Master Cases In Statistics: Unlocking The Foundation Of Data Analysis
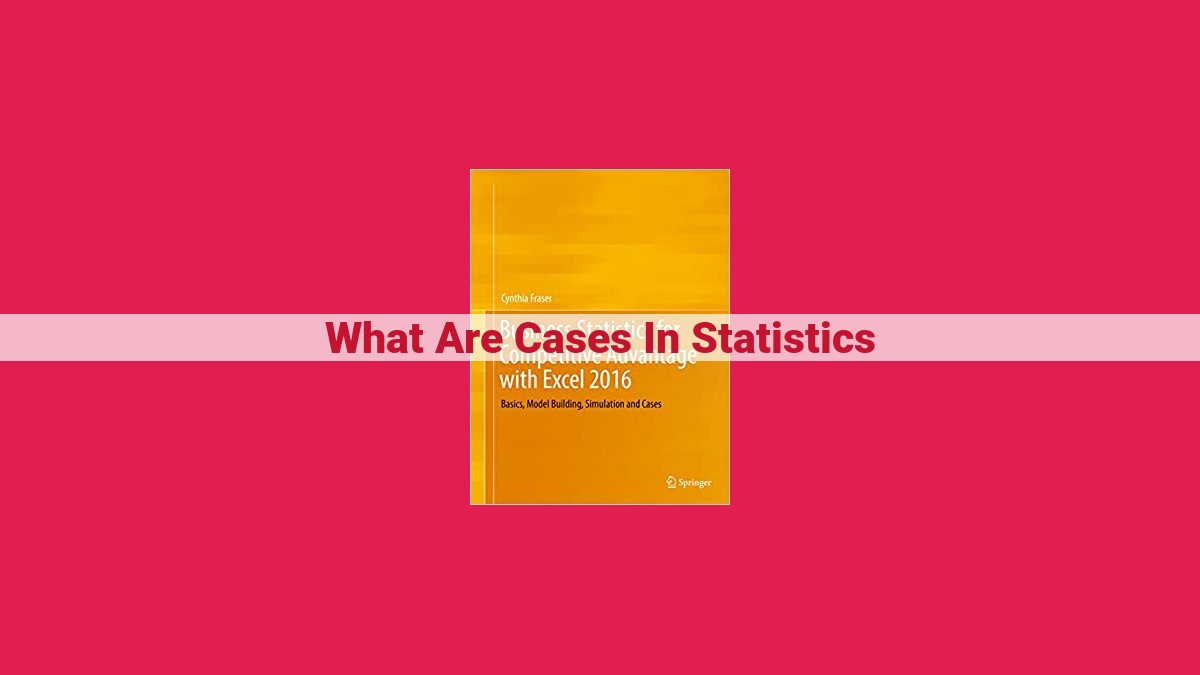
In statistics, cases represent individual data points that make up a dataset. They are the fundamental building blocks of statistical analysis, serving as the observations or subjects under investigation. Cases can be distinguished from populations, which refer to the entire group of individuals or objects being studied, and samples, which are subsets of populations selected for analysis. Understanding the concept of cases is crucial for accurately analyzing data, as their characteristics and types influence the choice of appropriate statistical techniques and the validity of conclusions drawn from the analysis.
Understanding Cases in Statistics: A Storytelling Guide
In the realm of statistics, data holds immense power. To uncover meaningful insights from raw numbers, we must first understand the building blocks of any statistical analysis: cases.
Cases are individual observations or measurements that make up a dataset. They represent the units of analysis, whether they be people, objects, events, or any other entity of interest. Understanding these cases is crucial for accurate data analysis and interpretation.
The Role of Cases in Statistical Analysis
Cases form the foundation of statistical inferences. They provide the raw material for researchers to draw conclusions about populations, the broader group from which the cases are drawn. By examining the characteristics of individual cases, statisticians can make generalizations about the entire population.
However, it’s important to note the distinction between cases, populations, and samples. A population is the complete set of all possible cases, while a sample is a smaller subset of cases selected to represent the population. Researchers often use samples to draw inferences about larger populations, making it essential to ensure that the sample is representative of the population.
Quantitative vs. Qualitative Variables
Cases can be described using variables, which are characteristics or traits that can vary from case to case. Variables can be either quantitative or qualitative:
-
Quantitative variables are numerical and can be measured on a continuous or discrete scale. Continuous variables can take any value within a given range (e.g., height), while discrete variables can only take specific values (e.g., number of children).
-
Qualitative variables are categorical and describe non-numerical attributes of cases. They can be nominal (e.g., gender) or ordinal (e.g., education level), indicating distinct categories with no inherent numerical value.
Understanding the type of variable associated with each case is essential for choosing appropriate statistical techniques and ensuring accurate data analysis.
Types of Variables in Statistics: Demystifying Categorical and Numerical Data
In the realm of statistics, understanding the nature of data is crucial for meaningful analysis. Data can be classified into two broad categories based on its characteristics: categorical and numerical variables. Grasping the distinction between these variable types empowers researchers to choose appropriate statistical techniques and draw valid conclusions.
Categorical Variables: Describing Attributes
Categorical variables, also known as qualitative variables, capture non-numerical attributes or characteristics. They represent categories or groups into which data can be divided. Categorical variables are further classified into two subcategories:
-
Nominal Variables: These variables represent distinct categories without any inherent order or hierarchy. For instance, gender (male, female) or blood type (A, B, AB, O) are nominal variables.
-
Ordinal Variables: While ordinal variables still represent categories, they possess a natural ordering or ranking. For example, a Likert scale (strongly agree, agree, neutral, disagree, strongly disagree) is an ordinal variable used to measure attitudes or opinions.
