Unveiling The Significance Of Criterion Variables: Measuring Outcomes And Establishing Relationships
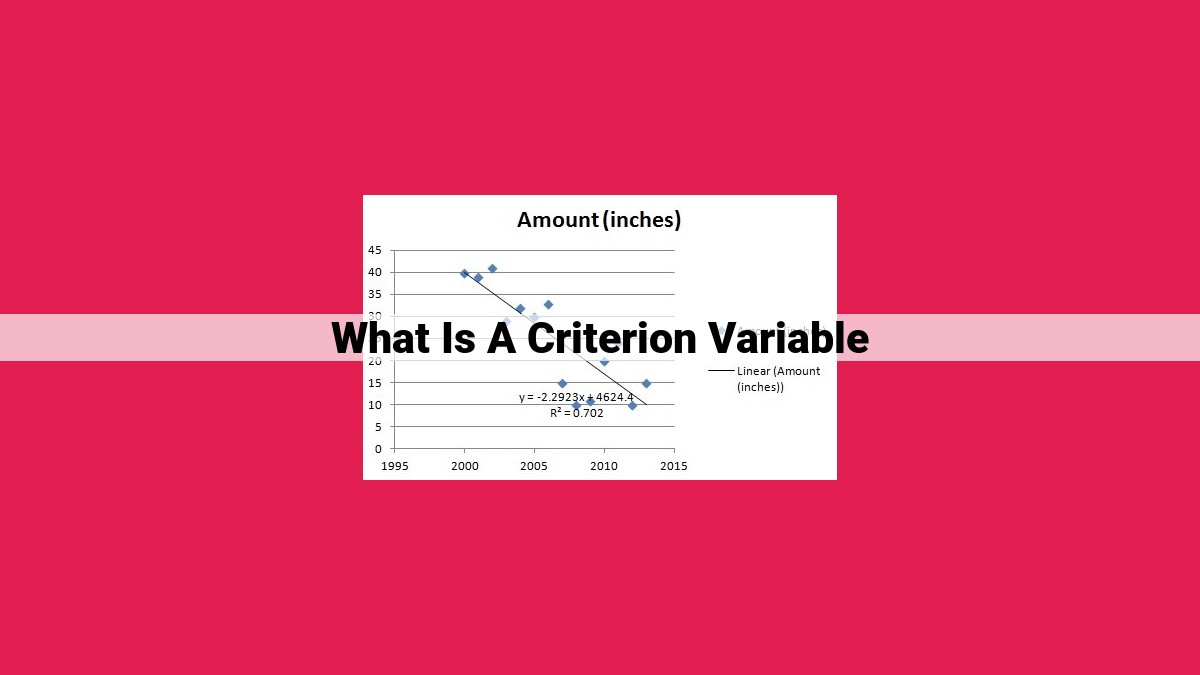
A criterion variable, also known as a dependent variable, outcome variable, or response variable, is a numerical value that measures the outcome of a phenomenon or experiment. It is used to assess the effect of independent variables and to establish relationships between variables. Criterion variables can be numerical, categorical, ordinal, or nominal, and they play a crucial role in statistical analyses, allowing researchers to measure outcomes, evaluate relationships, and make inferences. When selecting a criterion variable, factors such as the research question and the available data should be considered.
What is a Criterion Variable?
- Define a criterion variable as a dependent variable that measures the outcome of a phenomenon or experiment.
What is a Criterion Variable? A Comprehensive Guide for Beginners
In the world of research and statistical analysis, variables play a crucial role in measuring the characteristics and outcomes of phenomena. Among these variables, the criterion variable holds a special significance as the dependent variable that measures the end result of an experiment or investigation.
Defining the Criterion Variable
Simply put, a criterion variable is a variable that reflects the outcome you are interested in studying. It is the dependent variable that measures how the phenomenon being investigated affects or influences this outcome. For instance, if you are studying the effectiveness of a new teaching method, the student test scores could be your criterion variable, as they indicate the outcome or effect of the method.
Types of Criterion Variables
Criterion variables can take on different forms depending on the nature of the data being measured. The most common types include:
- Numerical: Measures that can be expressed in numerical values, such as test scores, weight, or income.
- Categorical: Measures that classify data into distinct categories, such as gender, job title, or marital status.
- Ordinal: Measures that rank data in a specific order, such as customer satisfaction ratings (very satisfied, satisfied, neutral, dissatisfied).
