Mastering Probabilities, Risks, And Rewards: The “What Are The Chances” Game
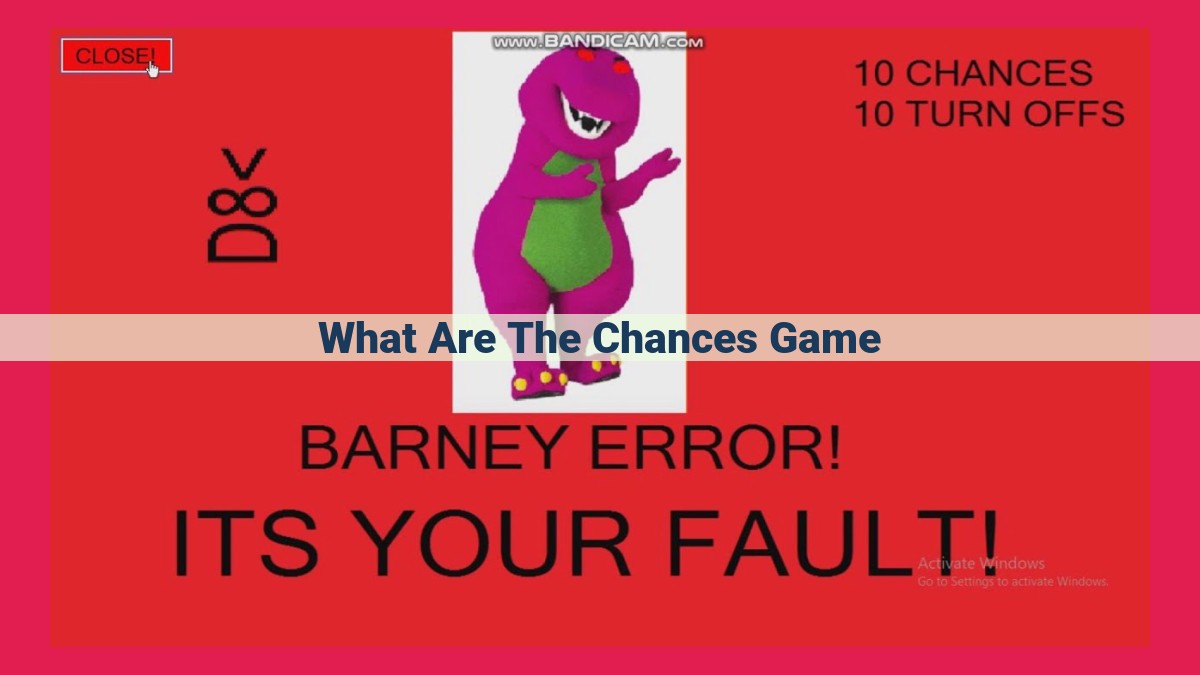
The “what are the chances” game delves into the concepts of probability, risk, reward, and the house edge in games of chance. It explores understanding probability and odds, assessing risk and potential loss, anticipating reward and potential gain, and balancing these factors through expected value. The game also highlights cognitive biases like the gambler’s fallacy and employs statistical methods such as Monte Carlo simulations, the central limit theorem, and standard deviation to predict outcomes and measure variation.
Understanding Probability: The Foundation of Chance
Probability, the cornerstone of chance, is fundamental to our understanding of the world around us. It quantifies the likelihood of events occurring, allowing us to make informed decisions and predictions.
Sample space refers to the set of all possible outcomes in a given situation. Events are subsets of the sample space, representing specific outcomes or combinations of outcomes that we’re interested in. The probability distribution assigns a numerical value to each event, indicating its likelihood of occurrence.
One notable theorem in probability theory is Bayes’ theorem, which provides a way to update our beliefs or probabilities based on new evidence. It’s a powerful tool for refining our understanding of uncertain situations and making more accurate predictions.
