Essential Conditions For Discrete Probability Distributions In Data Analysis
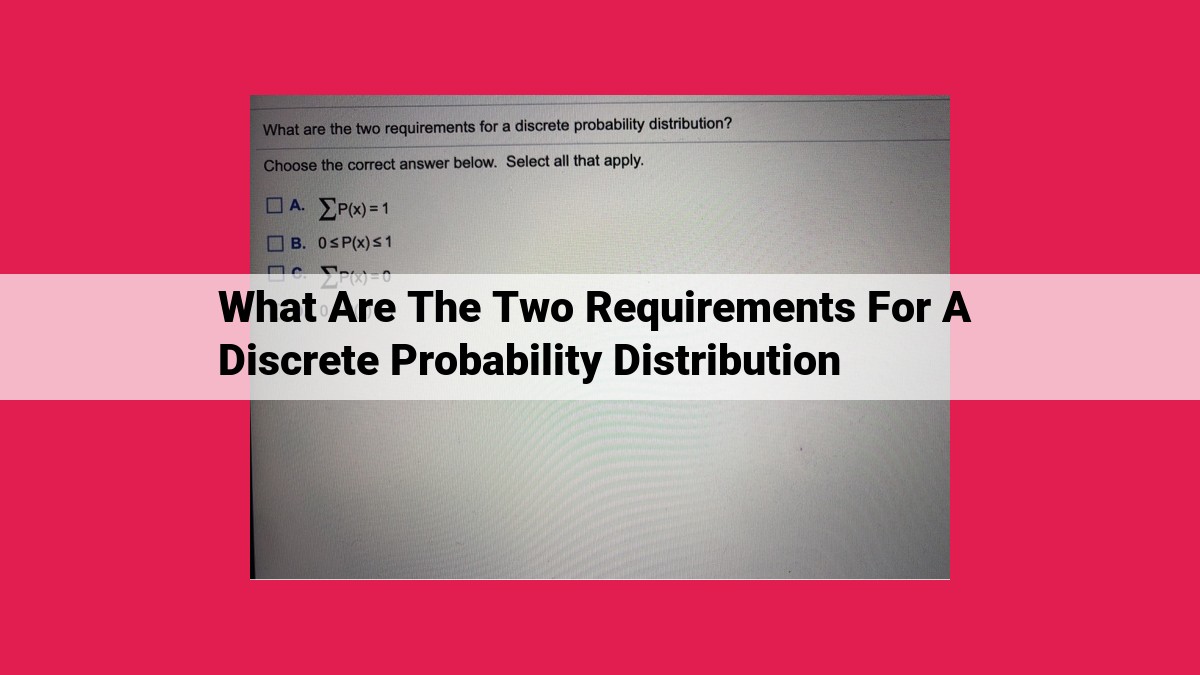
Discrete probability distributions require two conditions: the sum of probabilities across all outcomes must equal 1 (ensuring probabilities are normalized), and each individual probability must fall between 0 and 1 (indicating the absence of negative probabilities or probabilities exceeding certainty).
Step into the fascinating world of discrete probability distributions, a crucial concept in modeling random events and understanding their outcomes. These distributions paint a clear picture of the probabilities associated with specific outcomes, providing a powerful tool for analyzing uncertain situations.
Discrete probability distributions, unlike their continuous counterparts, deal with events that can only take on distinct, countable values. Imagine flipping a fair coin; it can only land on heads or tails, two discrete outcomes. Assigning probabilities to these outcomes is the essence of a discrete probability distribution.
The key characteristics of discrete probability distributions lie at the heart of their functionality. Firstly, the sum of probabilities for all possible outcomes must equal 1. This fundamental principle ensures that every possible outcome is accounted for, and the distribution forms a complete probability space.
Secondly, each individual probability value must fall between 0 and 1. These probabilities represent the likelihood of an outcome occurring, with 0 indicating impossibility and 1 indicating certainty. By adhering to this requirement, discrete probability distributions provide a meaningful representation of the probabilities associated with random events.
Understanding discrete probability distributions unlocks a world of applications in various fields. From calculating the probability of a patient recovering from an illness to predicting the outcomes of financial investments, these distributions play a pivotal role in decision-making and risk assessment.
First Requirement: Sum of Probabilities Equals 1
In the enchanting realm of probability theory, where chance encounters and unpredictable events dance, there lies a fundamental law that guides all probability distributions: the sum of all probabilities must equal 1. Just like the pieces of a perfect jigsaw puzzle, each probability within a distribution represents a portion of the whole.
Probability Axioms: The Guiding Principles
This cardinal rule stems from the very foundation of probability theory, encapsulated in the probability axioms. These axioms, like the Ten Commandments of probability, dictate the fundamental principles that govern how we assign probabilities to uncertain events.