Numerical Variables: Quantifying Observations
Numerical variables, also known as quantitative variables, measure numerical values or amounts. They can be further divided into two types:
-
Continuous Variables: These variables can take on any value within a specified range. They represent a continuous spectrum of values, such as height, weight, or temperature.
-
Discrete Variables: Discrete variables can only take on specific, distinct values. They represent countable quantities, such as the number of children in a family or the number of errors in a text.
Importance of Variable Type Distinction
Understanding the type of variable at hand is essential for choosing appropriate statistical methods. For example, statistical tests used to compare categorical variables (e.g., chi-square test) differ from those used to analyze numerical variables (e.g., t-test or ANOVA).
Furthermore, the level of measurement of variables influences the types of summary statistics and visualizations that can be used. For instance, categorical variables are often presented using frequency counts and bar charts, while numerical variables can be summarized using measures of central tendency (mean, median) and dispersion (standard deviation, range).
By mastering the distinction between categorical and numerical variables, researchers can navigate the world of statistics with confidence. Understanding these concepts empowers them to choose the right analytical tools, extract meaningful insights from data, and communicate their findings effectively.
Quantitative vs. Qualitative Variables: A Tale of Measurement and Analysis
In the realm of statistical storytelling, variables play a pivotal role, painting vivid pictures of the data we encounter. Like the characters in a novel, each variable possesses its own unique traits, shaping the narrative of our analysis. Among these traits, the level of measurement is paramount, differentiating variables into two distinct categories: quantitative and qualitative.
Quantitative Variables: Measuring with Numbers
Quantitative variables, like the pages of a novel, unfold with numerical values. They reside on a continuous scale, meaning they can take on any value within a specific range. Think of the weight of a book or the temperature on a summer day. These variables allow us to measure degrees of intensity, revealing patterns and trends that might otherwise remain hidden.
Qualitative Variables: Categorizing and Classifying
Qualitative variables, on the other hand, delve into the realm of categories. They assign non-numerical labels to data, capturing qualities rather than quantities. Imagine the genre of a book or the color of a flower. These variables classify items into distinct groups, providing a deeper understanding of the types of data we’re dealing with.
Measurement Levels and Analysis Techniques
The level of measurement profoundly influences the data analysis techniques we employ. For quantitative variables, we can perform arithmetic operations and calculate measures of central tendency like mean and median. Qualitative variables, however, require non-parametric tests that don’t assume an underlying distribution.
By comprehending the distinction between quantitative and qualitative variables, we gain a deeper appreciation for the data we work with. We unlock the power to choose appropriate analysis methods, extracting meaningful insights from the stories our data tells.
Understanding Subtypes of Variables
When working with data, it’s crucial to understand the different types of variables involved. Categorical variables (also known as qualitative variables) represent qualities or attributes that can be placed into categories. These variables don’t have numerical values associated with them.
There are two main types of categorical variables:
- Nominal variables: These categories have no inherent order or ranking. For example, eye color (blue, brown, green, etc.) or job title (teacher, doctor, lawyer, etc.).
- Ordinal variables: These categories have an order or ranking. For instance, education level (high school, bachelor’s degree, master’s degree, etc.) or customer satisfaction ratings (very unsatisfied, slightly unsatisfied, satisfied, very satisfied).
Numerical variables (also known as quantitative variables), on the other hand, represent quantities that can be measured and expressed as numbers. Numerical variables are subdivided into two primary types:
- Continuous variables: These variables can take on any value within a given range. Examples include height, weight, and age.
- Discrete variables: These variables can only take on distinct, whole-number values. Examples include the number of siblings or the number of cars owned.
Understanding the type of variable you’re dealing with is essential for choosing appropriate statistical techniques and making accurate interpretations. Continuous variables allow for more precise statistical analysis, while discrete variables are often used for counting or categorizing data. By recognizing the unique characteristics of each variable type, you can effectively analyze and interpret your data to gain valuable insights.
Example Applications
- Real-world examples of how cases, variables, and their types are used in data analysis
- Illustration of the practical importance of understanding these concepts
Example Applications of Cases and Variables in Data Analysis
In the realm of data analysis, understanding the concept of cases and variables is crucial for meaningful and effective insights. Cases represent individual subjects or observations in a dataset, while variables are the characteristics or attributes of those cases.
Let’s illustrate this with a real-world example:
Imagine a study conducted to analyze the factors influencing the health of patients at a hospital. Each patient is considered a case, and their information, such as age, gender, medical history, and test results, constitutes their variables. By examining the variables within each case, researchers can identify patterns and relationships that provide valuable insights into the factors contributing to patients’ health outcomes.
Another application lies in market research. Suppose a company surveys consumers to understand their preferences for a new product. Each customer who completes the survey becomes a case. Variables such as age, income, location, and brand loyalty help the company segment consumers into distinct groups, allowing them to tailor their marketing strategies accordingly.
Understanding the types of variables is equally important. Categorical (qualitative) variables classify cases into groups or categories, such as gender (male/female) or product preference (A/B/C). Numerical (quantitative) variables represent numerical values, like age or income.
The level of measurement of variables dictates the appropriate statistical techniques for analysis. Continuous variables allow for any value within a given range, while discrete variables can only take specific values, like integers. For instance, age can be considered a continuous variable, while the number of children in a household is discrete.
By understanding the interplay between cases and variables, data analysts can uncover valuable insights that drive decision-making and optimize outcomes. From healthcare to marketing and beyond, the practical importance of these concepts in data analysis cannot be overstated.