Mu (Μ): The Key To Understanding Data Distribution In Statistics
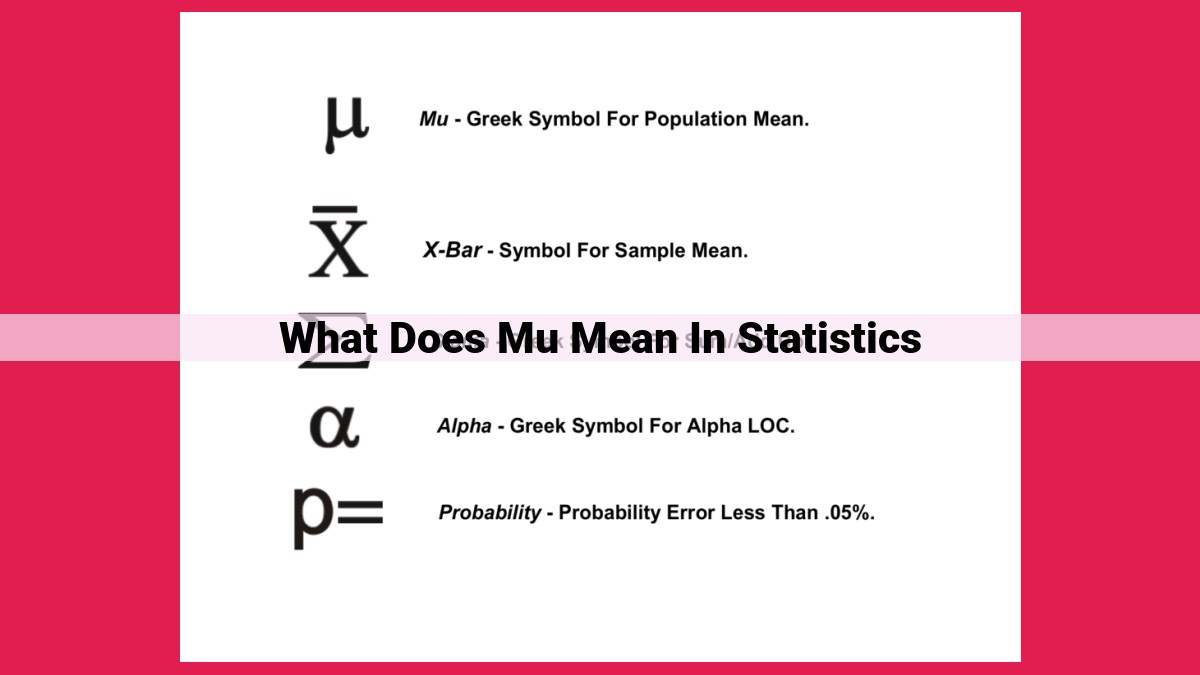
Mu (µ) is a crucial concept in statistics, representing the average or central tendency of a dataset. It reflects the typical value around which data points are distributed, providing a measure of how closely the data clusters around this average. Mu serves as the basis for understanding data distribution, allowing comparisons between different datasets and drawing inferences about the population from which the sample was drawn. It’s vital for hypothesis testing, estimation, and modeling, making it an essential tool for statistical analysis and decision-making.
- Define mu as the measure of central tendency or “average” in statistics.
- Explain its significance in understanding data distribution.
Understanding Mu (µ): The Foundation of Statistics
In the realm of statistics, mu (µ) stands as a cornerstone concept, unveiling the secrets of data distribution and providing a foundation for meaningful analysis. It’s a measure of central tendency, the elusive “average” that encapsulates the heart of a dataset. But what exactly is mu, and why is it so important?
Defining Mu: The Essence of Averages
Envision a group of students taking a test. Each student’s score represents a single data point, and the average of all these scores is the population mean, the true central tendency. However, in real-world scenarios, we often don’t have access to every single data point. Instead, we rely on sample means, calculated from a smaller subset of the population. These sample means give us an estimate of the elusive population mean.
Mu’s Significance: Illuminating Data Distribution
Mu serves as a beacon, guiding us through the murky depths of data. It pinpoints central tendency, the middle ground around which the data points congregate. Understanding central tendency is critical because it provides a glimpse into how the data is spread out. A dataset with a high mu indicates a concentration of data points towards the higher end of the spectrum, while a low mu suggests a bias towards the lower end.
Related Concepts: A Tapestry of Measures
Mu exists alongside a tapestry of related concepts that enrich our statistical vocabulary. The term mean often refers to mu, but it can also encompass other types of averages, such as weighted means, which consider the importance of individual data points, and geometric means and harmonic means, each with its unique applications.
Mu’s Vital Role in Statistical Analysis
Mu is the lifeblood of statistical analysis. It underpins hypothesis testing, where we compare our observations to a hypothesized mean. It empowers us to estimate population parameters, drawing inferences from sample data. And it guides us in creating statistical models that capture the underlying patterns in data.
