Hypothesis Testing: A Comprehensive Guide To Statistical Significance And Hypothesis Comparison
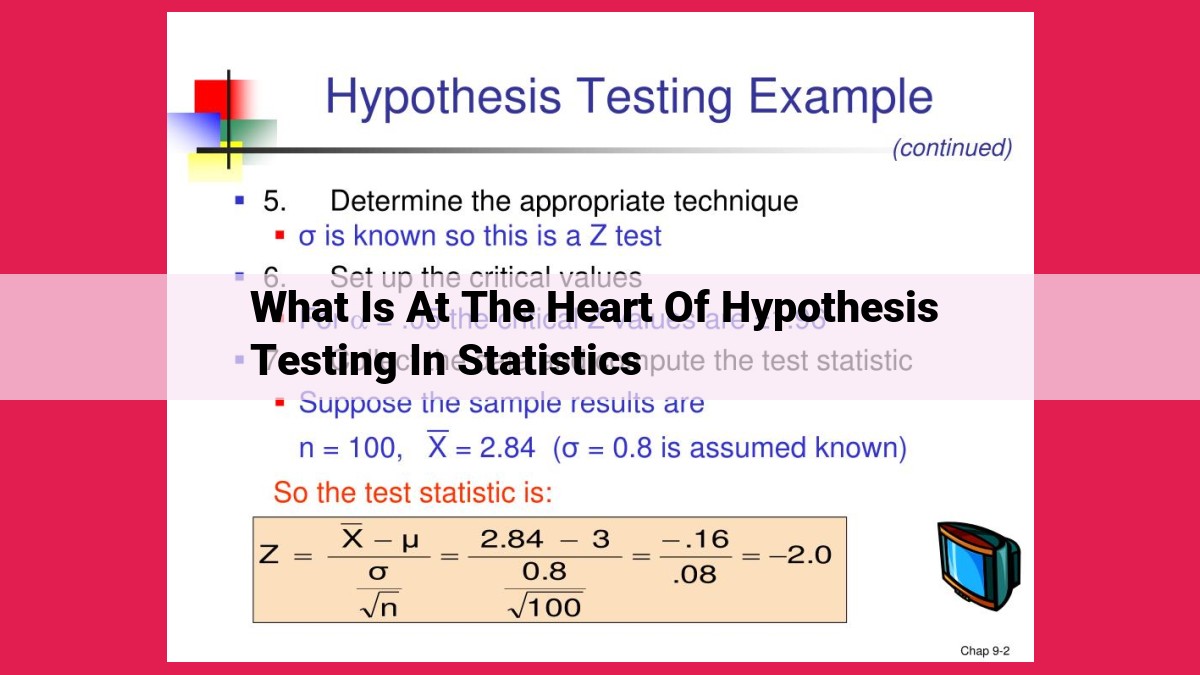
At the core of hypothesis testing lies the fundamental concept of comparing two statistical hypotheses: the null hypothesis (H0), which represents the initial assumption, and the alternative hypothesis (Ha), which proposes an opposing claim. Through the calculation of a p-value, hypothesis testing determines the probability of obtaining observed results under the null hypothesis. By comparing the p-value to a pre-established significance level, researchers can evaluate the credibility of the alternative hypothesis and make informed decisions about rejecting or failing to reject H0. Balancing the risk of Type I (false positive) and Type II (false negative) errors is crucial to ensure the reliability of conclusions drawn from statistical data.
In the realm of statistical research, hypothesis testing stands as a powerful tool, a beacon of clarity amidst the sea of uncertainty. It’s the process of making an informed guess (hypothesis) about a population parameter and then testing that guess against evidence.
Hypothesis testing is not merely a mathematical exercise; it’s a fundamental step in any scientific investigation. By systematically evaluating our hypotheses, we can uncover the hidden truths within data, drawing reliable conclusions that advance our understanding of the world.
Consider this: You’re a researcher who wants to determine if a new advertising campaign has increased sales. You form a hypothesis: The advertising campaign has increased sales by 10% and proceed to collect data. Hypothesis testing will then guide you in analyzing the data and determining whether the evidence supports your hypothesis.
