Height: A Comprehensive Guide To Measurement Level And Statistical Analysis
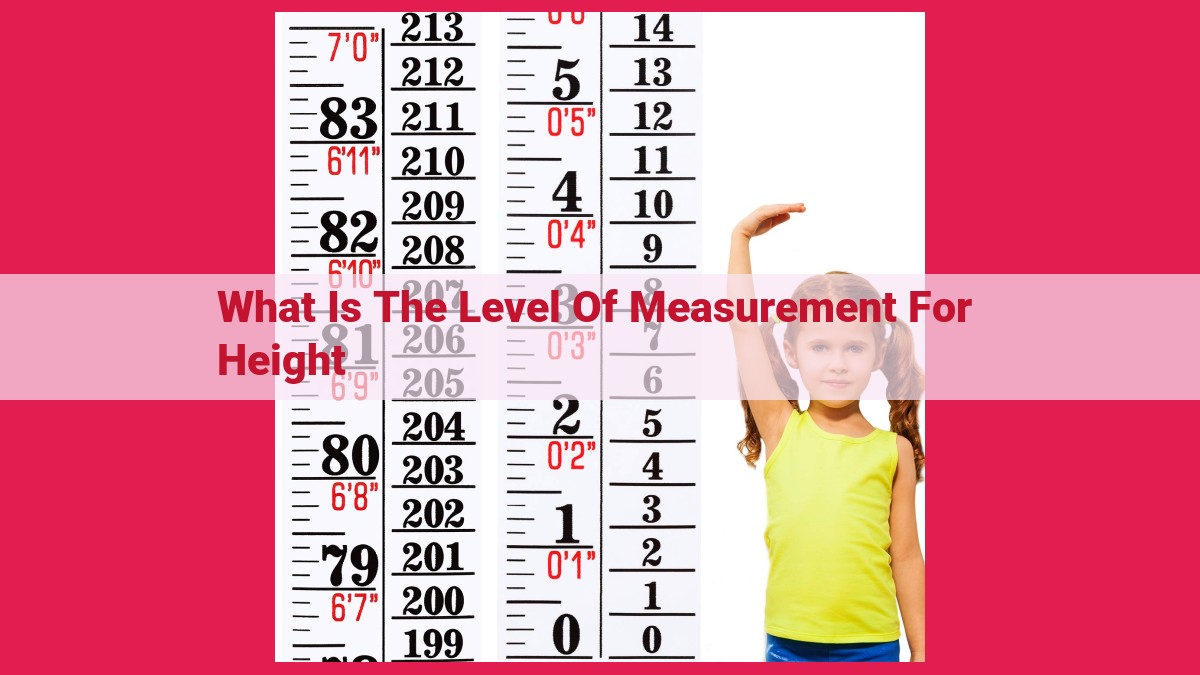
Height, a quantitative variable measuring vertical distance, is a ratio variable with a true zero point and the ability for absolute comparisons. This means that differences in height have meaningful interpretations, and ratios between heights can be validly calculated. Ratio variables hold the highest level of measurement, allowing for the most sophisticated statistical analyses. Understanding the level of measurement for height is crucial for choosing appropriate statistical methods and interpreting results accurately.
Unveiling the Secrets of Height: A Tale of Measurement and Meaning
In the realm of data and measurement, the concept of levels of measurement plays a pivotal role in shaping our understanding and analysis of the world around us. Height, a common and seemingly straightforward metric, holds a fascinating story when examined through this lens.
What Exactly is Height?
Height stands as a quantitative variable that measures the vertical distance from a designated point to the top of an object or person. In our everyday lives, we commonly measure height using units such as inches, centimeters, or meters. These units serve as standardized markers, enabling us to quantify and compare heights across individuals and objects.
The Levels of Measurement Ladder
The world of measurement is often categorized into four distinct levels of measurement:
1. Nominal Level: This level represents the simplest form of measurement, where data points are classified into distinct categories with no inherent order. For instance, gender (male or female) or race (Caucasian, Hispanic, African American) are nominal variables.
2. Ordinal Level: Ordinal variables possess categories that have a definite order, but the differences between the categories are not uniform. An example is socioeconomic status (low, medium, high) or educational attainment (high school diploma, bachelor’s degree, master’s degree).
3. Interval Level: Interval variables are characterized by equal intervals between data points. However, unlike ordinal variables, interval variables lack a true zero point. Examples include temperature (in degrees Celsius or Fahrenheit) or the number of days in a month.
4. Ratio Level: Ratio variables represent the highest level of measurement. They not only have equal intervals but also possess a true zero point. This means that a value of zero truly represents the absence of the measured quantity. Examples include height, weight, and money.