- Nominal: Measures that simply label or classify data without any implied order, such as names or product types.
The Importance of Criterion Variables
Criterion variables are essential for statistical analyses as they provide the basis for evaluating the effects of independent variables and making inferences. By measuring the changes or relationships between the criterion variable and other variables, researchers can understand how factors influence outcomes and draw conclusions about the phenomenon being studied.
Applications of Criterion Variables
Criterion variables find widespread applications in various research fields and statistical modeling scenarios. They are used to measure:
- Effectiveness: Efficacy of interventions or treatments (e.g., evaluating the impact of a new drug on patient recovery).
- Relationships: Association between variables (e.g., investigating the correlation between socioeconomic status and health outcomes).
- Predictive power: Ability of variables to predict future events (e.g., using past sales data to forecast future demand).
Choosing the Right Criterion Variable
Selecting the appropriate criterion variable is crucial for obtaining meaningful results. Factors to consider include:
- Research question: The variable should directly measure the outcome of interest.
- Data availability: The data should be reliable and available in a suitable format.
- Type of analysis: The choice of criterion variable will influence the statistical techniques used for analysis.
Criterion variables are indispensable in research and statistical analysis. They provide the foundation for measuring outcomes, understanding relationships, and making inferences about phenomena. By carefully selecting and interpreting the criterion variable, researchers can gain deeper insights into the world around us.
Types of Criterion Variables
In the realm of research and statistical analysis, the criterion variable takes center stage. It’s the dependent variable, the one we study to understand the impact of our independent variables. And just like a chameleon adapts to its surroundings, criterion variables come in various forms, each suited to a specific research purpose.
One common type is the numerical criterion variable. It measures an amount, quantity, or magnitude. Think of it as a continuous spectrum, where you can have any value within a certain range. For instance, in a study on the effects of fertilizer on plant growth, the height of the plants could be a numerical criterion variable.
Next, we have the categorical criterion variable. This one divides your data into distinct groups or categories. It’s like sorting your socks into piles based on color. For example, in a survey about music preferences, the genre of music could be a categorical criterion variable, with categories like “rock,” “pop,” and “classical.”
A third type is the ordinal criterion variable. It’s similar to a categorical variable, but here the categories have a natural order. It’s like ranking your favorite movies from best to worst. In a study on job satisfaction, the level of satisfaction could be an ordinal criterion variable, with categories like “very satisfied,” “satisfied,” and “dissatisfied.”
Finally, we have the nominal criterion variable. This one represents unrelated or qualitative categories. It’s like labeling your spices as “salt,” “pepper,” and “oregano.” For instance, in a study on personality types, the Myers-Briggs Type Indicator (MBTI) could be a nominal criterion variable, with categories like “introverted” and “extroverted.”
Understanding the different types of criterion variables is crucial for choosing the right one for your research question. It’s like picking the perfect key to unlock the door to valuable insights.
Understanding the Interplay between Criterion Variables and Related Concepts
The Role of Criterion Variables in Statistical Analyses
Within the realm of statistics, criterion variables play a pivotal role in assessing the outcomes of experiments and phenomena. These dependent variables stand as the measures of interest, providing valuable insights into the impact of various independent variables or treatments.
Interwoven Terms: Dependent Variables, Outcome Variables, and Beyond
The term “criterion variable” is often used interchangeably with dependent variable, emphasizing its dependence on the manipulated independent variable. However, in certain contexts, researchers may also refer to such variables as outcome variables or response variables. While these terms capture similar concepts, their usage may vary depending on the research field or context.
Endpoints and Y-Variables: Situational Distinctions
In clinical research, for instance, the term endpoint is commonly employed to denote specific health outcomes measured within a study. Similarly, in regression analyses, the Y-variable represents the dependent variable being examined. These distinctions are made to align with the specific terminologies used in these contexts.
Connecting the Dots: A Unified Understanding
Ultimately, all of these terms share a common thread: they refer to the variable measured to assess the effects of the independent variable or intervention. By understanding these interconnected concepts, researchers can navigate the statistical landscape more effectively, making informed choices in their research designs and interpretations.
The Significance of Criterion Variables in Statistical Analysis
In the realm of statistical research, criterion variables hold immense importance, serving as the key indicators that measure the outcomes of experiments and phenomena. Their primary role lies in quantifying the impact of independent variables and evaluating the relationships between variables, enabling researchers to draw meaningful conclusions.
