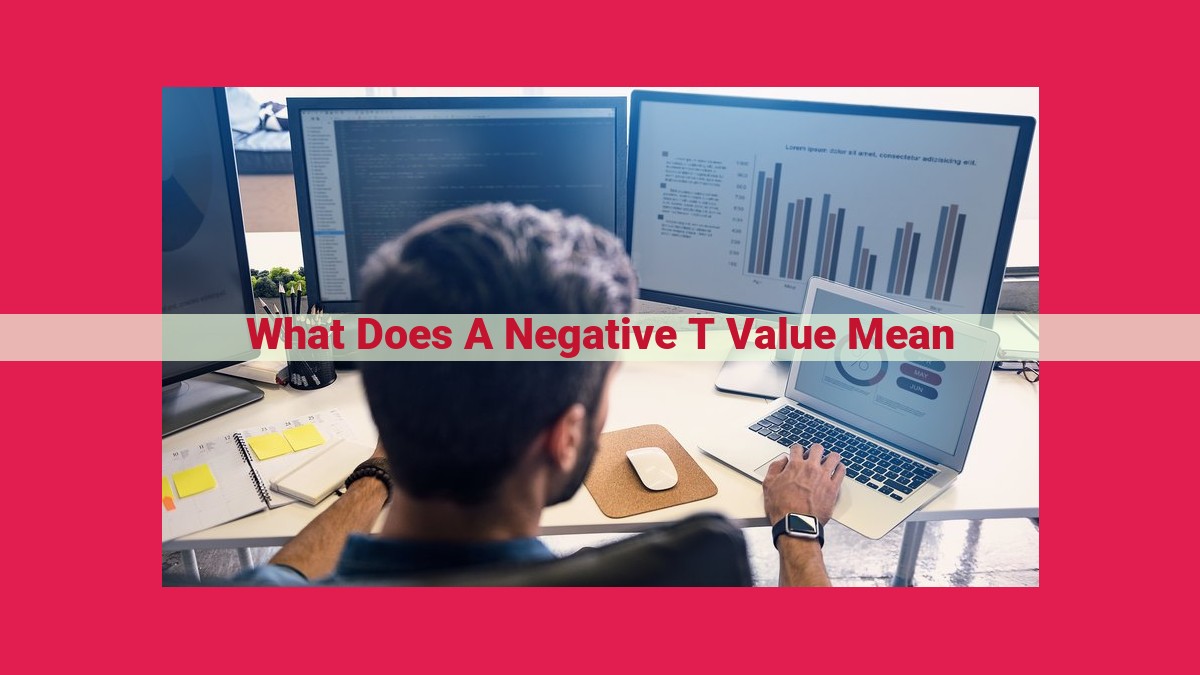
A negative t-value in hypothesis testing indicates that the observed difference between the sample mean and the hypothesized mean falls below the expected value, assuming the null hypothesis (H0) is true. This suggests that the predicted difference in the direction of the alternative hypothesis (Ha) is unlikely. When the observed t-value is negative, it lends support to H0 and suggests that the difference observed in the sample could have occurred by chance, given the level of significance (alpha) set for the test.
The Concept of Level of Significance: Unlocking the Mysteries of Hypothesis Testing
In the realm of research and data analysis, hypothesis testing plays a pivotal role in confirming or rejecting our assumptions about the world. And at the heart of hypothesis testing lies a fundamental concept known as the level of significance. Let’s dive into its meaning and significance.
What is the Level of Significance (alpha)?
Picture this: You’re flipping a coin and want to test if it’s fair. You set a threshold of 5% (or 0.05) as the level of significance. This means that if the coin lands heads more than 5% of the time, you’ll reject the hypothesis that it’s fair.
The level of significance (alpha) is the maximum probability of making a Type I error, which occurs when we reject the null hypothesis when it is actually true. In other words, it’s the risk we’re willing to take to be wrong. A lower alpha indicates a stricter threshold and reduces the likelihood of a Type I error.
The Enigma of the Negative T-Value in Hypothesis Testing
In the realm of statistics, hypothesis testing is a dance between assumptions and outcomes. One crucial element in this intricate choreography is the t-value, a measure that reveals the likelihood that our observed results differ from what we would expect under the assumption of no true difference, known as the null hypothesis.
While a positive t-value suggests that the observed difference is likely to be due to a true difference between groups, a negative t-value presents a contrasting scenario. It indicates that the observed difference is in the opposite direction from what we had initially predicted.
Let’s unravel this enigma with an example: Suppose we have two groups, A and B, and we want to determine if Group A performs better than Group B on a certain task. Our null hypothesis states that there is no difference between the two groups (A = B).
If we obtain a negative t-value, it means that the observed difference between the groups is in the opposite direction to our predicted difference. In other words, Group B performed better than Group A, contrary to our initial hypothesis.
This negative t-value supports the null hypothesis, indicating that there is no statistically significant difference between Group A and Group B. It challenges our initial belief that Group A would outperform Group B, casting doubt on the existence of a true difference between the groups.
Understanding the implications of a negative t-value is essential for making sound scientific judgments. It safeguards us from drawing erroneous conclusions and ensures the integrity of our research findings.
Related Concepts
- P-value: Definition and importance in hypothesis testing.
- Hypothesis Testing: Brief overview of the process.
- Null Hypothesis: Definition and role in hypothesis testing.
- Alternative Hypothesis: Definition and role in hypothesis testing.