Height: A Ratio Variable
Based on the characteristics of the different levels of measurement, we can conclude that height is indeed a ratio variable. It has a true zero point (zero height represents the absence of height) and its intervals are equal (each unit of height represents the same vertical distance).
This understanding has significant implications for how we analyze and interpret height data. We can not only compare heights in terms of relative differences (e.g., one person is taller than another) but also in terms of absolute values (e.g., one person’s height is twice that of another).
The level of measurement for a variable, such as height, is crucial for determining the appropriate analytical and statistical techniques to use. By understanding the nuances of measurement levels, we can unlock the full potential of data and uncover meaningful insights from the world around us.
Demystifying Levels of Measurement: A Guide to Understanding Height
When it comes to measuring things, we have different ways of doing it. Some measurements tell us just what they are (like the name of a color), while others tell us about how things compare (like how tall or heavy someone is). These different ways of measuring are called levels of measurement.
There are four main levels of measurement:
- Nominal
- Ordinal
- Interval
- Ratio
Nominal level is the most basic level of measurement. It simply categorizes things into different groups, without any inherent order. For example, gender (male or female) is a nominal variable.
Ordinal level is a step up from nominal. It ranks things in a specific order, but the differences between the ranks may not be equal. For instance, socioeconomic status (low, medium, high) is an ordinal variable.
Interval level measurements have equal intervals between them, but they don’t have a true zero point. An example of an interval variable is temperature in Celsius or Fahrenheit.
Ratio level measurements have both equal intervals and a true zero point. This means that you can make meaningful comparisons between different values. Height and weight are classic examples of ratio variables.
Height: A Ratio Variable
So, where does height fit into all of this? Well, height is a ratio variable because it has a true zero point (no negative heights) and equal intervals between measurements. This means that you can meaningfully compare the height of two people, such as saying that one person is twice as tall as the other.
Understanding levels of measurement is crucial for choosing the appropriate statistical analyses for your data. By following these levels, you can ensure that you’re using the right tools to draw accurate conclusions from your research.
Nominal Level: Categories with No Inherent Order
In the realm of data analysis, levels of measurement classify variables based on the type of information they convey. The nominal level, the most basic level, represents variables where data items fall into distinct categories with no inherent order.
Imagine you’re gathering data on the gender of students in a class. Each student belongs to either the male or female category. These categories have no intrinsic order, meaning it doesn’t make sense to say that being male is “greater than” or “less than” being female.
Another example of a nominal variable is race. Individuals belong to different racial categories (e.g., White, Black, Asian), but there’s no logical order among these categories.
Dummy Variables and Factor Variables
In statistical analysis, nominal variables are often represented using dummy variables or factor variables. Dummy variables are binary variables that take the value 1 if the observation belongs to a particular category and 0 otherwise. For example, in the gender example, you could create a dummy variable called “Male” that takes the value 1 for male students and 0 for female students.
Factor variables are a generalization of dummy variables, allowing for more than two categories. Using our gender example, you could create a factor variable with two levels: “Male” and “Female”. This variable would provide more information than a single dummy variable.
Key Takeaway
Nominal variables represent data that falls into distinct categories with no inherent order. They’re commonly used to classify individuals or groups into different characteristics, such as gender, race, or eye color. These variables are often represented using dummy or factor variables in statistical analysis.
Ordinal Level: Unveiling the Hierarchy in Ordered Categories
As we journey through the realm of measurement, we encounter the ordinal level, a fascinating world where categories dance in an inherent order. Unlike nominal variables, where categories stand side by side with no inherent hierarchy, ordinal variables embrace a sense of “greater than” or “less than.”
Picture socioeconomic status (SES), the social ladder we often climb or descend. SES divides people into distinct categories, such as “lower class,” “middle class,” and “upper class.” While these categories are distinct, they’re arranged in an obvious hierarchy, with each step up or down the ladder representing a higher or lower status.
This ordered nature of ordinal variables opens up a new world of statistical possibilities. We can now explore non-parametric tests, statistical tools designed to analyze ordinal data without assuming normality or equal intervals. Rank correlation, a measure of the relationship between two ordinal variables, tells us how strongly the ranks of one variable are associated with the ranks of the other. Median, the middle value of an ordered dataset, provides a meaningful measure of central tendency for ordinal data.
In the realm of height measurement, ordinal levels play a crucial role. Imagine a group of people standing in a line, from shortest to tallest. While we can’t measure their height in precise units, we can assign them to ordinal categories, such as “short,” “medium,” and “tall.” This allows us to compare their heights and make statements like, “John is taller than Mary.”
Understanding the ordinal level of measurement empowers us to analyze data with more nuance and accuracy. It allows us to unearth patterns and relationships that would otherwise remain hidden. By harnessing the power of ordinal variables, we can gain a deeper understanding of the world around us.