Mu (µ) is the cornerstone of statistics, providing a profound understanding of data distribution and serving as an indispensable tool for statistical inference. Whether we aim to compare datasets, estimate population parameters, or unravel complex relationships, mu shines as a guiding light, illuminating the path towards informed conclusions and data-driven insights.
Population Mean and Sample Mean: Understanding the True and Estimated Averages
In the realm of statistics, we often encounter the concept of central tendency, a crucial measure that provides insights into the behavior of a dataset. One prominent representative of central tendency is the mean, also known as mu (µ). However, when working with real-world data, we rarely have access to the entire population under study. Instead, we rely on samples, a subset of the population, to make inferences about the true population characteristics. This is where the distinction between population mean and sample mean becomes essential.
The population mean (µ) represents the true average of the population. It is the arithmetic sum of all values in the population divided by the number of elements. However, in practice, obtaining the population mean can be challenging, especially for large or infinite populations.
Enter the sample mean (x̄), an estimate of the population mean calculated from a representative sample of the population. The sample mean is the arithmetic sum of all values in the sample divided by the sample size.
Sample means play a pivotal role in statistical inference. By observing the behavior of sample means, statisticians can make inferences about the behavior of the population mean. For instance, if we take multiple random samples from a population, we expect the sample means to vary around the population mean. The Central Limit Theorem states that as the sample size increases, the distribution of sample means approaches a normal distribution regardless of the distribution of the population.
This understanding enables statisticians to use sample means to test hypotheses, make predictions, and estimate population parameters. For example, in market research, a company might survey a sample of customers to estimate the average satisfaction level with their product. By comparing the sample mean to a hypothesized population mean, the company can determine if their customers are generally satisfied with the product.
In summary, the population mean represents the true average of the entire population, while the sample mean is an estimate of the population mean obtained from a representative sample. By understanding the relationship between population means and sample means, statisticians can effectively draw conclusions about the population based on sample data, opening doors to valuable insights and decision-making in various fields.
Central Tendency and Average: Understanding Mu (μ)
In the realm of statistics, mu (μ) reigns supreme as the measure of central tendency, the elusive “average” that embodies the essence of a dataset. Just as a compass points to true north, mu guides us towards the heart of data distribution.
Types of Averages:
Beyond mu, the arithmetic mean, a.k.a. simply “average,” there lies a constellation of its brethren:
-
Weighted mean: A variant of mu that assigns different weights to data points, reflecting their importance.
-
Geometric mean: Ideal for multiplicative data, where multiplying values is more meaningful than adding.
-
Harmonic mean: Useful in scenarios where taking the inverse of values is appropriate, such as calculating average speed.
Understanding Central Tendency:
Mu stands as a sentinel, guarding the central point of a dataset. It tells us where most data values congregate, providing a valuable snapshot of the data’s distribution. This knowledge empowers us to make inferences, predict outcomes, and draw meaningful conclusions.
Delving into the World of Mu (µ): The Heartbeat of Statistics
In the vast realm of statistics, there exists a pivotal symbol that holds immense significance: Mu (µ). This enigmatic Greek letter represents an elusive value, the elusive “average” that lies at the core of our understanding of data. Join us as we delve into the fascinating world of Mu and its indispensable role in statistical analysis.
Mu: The Measure of Central Tendency
Mu, in its essence, captures the heartbeat of a dataset, providing a concise representation of its central tendency. It represents the hypothetical “average” value that would result if every data point were added together and divided by the total number of observations. Understanding Mu is crucial, as it unveils the heartbeat of a dataset, revealing its overall shape and distribution.
Mean, Mu, and Their Intimate Dance
While Mu often masquerades as the “mean,” a crucial distinction exists. Mu represents the true average of an entire population, a value that may remain forever hidden from us. In contrast, the “sample mean” (often denoted as x̄) represents our best estimate of Mu, derived from a smaller subset of the population. This sample mean serves as a proxy for Mu, allowing us to make inferences about the larger population from which it was drawn.
A Tale of Three Means: Arithmetic, Weighted, and More
Beyond the familiar arithmetic mean, Mu, the statistical world boasts a tapestry of average measures, each tailored to specific scenarios. Weighted means, for instance, assign different weights to data points, emphasizing their importance in the overall calculation. Geometric means, on the other hand, excel at capturing multiplicative growth or decay patterns, while harmonic means find their niche in scenarios involving rates and proportions.
Unveiling the Significance of Mu in Statistical Analysis
Mu’s significance in statistical analysis extends far beyond mere description. It serves as a lynchpin for hypothesis testing, where we compare sample means to Mu to determine statistical significance. It supports the construction of confidence intervals, pinpointing the range within which we can expect Mu to lie. Further, Mu empowers us to create statistical models, predicting future outcomes based on past trends and patterns.
In the realm of statistics, Mu reigns supreme, acting as an indispensable compass guiding our understanding of data. Whether serving as the heartbeat of central tendency, informing our inferences about population means, or empowering our statistical models, Mu holds the key to unlocking the secrets hidden within data. Embracing its significance is paramount for effective data analysis and informed decision-making.