Measuring Outcomes: A Window to the World
Criterion variables provide a direct measurement of the outcomes that researchers seek to understand. They are the dependent variables that reflect how the independent variables (manipulated by the researcher) influence the results. By examining the changes in the criterion variable, researchers can gauge the effectiveness of interventions, compare different treatments, and establish causal relationships.
Evaluating Relationships: Connecting the Dots
Statistical analyses rely heavily on criterion variables to assess relationships between variables. Statistical tests, such as correlation and regression, utilize criterion variables to determine the strength of associations and the directionality of effects. Understanding these relationships allows researchers to identify patterns, make predictions, and develop explanatory models that advance our knowledge of the world.
Making Inferences: Stepping into the Unknown
Criterion variables are crucial for drawing inferences from statistical data. By analyzing the distribution of values in the criterion variable, researchers can estimate the probability of certain outcomes and make predictions about future events. This knowledge enables them to generalize their findings to a wider population, providing valuable insights that can inform decision-making and policy development.
Applications of Criterion Variables
In the realm of statistical analysis, criterion variables play a pivotal role in illuminating the outcomes of research endeavors. They serve as the dependent variables, acting as the barometer against which the influence of independent variables is measured. Their versatility extends across a wide spectrum of research disciplines, empowering researchers to delve into the intricacies of real-world phenomena.
One compelling application of criterion variables lies in the field of medicine. Here, they are instrumental in assessing the efficacy of new treatments, evaluating patient outcomes, and monitoring disease progression. For instance, in a clinical trial, the change in a patient’s health status or the duration of their illness might be designated as criterion variables to gauge the effectiveness of an experimental drug.
Criterion variables also find their place in social science research. They facilitate investigations into human behavior, attitudes, and perceptions. Consider a psychologist exploring the impact of social media on mental well-being. The criterion variable in this scenario could be the level of anxiety or depression reported by participants, providing insights into the potential consequences of social media use.
Beyond the realms of medicine and social science, criterion variables extend their reach into business and economics. They are indispensable for gauging the success of marketing campaigns, assessing customer satisfaction, and forecasting financial performance. For instance, in a marketing experiment, the number of products sold or the click-through rate on an advertisement might serve as criterion variables, shedding light on the effectiveness of different strategies.
In summary, criterion variables are the cornerstone of statistical analysis, enabling researchers to quantify the outcomes of their investigations. Their applications span a vast array of fields, from medicine and social science to business and economics. By unraveling the intricate relationships between independent and dependent variables, criterion variables empower researchers to draw meaningful conclusions, make informed decisions, and advance our understanding of the world around us.
How to Choose the Right Criterion Variable
Selecting the appropriate criterion variable is crucial for successful statistical analyses and research outcomes. Several factors necessitate consideration when making this choice:
Type of Research Question: The research question’s nature directly influences the selection of a criterion variable. For instance, if the goal is to predict an outcome, a numerical criterion variable (e.g., sales volume) may be optimal. Conversely, if the objective is to categorize participants based on their response, a categorical criterion variable (e.g., customer satisfaction) would be more suitable.
Nature of Data Available: The data available for analysis also plays a crucial role in selecting the criterion variable. If the data is quantitative (i.e., numerical), then numerical criterion variables can be used. If the data is qualitative (i.e., non-numerical), then categorical criterion variables are more appropriate.
Measurement Scale: The measurement scale of the variable indicates its level of precision. Nominal criterion variables have no inherent order, while ordinal criterion variables have a specific order but no equal intervals between values. Interval criterion variables have equal intervals between values but no true zero point, while ratio criterion variables have equal intervals and a true zero point. The selection of the measurement scale depends on the nature of the data.
Reliability and Validity: The criterion variable should be reliable, meaning consistent measurements across multiple observations. It should also be valid, meaning it accurately measures the intended concept. These qualities ensure that the results derived from the analysis are meaningful and accurate.
Practical Considerations: Practical considerations such as availability of data, cost of data collection, and time constraints may also influence the choice of the criterion variable. Researchers must balance these factors to select a variable that meets their research goals while being feasible to implement.
By carefully considering these factors, researchers can ensure that they select the most appropriate criterion variable for their research objectives. This choice lays the foundation for robust statistical analyses and meaningful interpretations of the results.