Interval Level: Bridging the Gap Between Ordinal and Ratio Scales
In our exploration of levels of measurement, we now encounter the interval level. This scale occupies a middle ground between the ordinal and ratio levels, offering more precision than the former while lacking the true zero point of the latter.
Interval variables possess equal intervals or uniform distances between their values, much like a thermometer measuring temperature. For instance, the difference between 50 degrees Fahrenheit and 60 degrees Fahrenheit is the same as the difference between 70 degrees Fahrenheit and 80 degrees Fahrenheit. This uniformity of intervals allows us to conduct more sophisticated statistical analyses.
One key concept associated with interval variables is central tendency. This refers to the average or middle point of a data distribution. Common measures of central tendency for interval variables include the mean (average) and median (middle value). The standard deviation, a measure of data spread, is also applicable to interval variables.
Furthermore, interval scales enable the use of parametric tests in statistical analysis. Parametric tests rely on the assumption that data is normally distributed and follows certain statistical distributions. These tests provide more powerful statistical inferences than non-parametric tests, which are used for ordinal data.
Examples of interval variables include temperature, time intervals (e.g., days, months), IQ scores, and standardized test scores. While these variables have equal intervals, they lack a true zero point. For example, 0 degrees Fahrenheit does not represent the absence of temperature, and an IQ score of 0 does not indicate a lack of intelligence.
Understanding the level of measurement for a particular variable is crucial for appropriate statistical analysis. Interval variables provide a more precise representation of data compared to ordinal variables, allowing for more robust statistical methods and meaningful comparisons.
Ratio Level: True Zero Point and Absolute Comparisons
When it comes to levels of measurement, ratio variables stand out as the most robust and informative. They possess two defining characteristics that set them apart from other levels: a true zero point and the ability to make absolute comparisons.
A true zero point means that the absence of a quantity is meaningful. For instance, when we measure height, a value of zero indicates that there is no height, a clear and absolute state. This differs from ordinal variables, where the intervals between categories are equal but the zero point is arbitrary or hypothetical.
The ability to make absolute comparisons is another crucial feature of ratio variables. Because they have a true zero point, we can not only rank and order the values but also make meaningful comparisons in terms of magnitude. For example, if Person A is 5 feet tall and Person B is 10 feet tall, we can confidently say that Person B is exactly twice as tall as Person A.
This absolute scale allows us to perform powerful statistical analyses such as calculating means, standard deviations, and conducting parametric tests. These tests are more sensitive and informative than non-parametric tests used with ordinal and nominal variables.
Examples of ratio variables abound in everyday life. Height, weight, money, area, and temperature are all common ratio variables. They provide us with precise and meaningful information about the quantities they measure, allowing for accurate comparisons and in-depth statistical analysis.
In conclusion, ratio variables offer the highest level of measurement precision because they possess a true zero point and enable absolute comparisons. This makes them particularly valuable for scientific research, data analysis, and decision-making where accurate and meaningful measurements are essential.
Understanding Height: A Quantitative Measure
In the realm of measurements, height stands tall as a quantitative variable, capturing the vertical distance from head to toe. Expressed in common units like inches, centimeters, or meters, height provides valuable insights into an individual’s physical attributes.
Levels of Measurement: A Framework for Variables
To categorize quantitative variables like height, we employ the concept of levels of measurement. These levels, ranging from nominal to ratio, define the properties and capabilities of the data.
Nominal Level: Categorical with No Order
At the most basic level, nominal variables represent categories without inherent order or numerical values. Examples include gender (male or female) or race (Asian, African American, Caucasian).
Ordinal Level: Ordered Categories
Ordinal variables, like socioeconomic status or educational attainment, possess categories with an inherent order. While respondents can be ranked or compared within these categories, the actual distance between them is not quantifiable.
Interval Level: Equal Intervals, No True Zero
Interval variables, such as temperature or dates, have equal intervals between values but lack a true zero point. These variables allow for comparisons of differences and the calculation of measures like mean and standard deviation.
Ratio Level: True Zero, Absolute Comparisons
At the highest level, ratio variables like height, weight, and money possess a true zero point and equally spaced intervals. This unique characteristic enables absolute comparisons and meaningful interpretations of mathematical operations, such as ratios and proportions.
Height: A Ratio Variable
Height, as a measurable physical characteristic, falls under the category of ratio variables. It has a true zero point, representing the absence of height, and equally spaced intervals along its measurement scale. This allows us to compare heights absolutely, making statements like “Person A is twice as tall as Person B” valid.
Understanding the level of measurement for height is crucial for researchers and analysts alike. By recognizing its ratio nature, we can utilize the full range of statistical techniques and draw meaningful conclusions from height-related data. This understanding empowers us to explore and interpret the complexities of human physical attributes with greater precision.