Unveiling Factors That Widen Confidence Intervals: Sample Size, Data Variability, And Confidence Level
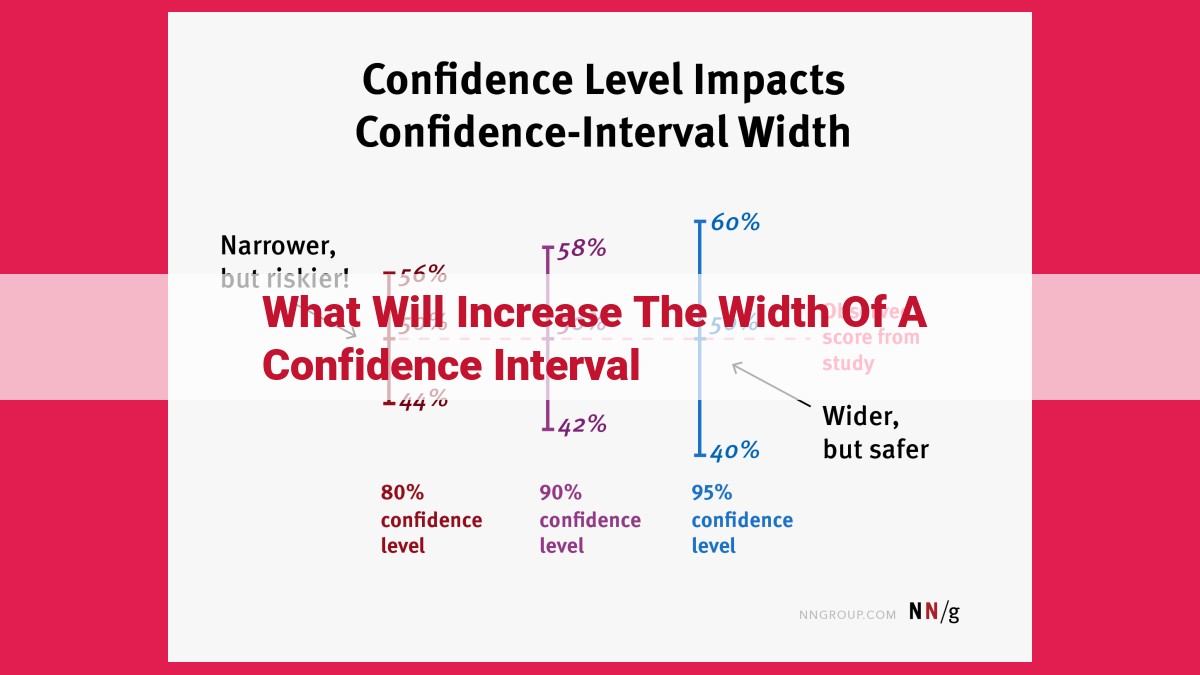
Factors that increase the width of a confidence interval include: smaller sample size because smaller samples are less representative of the population, greater variability in data as more spread-out data leads to less precise estimates, and a lower level of confidence because a higher level of confidence requires a wider interval to capture the true population parameter with greater certainty.
Factors that Increase the Width of a Confidence Interval
In the realm of statistics, confidence intervals play a crucial role in providing a range of plausible values for a population parameter. However, the width of these intervals, which represents the level of precision, is influenced by several key factors. Understanding these factors is essential for accurately interpreting and utilizing confidence intervals.
Smaller Sample Size
The size of the sample you draw from the population has a direct impact on the width of the confidence interval. Imagine taking a sample of 10 people to estimate the average height in a city. If you were to draw a second sample of 100 people, the confidence interval for the second sample would typically be narrower. This is because the larger sample provides more data points, which reduces the likelihood of extreme values and provides a more accurate representation of the population.
Greater Variability in Data
The variability of the data you collect also affects the width of the confidence interval. Variability refers to the spread or dispersion of the data. A population with a high level of variability, such as one with individuals of significantly different heights, will result in a wider confidence interval. This is because the range of possible values is larger, making it more difficult to precisely estimate the population parameter.
Lower Level of Confidence
The confidence level you choose also influences the interval’s width. A higher confidence level, such as 95%, requires a wider interval to account for the increased probability of including the true population parameter. Conversely, a lower confidence level, such as 90%, results in a narrower interval but also a higher risk of excluding the true parameter.
Understanding these factors is crucial for interpreting confidence intervals effectively. By considering the sample size, variability, and desired confidence level, you can tailor your sampling strategy to achieve the desired level of precision in your estimates.
