Understanding Lurking, Confounding, And Effect Modifier Variables For Accurate Statistical Analysis
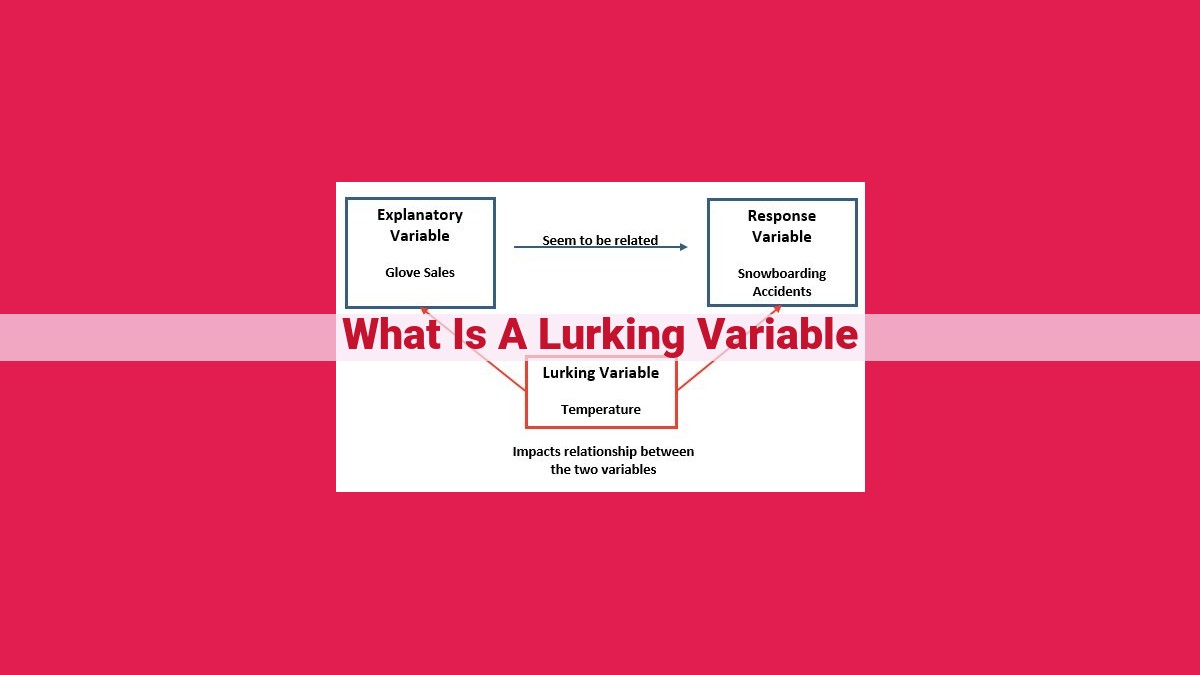
Lurking variables, unaccounted for in statistical analysis, can bias results. Confounding variables, affecting exposure and outcome, can distort conclusions. Effect modifiers alter the strength or direction of relationships. Recognition of these variables is crucial for accurate interpretation.
Lurking Variables: Unveiling the Hidden Influences on Statistical Analysis
When it comes to statistical analysis, it’s easy to assume that the variables we measure are the only ones shaping the results. However, there’s often an unseen force lurking in the background, influencing the relationships we observe: the lurking variable.
Defining the Lurking Variable
A lurking variable is an unaccounted-for variable that exerts an influence on the observed relationship between two other variables. It’s like an unseen puppeteer, pulling the strings behind the scenes and distorting the results.
Lurking variables can lead to misinterpretation, bias, and confounding. Misinterpretation occurs when we mistake the relationship between the lurking variable and the dependent variable as the relationship between the independent and dependent variables. Bias arises when the lurking variable is unevenly distributed across different groups, skewing the results. Confounding occurs when the lurking variable affects both the independent and dependent variables, making it difficult to determine which variable is truly causing the observed effect.
Exploring the Impact
Lurking variables can have a profound impact on statistical analysis. For example, in a study examining the relationship between coffee consumption and heart disease, the lurking variable of age could confound the results. Older adults are more likely to both drink coffee and have heart disease, making it difficult to determine if coffee consumption is truly the culprit.
Another example is in studies linking smoking to lung cancer. The lurking variable of education level could confound the results, as smokers are more likely to have lower education levels, which is also associated with an increased risk of lung cancer.
Avoiding the Pitfalls
Recognizing the presence of lurking variables is crucial for reliable statistical analysis. Researchers must carefully consider all potential lurking variables and take steps to control for their influence.