By grasping the concepts of sample space, event, and probability distribution, we can build a solid foundation for understanding the intricacies of chance and making informed decisions in the face of uncertainty.
Odds: Quantifying the Realm of Possibility
In the realm of uncertainty, where chance and fortune intertwine, odds emerge as a powerful tool to quantify the likelihood of events. While probability denotes the mathematical chance of an outcome, odds offer a more intuitive and relatable measure.
Just as a coin toss has two equally likely outcomes (heads or tails), the odds of each outcome are 1:1. This means that for every one favorable outcome (e.g., heads), there is one unfavorable outcome (e.g., tails).
However, not all events are so evenly balanced. Let’s consider the probability of rolling a six on a standard die. There is only one favorable outcome (rolling a six) out of a total of six possible outcomes. Therefore, the probability of rolling a six is 1/6.
Converting Probability to Odds
To convert a probability to odds, use the following formula:
Odds = (Probability of Success) / (Probability of Failure)
For the die roll example, the probability of failure (not rolling a six) is 5/6. Thus, the odds of rolling a six are:
Odds = (1/6) / (5/6) = 1:5
This means that for every one successful outcome (rolling a six), there are five unsuccessful outcomes.
Implications of Odds
Understanding odds is crucial for informed decision-making in various scenarios. For instance, in gambling, players can use odds to calculate their chances of winning and the potential payouts. In risk management, odds help assess the likelihood of adverse events and the potential consequences.
By quantifying possibility through odds, we gain a deeper understanding of the uncertain world around us. Whether it’s the toss of a coin, the roll of a die, or the outcome of a sporting event, odds empower us with a practical tool to navigate the realm of chance and make informed choices.
Assessing Potential Loss: Understanding Risk
In the realm of decision-making, risk emerges as a crucial factor that demands our attention. Risk encapsulates the potential for loss or harm associated with a particular action or event. To grasp the nature of risk effectively, it’s imperative to delve into the concepts of probability, severity, and expected loss.
Probability: Simply put, probability signifies the likelihood of an event occurring. It’s expressed as a numerical value between 0 and 1. An event with a probability of 0 is impossible, while a probability of 1 indicates certainty.
Severity: Severity measures the magnitude of the loss or harm that may result from an event. It can be expressed in monetary terms, health consequences, or other quantifiable metrics.
Expected Loss: Expected loss represents the average amount of loss that can be anticipated over a specific period. It’s calculated by multiplying the probability of an event by its potential severity.
The interplay between probability, severity, and expected loss provides valuable insights into potential risks. A higher probability and greater severity result in a higher expected loss, indicating a more significant risk. Conversely, a lower probability or severity reduces the expected loss and, in turn, the level of risk.
Understanding risk is paramount for informed decision-making. By carefully considering the probability, severity, and expected loss, individuals and organizations can make well-calculated choices that minimize potential losses and maximize opportunities.
Reward: Embracing the Promise of Potential Gain
In the realm of decision-making, where chance and strategy intertwine, we often find ourselves weighing the risks against the potential rewards. Reward, in this context, refers to the anticipated positive outcome of an uncertain event. Understanding how to quantify and assess reward is crucial for navigating decision-making under uncertain circumstances.
The Probability Factor:
Just as probability measures the likelihood of an event occurring, it also plays a pivotal role in determining the potential reward. Probability represents the proportion of favorable outcomes to all possible outcomes in a given situation. The higher the probability of an event, the greater the chance of obtaining the desired reward.
The Value of Gain:
Value refers to the desirability or worthiness of a particular outcome. It is often expressed in terms of monetary value, personal satisfaction, or other forms of utility. The greater the value of the reward, the more attractive it becomes.
Expected Gain: Blending Probability and Value:
Expected gain is a mathematical calculation that combines probability and value. It represents the average value of the reward that can be anticipated over multiple repetitions of the uncertain event. The formula for expected gain is:
Expected gain = Probability of event × Value of reward
The higher the expected gain, the more compelling the reward becomes. It provides decision-makers with a quantitative estimate of the potential benefit associated with a particular action or decision.