Delving into the Impact of Sample Size on Confidence Interval Width: A Journey of Statistical Exploration
Introduction:
In the realm of statistics, confidence intervals play a crucial role in understanding the reliability of our inferences about a population. These intervals provide a range of values within which we believe the true population parameter lies, with a certain level of confidence. However, the width of these confidence intervals can vary depending on several factors, one of which is the sample size.
Unveiling the Connection between Sample Size and Confidence Interval Width:
The relationship between sample size and confidence interval width is an inverse one. Smaller sample sizes lead to wider confidence intervals, while larger sample sizes result in narrower confidence intervals. This is because a smaller sample provides less information about the population, making it more difficult to make precise estimates.
Understanding the Concepts of Sampling and Population Size:
In the context of confidence intervals, we often encounter two key concepts: sampling and population size. Sampling refers to the process of selecting a subset (or sample) from a larger group (or population) to make inferences about the entire population. Population size refers to the total number of individuals or elements in the population.
Exploring the Impact of Sample Size through an Example:
Imagine we want to estimate the average height of students in a university. If we randomly select a sample of 50 students from a population of 10,000 students, our confidence interval will be wider compared to a situation where we select a sample of 500 students. This is because the smaller sample provides less information about the true average height of the population, leading to a less precise estimate.
How Variability in Data Impacts Confidence Intervals
When we analyze data, variability is a crucial factor that influences the width of our confidence intervals. Confidence intervals help us estimate the range of values within which the true population parameter is likely to fall.
Variability refers to the spread or dispersion of data points. It indicates how much the data values differ from the mean (average). Higher variability means that the data points are more spread out, while lower variability indicates data points clustered around the mean.
Variability and Confidence Interval Width
As variability increases, the width of the confidence interval also expands. This is because greater variability suggests that the data is less predictable, making it harder to pinpoint the true population parameter with precision.
Standard Deviation, Variance, and Range
-
Standard deviation is a statistical measure that quantifies variability by calculating the average distance of data points from the mean. A higher standard deviation indicates more variability.
-
Variance is the square of the standard deviation. It provides the spread of data in squared units.
-
Range is the simplest measure of variability, calculated as the difference between the maximum and minimum data values.
Example:
Imagine a population of student exam scores. If the standard deviation of these scores is high, it means that there is a wide range of scores. Some students may score very high, while others may score very low. In this case, the width of the confidence interval for the mean score will be larger compared to a population with lower variability.
Understanding the impact of variability on confidence intervals is essential for accurate data analysis. As variability increases, the confidence interval widens, indicating less precision in estimating the true population parameter. Therefore, it’s crucial to consider the variability of data when interpreting confidence intervals and making statistical inferences.
Understanding the Inverse Relationship Between Confidence Level and Confidence Interval Width
In the realm of statistics, confidence intervals play a crucial role in helping us make inferences about a population based on a sample. The width of these intervals, however, is not fixed and can vary depending on several factors. One such factor is the confidence level.
Confidence Level and Confidence Interval: An Inverse Relationship
The confidence level represents the probability that the true population parameter lies within the calculated confidence interval. Typically, this level is expressed as a percentage, such as 95% or 99%. It’s important to note that a higher confidence level comes at the expense of a wider confidence interval.
Why the Wider Interval?
Imagine you’re conducting a survey to estimate the average height of adults in your city. If you set a high confidence level, say 99%, it means you want to be extremely confident that the true average height falls within your calculated interval. To achieve this, the sample size you need to collect would be larger than if you had chosen a lower confidence level.
The Larger Sample and Smaller Range
A larger sample provides more data points, reducing the likelihood of extreme values skewing the results. This in turn reduces the variability in your data, which is a measure of how spread out the data points are. A smaller variability results in a smaller range of possible values for the true population parameter, hence a wider confidence interval.
The Significance Level: A Related Concept
The significance level is another important concept related to confidence intervals. It represents the probability of rejecting the null hypothesis (the assumption that there is no significant difference between two populations) when it is in fact true. A lower significance level, like 0.05 or 0.01, leads to a higher confidence level. This is because a lower significance level requires stronger evidence to reject the null hypothesis, and a stronger confidence level requires a wider interval to provide that evidence.
Balancing the Confidence Level and Interval Width
In practice, choosing an appropriate confidence level depends on the desired level of uncertainty and the trade-off between accuracy and sample size. A higher confidence level provides greater certainty, but it also requires a larger sample size and a wider confidence interval. Striking the right balance is crucial for ensuring meaningful and accurate statistical inferences.