By embracing hypothesis testing, we elevate the quality of our research, minimizing the risk of making erroneous conclusions. It’s the scientific compass that keeps us on course, ensuring that our findings are grounded in data-driven insights.
Fundamental Concepts of Hypothesis Testing
- A. Null Hypothesis (H0)
- Understanding the default assumption about a population parameter
- B. Alternative Hypothesis (Ha)
- Stating the opposing claim to the null hypothesis
- C. Statistical Significance
- Identifying the threshold for accepting the alternative hypothesis (determining the credibility of the alternative hypothesis)
- D. P-value
- Calculating the probability of obtaining observed results under the null hypothesis
- E. Type I Error
- Avoiding the risk of rejecting a true null hypothesis (false positive)
- F. Type II Error
- Minimizing the possibility of failing to reject a false null hypothesis (false negative)
Fundamental Concepts of Hypothesis Testing
In hypothesis testing, we investigate claims about the world by contrasting two opposing ideas, known as the null hypothesis (H0) and the alternative hypothesis (Ha).
The null hypothesis represents the default assumption about some population parameter. It often states that there is no significant difference or effect. For instance, if we’re testing the effectiveness of a new drug, the null hypothesis implies that the drug has no impact on patients.
Conversely, the alternative hypothesis poses the opposing claim to the null. In our drug example, the alternative hypothesis might suggest that the new drug does improve patient outcomes.
To evaluate the credibility of the alternative hypothesis, we establish a threshold for acceptance, known as statistical significance. This threshold is typically set at a p-value of 0.05, meaning that the probability of obtaining the observed results under the null hypothesis must be less than 5% to reject it.
The p-value is calculated based on the observed data. It reflects the likelihood that the observed difference or effect occurred by chance alone. A low p-value indicates that the null hypothesis is unlikely to be true, lending support to the alternative hypothesis.
Minimizing Errors
Hypothesis testing involves two types of potential errors:
-
Type I error (false positive): Rejecting a true null hypothesis. This occurs when we incorrectly conclude that there is a significant difference or effect when, in reality, there isn’t.
-
Type II error (false negative): Failing to reject a false null hypothesis. This happens when we conclude that there is no significant difference or effect when there actually is.
Balancing the risk of making these errors is crucial. A lower significance level reduces the risk of a Type I error but increases the risk of a Type II error, and vice versa. Researchers must carefully consider the consequences of each type of error in their specific research context.
Balancing Type I and Type II Errors
In the world of statistical inference, we often find ourselves balancing the risks associated with two types of errors: Type I errors (false positives) and Type II errors (false negatives). These errors stem from the delicate dance between statistical significance and hypothesis testing.
Let’s imagine a researcher who wants to test whether a new drug is effective. They start with the null hypothesis (H0) that the drug has no effect, which serves as a default assumption. To challenge this assumption, they formulate an alternative hypothesis (Ha) that the drug does have an effect.
The statistical significance level, often represented by p, is the threshold we set for rejecting the null hypothesis. If the p-value (the probability of our observed results assuming the null hypothesis is true) falls below the significance level, we reject the null hypothesis and accept the alternative hypothesis.
However, this decision-making process is not without its risks. A Type I error occurs when we falsely reject the true null hypothesis. This error is akin to unjustly accusing an innocent person of a crime. To minimize the chances of a Type I error, we set a strict significance level, typically 0.05 or 0.01.
On the other hand, a Type II error occurs when we fail to reject a false null hypothesis. This error is like letting a guilty individual go free. To reduce the risk of a Type II error, we increase the significance level or increase the sample size.
The balance between these error rates is crucial. A lower significance level reduces the risk of a Type I error but increases the risk of a Type II error. Conversely, a higher significance level lowers the risk of a Type II error but increases the risk of a Type I error.
Researchers must carefully consider the consequences of each type of error when setting their significance level. For example, in medical research, a Type I error could lead to unnecessary treatments, while a Type II error could prevent patients from receiving effective interventions.
By understanding the trade-offs between Type I and Type II errors, researchers can make informed decisions that balance the risks and enhance the credibility of their statistical conclusions.