By understanding the concepts of probability, value, and expected gain, we can make more informed decisions when faced with uncertain outcomes. We can weigh the potential rewards against the risks and make choices that maximize our chances of achieving positive outcomes.
Expected Value: Navigating Risk and Potential Reward
When making decisions involving chance, it’s crucial to understand the concept of expected value. It’s a powerful tool that balances the potential risks and rewards associated with an event, helping you make informed choices. Let’s break it down:
What is Expected Value?
Expected value is the weighted average of all possible outcomes, considering the probability of each outcome. It’s calculated by multiplying each outcome by its probability and then summing up these products. This value provides a measure of the expected result of an event.
Probability, Mean, and Variance
Expected value relies on three key statistical concepts:
- Probability: The likelihood of an event occurring.
- Mean: The average of all possible outcomes.
- Variance: A measure of how spread out the outcomes are.
Balancing Risk and Reward
Expected value helps us compare the risk and reward involved in a decision. A positive expected value indicates that the potential reward outweighs the risk, while a negative expected value suggests that the risk is greater than the potential reward.
By considering the expected value, we can make more rational decisions by choosing options with a higher likelihood of a favorable outcome. However, it’s important to remember that expected value does not guarantee a specific outcome, but rather provides an estimate of the average outcome.
Example:
Imagine a coin toss game where you bet $1 to win $2 if the coin lands on heads and lose $1 if it lands on tails. The probability of heads is 0.5 and tails is also 0.5.
Probability of winning: 0.5
Reward if winning: $2
Probability of losing: 0.5
Loss if losing: $1
Expected value: (0.5 * $2) + (0.5 * -$1) = $0.50
In this scenario, the expected value is positive, indicating that on average, you can expect to win $0.50 per game. However, it’s important to remember that individual outcomes may vary, and you may win or lose more in any given game.
The House Edge: Unveiling the Game’s Advantage
In the realm of casino games, there’s an unspoken truth that every player should be aware of: the house edge. This is the inherent advantage that the game holds over the players, favoring the casino’s odds of winning in the long run. So, what exactly is the house edge, and how does it work?
The Probability Advantage
The house edge stems from the probability of winning. In most casino games, the probabilities are stacked in favor of the house. For example, in a standard game of craps, the probability of rolling a 7 is significantly higher than the probability of rolling any other number. This means that the casino has a higher chance of winning each individual bet.
Expected Return: A Measure of the House Edge
To quantify the house edge, we use a concept called expected return. This is the average amount of money a player can expect to lose over time. It’s calculated by multiplying the probability of winning by the amount won, and the probability of losing by the amount lost. For instance, if a game has a 5% house edge, it means that for every \$100 you wager, you can expect to lose \$5 on average over an extended period.
Consequences for Players
Understanding the house edge is crucial for players. It means that playing casino games on a regular basis is essentially a losing proposition, as the odds are always against you. However, this doesn’t mean that playing for fun is impossible. By choosing games with a lower house edge, setting limits, and managing your bankroll wisely, you can minimize your losses and enjoy gambling responsibly.
The Gambler’s Fallacy: A Cognitive Bias That Can Cost You Dearly
Imagine you’re at a roulette table, watching the ball spin around and around. You’ve seen it land on black five times in a row. The urge to bet on red may be strong, but don’t fall for it. This is the gambler’s fallacy at play, the belief that past outcomes influence future ones.
The truth is, each spin of the roulette wheel is an independent event. The ball has no memory of where it landed previously. The probability of it landing on red or black remains 50%, regardless of what happened in the past.
Yet, we humans have a psychological tendency to see patterns where none exist. We like to find order in chaos. When we see a streak of black or red on the roulette wheel, we start to believe that the other color is “due.” This is called the clustering illusion.