One strategy is to stratify the data based on the lurking variable, analyzing the relationship between the independent and dependent variables separately for each subgroup. Another method is to use multivariable models, which allow researchers to adjust for the effects of multiple lurking variables simultaneously.
Lurking variables are an inherent challenge in statistical analysis. By recognizing their potential influence and taking steps to control for their effects, researchers can uncover the true relationships between variables and draw meaningful conclusions. Embracing the concept of lurking variables empowers us to unravel the hidden forces shaping our statistical findings and make more informed decisions.
Exploring the Impact of Effect Modifiers in Statistical Analysis
Unveiling the Hidden Forces Shaping Relationships
Lurking variables, like mischievous spirits, can haunt our statistical analyses, twisting the truth before our very eyes. Among these lurkers, effect modifiers stand out as enigmatic puppeteers, subtly altering the dynamics of exposure-outcome relationships.
What are Effect Modifiers?
Imagine a study exploring the relationship between caffeine intake and headache frequency. You might expect a straightforward pattern: the more caffeine consumed, the fewer headaches. But what if a hidden factor, such as gender, intervened? In this scenario, gender acts as an effect modifier, changing the magnitude or direction of the caffeine-headache link.
The Gender Enigma
For instance, the study might reveal that women experience a more pronounced decrease in headaches with increased caffeine intake compared to men. This finding unveils a previously hidden layer of complexity, suggesting that gender modifies the strength of the caffeine-headache association.
Beyond Gender: Other Modifying Forces
Effect modifiers can emerge from diverse sources. Consider age in a study on the association between smoking and lung cancer. Older individuals may exhibit a stronger link between smoking and lung cancer than younger people, highlighting age as a potential effect modifier.
Implications for Statistical Analysis
The presence of effect modifiers poses a challenge for researchers. Ignoring them can lead to misleading conclusions and inflated effect sizes. To mitigate these risks, statisticians employ stratified analysis, dividing the sample into subgroups based on the effect modifier. This allows them to assess the relationship between exposure and outcome within each subgroup, revealing the true nature of the associations.
Effect modifiers are like hidden players in a statistical game, subtly shaping the outcome. Recognizing their influence is crucial for obtaining accurate and meaningful results. By embracing stratified analysis and acknowledging the potential for effect modification, we can unveil the true nature of exposure-outcome relationships and avoid the pitfalls of lurking variables.
Lurking Variables: Their Impact on Statistical Analysis
In the realm of statistical analysis, lurking variables are like hidden puppet masters, manipulating results without being seen. These unaccounted-for variables can introduce bias and confound our understanding of data. By recognizing and controlling for lurking variables, we can uncover the true relationships between variables.
Confounding Variables: The Unseen Mediator
Confounding variables are sneaky characters that influence both the exposure and outcome variables. They create a false association between the two, making it seem like one causes the other.
Consider the classic example of lung cancer and smoking. Age is a confounding variable in this scenario. Older people are more likely to smoke and also more likely to develop lung cancer. If we don’t account for age, we might mistakenly conclude that smoking causes lung cancer, when in reality, it’s simply that older people are more susceptible to both.
Effect Modifiers: The Variable That Changes the Game
Effect modifiers are like the secret ingredients that alter the strength or direction of an exposure-outcome relationship. They highlight factors that can make a big difference in how variables interact.
Let’s say we’re looking at headache frequency and caffeine intake. Gender is an effect modifier in this case. Women tend to be more sensitive to caffeine, meaning they may experience more headaches with lower caffeine intake compared to men. Ignoring gender as an effect modifier could lead us to underestimate the impact of caffeine on headache frequency in women.
Case Study: Lurking Variables, Confounding, and Effect Modification in Action
In a study on caffeine intake and headache frequency, researchers discovered that:
- Lurking variable: Sleep quality – People who slept poorly had more headaches, regardless of caffeine intake.
- Confounding variable: Age – Older people reported more headaches, which could be due to other age-related factors.
- Effect modifier: Caffeine sensitivity – Those with caffeine sensitivity experienced more headaches with lower caffeine intake.
This case study illustrates the importance of considering all relevant factors in statistical analysis. By controlling for confounding variables and accounting for effect modifiers, we can better understand the associations between variables and draw more accurate conclusions.
Recognizing lurking variables is crucial for reliable statistical analysis. By identifying and addressing these hidden influencers, we can:
- Uncover the true relationships between variables
- Avoid misleading conclusions and biased interpretations
- Ensure the accuracy and validity of our research findings
Careful study design and thorough data interpretation are essential to minimize the impact of lurking variables. By embracing this awareness, we can make more informed decisions based on reliable statistical evidence.