The first axiom postulates that the probability of any event cannot be negative. After all, probability is the measure of how likely something is to happen. A negative probability would imply that an event is less than nothing likely—a logical paradox!
The second axiom declares that the probability of the entire sample space (the set of all possible outcomes) must be 1. This simply means that something must happen, even if we don’t know what it is. It’s like flipping a coin; the total probability of getting heads or tails must add up to 1, because there are no other possible outcomes.
Bayes’ Theorem: Unraveling the Conditional Connection
Enter Bayes’ Theorem, a powerful tool that allows us to calculate the conditional probability of an event occurring given the knowledge of another event. Conditional probability is crucial in real-world scenarios, such as calculating the probability of a patient having a disease based on their symptoms.
Bayes’ Theorem leverages the knowledge of prior probabilities (what we know about an event before observing any data) and posterior probabilities (what we know after incorporating new information) to refine our probability estimates.
In conclusion, the requirement that the sum of probabilities equals 1 is a cornerstone of probability theory. It ensures that our probability distributions are consistent and that the probabilities we assign to events are meaningful and accurate. Just as a well-built house rests on a sturdy foundation, the validity of probability calculations relies on this fundamental principle.
Second Requirement: Each Probability is Between 0 and 1
- Description of probability distributions and their role in assigning probabilities
- Introduction to cumulative distribution functions and probability density functions
Second Requirement: Each Probability is Between 0 and 1
In the realm of probability theory, the fundamental principle dictates that any probability distribution must satisfy certain requirements to ensure its validity and accuracy. One such crucial requirement is that each individual probability assigned must lie within the range of 0 to 1. This constraint embodies the foundational concept of probability as a measure of likelihood or occurrence.
Probability Distributions: The Guardians of Probability
Probability distributions serve as essential frameworks for assigning probabilities to possible outcomes within a given event or experiment. They provide a systematic approach to quantifying the likelihood of various scenarios, which is crucial for decision-making, risk assessment, and statistical inference.
The requirement that each probability be between 0 and 1 ensures the internal consistency of the probability distribution. A probability of 0 indicates an impossible or highly improbable event, while a probability of 1 represents an event that is certain to occur. By confining probabilities within this range, probability distributions ensure that their assigned values are meaningful and align with the principles of probability theory.
Cumulative Distribution Functions and Probability Density Functions: Unraveling the Probability Puzzle
To fully grasp the significance of this requirement, it’s essential to delve into the concepts of cumulative distribution functions (CDFs) and probability density functions (PDFs). CDFs provide a comprehensive view of the distribution by showcasing the probability of an event occurring at or below a given value. PDFs, on the other hand, represent the probability of an event taking on a specific value.
The requirement that probabilities be between 0 and 1 has profound implications for these functions. CDFs must always be non-decreasing, starting at 0 and culminating in 1 as the event’s value approaches infinity. Similarly, PDFs must be non-negative, ensuring that probabilities remain positive throughout the range of possible values.
Real-World Applications: Probability in Action
The requirement that probabilities be between 0 and 1 underpins the practical applications of discrete probability distributions in various fields. From predicting the outcome of a coin flip in statistics to assessing financial risks in finance, or modeling the spread of a disease in data science, these distributions play a pivotal role in quantifying uncertainty and making informed decisions.
Embracing the Certainty of Probability
In essence, the requirement that each probability is between 0 and 1 serves as a cornerstone of probability theory, ensuring the reliability and coherence of probability distributions. It instills confidence in the assigned probabilities, empowering us to navigate the uncertain world around us with a more informed perspective.
Applications of Discrete Probability Distributions
Discrete probability distributions play a crucial role in various real-world scenarios, providing a solid foundation for reliable probability calculations. From statistics and finance to data science, these distributions are indispensable tools for analyzing and understanding data.
In the realm of statistics, discrete probability distributions are employed to model discrete random variables, such as the number of customers visiting a store in a day or the number of accidents occurring in a city per week. These models enable statisticians to make probabilistic statements about the outcomes of experiments and draw meaningful conclusions from data.
In the realm of finance, discrete probability distributions are employed to assess the risk and uncertainty associated with financial investments. For instance, the binomial distribution is commonly used to determine the probability of a stock price increase or decrease. Moreover, discrete probability distributions are indispensable in insurance, where they are used to estimate the likelihood of events such as accidents or illness.
In the realm of data science, discrete probability distributions are employed to analyze complex datasets and identify patterns. The Poisson distribution is often used to model the number of occurrences of an event within a fixed interval, while the geometric distribution is utilized to model the number of trials required to obtain a desired outcome.
In conclusion, discrete probability distributions are powerful tools that provide the foundation for accurate probability calculations. Their applications span a wide range of fields, from statistics and finance to data science, empowering us to make informed decisions, draw meaningful conclusions, and understand the complexities of the world around us.