But it’s just an illusion. Random events by their nature have no patterns. They’re like a series of coin flips. Just because you flipped a coin ten times and got heads doesn’t mean the next flip is more likely to be tails. Each flip is a new event, with a 50% chance of either outcome.
Understanding the gambler’s fallacy can save you money. Don’t let your emotions cloud your judgment when gambling. Remember that past outcomes have no bearing on future ones. Stick to the odds and bet wisely.
The Monte Carlo Method: Simulating Uncertainty and Predicting the Future
In the realm of probability and risk analysis, Monte Carlo Method emerges as a powerful tool for grappling with uncertain events and predicting future outcomes. It’s a storytelling approach to understanding random processes, using random numbers as building blocks to create countless possible scenarios.
Imagine a vast room filled with dice, each representing a different aspect of a complex system. By rolling these dice repeatedly, the Monte Carlo Method generates a multitude of possible outcomes, creating a virtual portrait of how the system might behave.
Just like a weather forecast that relies on computer simulations, the Monte Carlo Method enables us to simulate future events and gauge their likelihood. By running countless simulations, we can uncover patterns and trends that would otherwise remain hidden within the sea of possibilities. Through this computational storytelling, we gain invaluable insights into the unpredictable nature of chance.
In essence, the Monte Carlo Method transforms uncertainty into a tangible narrative, empowering us to make better decisions even when the future is shrouded in mystery. Whether predicting market fluctuations or forecasting the behavior of complex ecosystems, this method unravels the secrets of uncertainty, allowing us to navigate the unknown with increased confidence.
The Central Limit Theorem: Unraveling the Mystery of Sample Means
In the realm of probability, the Central Limit Theorem emerges as a beacon of hope, guiding us toward predicting the behavior of sample means. It unravels the fascinating truth that regardless of the underlying probability distribution of a population, the distribution of sample means converges toward a bell-shaped normal distribution as the sample size increases.
Consider a vast population with an unknown probability distribution: the average value (mean) of this population remains shrouded in mystery. Fortunately, the Central Limit Theorem empowers us to estimate this elusive mean by collecting multiple samples from the population. Astonishingly, the distribution of these sample means, regardless of the original population’s shape, approaches a normal distribution.
This phenomenon arises from the fundamental property of sample means. By definition, a sample mean is the sum of individual observations divided by the sample size. As we increase the sample size, the random fluctuations in individual observations tend to cancel each other out, resulting in a more stable and predictable distribution.
The Central Limit Theorem holds immense significance in statistical inference. It allows us to make inferences about the population mean based on sample data. By calculating the mean and standard deviation of the sample means, we can determine the confidence interval within which the true population mean is likely to lie.
This remarkable theorem finds widespread application in various fields, including quality control, medical research, and financial forecasting. It provides a powerful tool for researchers and analysts to draw meaningful conclusions from sample data, even when the underlying population characteristics remain unknown.
Standard Deviation: Quantifying Variation
When it comes to understanding chance and probability, measures like variance and standard deviation become vital tools in our arsenal. These statistical concepts provide a quantitative measure of how far spread out or variable a set of outcomes is.
Variance calculates the average of the squared differences between each data point and the mean of the dataset. This measure indicates the variability of the observations within the group.
The standard deviation, on the other hand, is the square root of the variance. It provides a more convenient unit of measurement for variability, as it is expressed in the same unit as the original data.
High values of standard deviation indicate that the data is widely dispersed around the mean, while low values suggest that the data is closely clustered around the mean. By calculating the standard deviation, we can quantify the spread of outcomes and assess whether there is significant variation within a dataset.
For instance, in a study of basketball players’ heights, a standard deviation of 3 inches would imply that most players are within 3 inches of the average height. Conversely, a standard deviation of 6 inches would indicate a wider range of heights, with many players significantly taller or shorter than the average.
Understanding standard deviation and variance is crucial for analyzing data and making inferences about populations. They provide insights into the dispersion of values and help us draw meaningful conclusions from our observations.