Understanding Hypothesis Testing: The Significance of Related Concepts
In the realm of scientific inquiry, hypothesis testing plays a pivotal role in verifying our beliefs and assumptions. However, to delve into the intricacies of this process, a thorough understanding of several key concepts is essential, namely the p-value, hypothesis testing, null hypothesis, and alternative hypothesis.
P-Value: A Measure of Evidence Against the Null
The p-value is a statistical measure that quantifies the likelihood of observing a result as extreme as or more extreme than the one we obtained from our data, assuming that the null hypothesis is true. In other words, the p-value tells us how well our data supports the null hypothesis. The lower the p-value, the less likely it is that our data would have come from a population where the null hypothesis is true. This indicates that our data provides strong evidence against the null hypothesis and therefore supports our alternative hypothesis.
Hypothesis Testing: A Structured Approach to Scientific Inquiry
Hypothesis testing is a structured process of evaluating whether there is sufficient evidence to reject a null hypothesis. The null hypothesis is a statement that suggests there is no difference or effect. The alternative hypothesis, on the other hand, proposes that there is a difference or effect. By testing the null hypothesis, we can assess whether our data provides enough support to dismiss it in favor of the alternative hypothesis.
Null Hypothesis: The Placeholder of No Effect
The null hypothesis is often denoted as H₀ and represents the assumption that there is no significant difference or effect. It serves as a placeholder against which we compare our data. Rejecting the null hypothesis means concluding that our data provides sufficient evidence against the idea of no difference or effect.
Alternative Hypothesis: The Statement of a Difference
The alternative hypothesis, denoted as *H₁, is the statement that there is a significant difference or effect. If we reject the null hypothesis, we accept the alternative hypothesis. The alternative hypothesis is our research question or the prediction we are testing with our data.
Understanding One-Tailed and Two-Tailed Hypothesis Tests
In the realm of hypothesis testing, selecting the appropriate type of test is crucial. Enter the one-tailed and two-tailed tests, each with distinct purposes and implications.
A one-tailed test, also known as a directional test, is employed when the researcher has a prior expectation regarding the direction of the difference between two groups or populations. It’s akin to saying, “I predict that Group A will perform better than Group B.” Based on this prediction, the researcher sets a one-sided level of significance (alpha). If the results align with the prediction, the researcher can conclude support for the hypothesis.
In contrast, a two-tailed test, or non-directional test, is used when the researcher has no prior expectation about the direction of the difference. It’s like saying, “I have no clue which group will perform better.” In this case, the researcher establishes a two-sided level of significance, ensuring sensitivity to differences in either direction. The results may either support the hypothesis or fail to reject the null hypothesis.
Choosing between a one-tailed and two-tailed test depends on the research question and the availability of prior knowledge or theoretical support for a specific direction. One-tailed tests provide greater power when the prediction is accurate, but increase the risk of a Type II error (failing to detect a true difference). Two-tailed tests are more conservative and protect against Type II errors, but require a larger sample size to achieve the same level of power.
Remember, the level of significance (alpha) plays a pivotal role in both types of tests. It represents the probability of rejecting the null hypothesis when it is actually true. Lower alpha values indicate a more stringent standard, making it harder to reject the null hypothesis.
By carefully considering the research question and the available evidence, researchers can select the appropriate hypothesis test to draw meaningful conclusions from their data.
**The Power of Hypothesis Testing: Unveiling Errors and Understanding Significance**
In the intricate world of statistics, hypothesis testing plays a crucial role in revealing meaningful patterns from data. One key component of this process is the concept of the level of significance, which sets a threshold for determining whether observed results are due to chance or to a meaningful underlying difference.
However, the story doesn’t end there. Hypothesis testing involves a delicate balance between two types of errors that can have significant consequences for our interpretations: Type I and Type II errors. By understanding these concepts, we gain a deeper appreciation for the power and limitations of statistical inference.
The Power of a Test: Embracing the True
The power of a test represents the probability of correctly rejecting a false null hypothesis (the hypothesis that assumes no difference). A powerful test is more likely to detect a true difference, reducing the risk of missing something important.
Type I Error: False Alarms in the Statistical Realm
Imagine a scenario where a hypothesis test suggests that an experimental treatment has a statistically significant effect on a measured outcome. However, upon further scrutiny, it turns out that the observed difference is purely a matter of chance. This is known as a Type I error.
Type I errors occur when we reject a true null hypothesis, leading us to believe that there is a difference when there isn’t. Consequences of such errors can range from wasted resources and incorrect conclusions to potentially misleading policy decisions.
Type II Error: Overlooking the Obvious
Now, let’s flip the script. Suppose we conduct a hypothesis test and fail to reject the null hypothesis, concluding that there is no significant difference. However, in reality, a meaningful difference exists that we missed. This is known as a Type II error.
Type II errors occur when we fail to reject a false null hypothesis, leading us to incorrectly conclude that there is no difference when there is one. Consequences of such errors can be detrimental, especially in areas like medical research or financial decision-making.
Navigating the Statistical Maze
To minimize the risk of both Type I and Type II errors, researchers and statisticians must carefully consider factors such as sample size, effect size, and the level of significance. Optimizing these elements can enhance the power of a test, reducing the likelihood of both false positives and false negatives.
Understanding the power of a test and the potential for errors is paramount in conducting rigorous and meaningful statistical analyses. By embracing this knowledge, researchers can make more informed decisions, ensuring that their findings accurately reflect the underlying patterns in data.