Create Standard Curves In Excel: A Comprehensive Guide For Concentration Determination
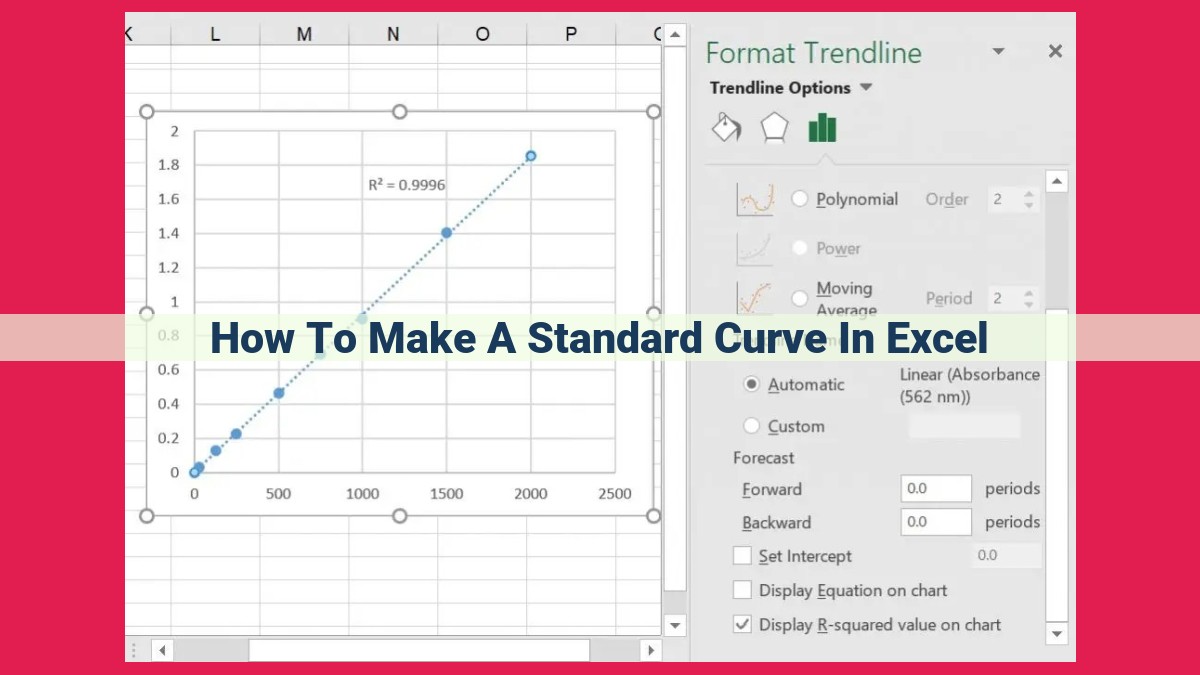
Creating a standard curve in Excel involves preparing data with concentrations and responses, plotting them in a scatter plot, and adding a linear regression trendline. The slope represents the rate of change in response with concentration, while the y-intercept indicates the response at zero concentration. The R-squared value measures the linear relationship strength. By measuring an unknown sample’s response and using the trendline equation, its concentration can be determined. Troubleshooting residuals ensures data accuracy by identifying outliers or errors.
A Guide to Crafting Standard Curves in Excel: Unveiling the Secrets of Concentration Determination
In the realm of chemistry and biochemistry, standard curves hold immense significance as they empower scientists to accurately determine the concentrations of unknown samples. Armed with a spectrophotometer or other analytical instrument, researchers can measure the response (e.g., absorbance, fluorescence) of samples containing varying concentrations of the analyte (substance of interest). To decipher this data and establish a reliable relationship between response and concentration, we harness the power of linear regression.
What is a Standard Curve?
A standard curve is a graphical representation that depicts the correlation between the response of a series of known concentrations of an analyte and their corresponding concentrations. By plotting the known concentrations along the x-axis and the responses along the y-axis, a linear trendline can be fitted to the data points, establishing a mathematical relationship that enables us to determine the concentration of an unknown sample based on its measured response.
Crafting Standard Curves in Excel
To construct a standard curve in Excel, follow these steps:
-
Prepare Your Data: Measure the responses of the known concentrations and meticulously record both the concentrations and responses in an Excel spreadsheet.
-
Plot the Standard Curve: Create a scatter plot with the concentrations as the x-axis values and the responses as the y-axis values.
-
Add Linear Regression: Insert a linear regression trendline to the scatter plot. The trendline equation will appear in the chart, providing a mathematical representation of the relationship between concentration and response.
Creating a Standard Curve in Excel: A Step-by-Step Guide
In the realm of scientific analysis, standard curves are essential tools for determining the concentrations of unknown samples. By creating a linear regression model that relates known concentrations to their corresponding responses, we can use these curves to predict the concentrations of new samples based on their responses.
With the help of Microsoft Excel, this process becomes straightforward and accessible. Let’s dive into the steps involved in crafting a standard curve in Excel:
1. Prepare Your Data
Before embarking on our Excel adventure, we need to obtain two sets of data: concentrations and responses. These values represent the known concentrations of our standards and the corresponding responses or measurements we obtain from them. Once we have these values, we can input them into an Excel spreadsheet.
2. Plot the Standard Curve
With our data ready, we can create a scatter plot that visually represents the relationship between concentrations and responses. To do this, select the concentration values as the x-axis and the response values as the y-axis. Excel will generate a scatter plot that plots each concentration-response pair.
3. Add Linear Regression
Now, it’s time to fit a linear regression trendline to our scatter plot. A trendline is a line that summarizes the linear relationship between two variables. By adding a linear trendline, we can determine the slope and y-intercept of the line, which are crucial for interpreting the curve.
To add a trendline, select your scatter plot and click on the “Add Trendline” option in the “Chart Design” tab. Choose “Linear” as the trendline type and ensure that the “Display Equation on Chart” option is checked. This will display the linear regression equation on your chart, which will be in the form of y = mx + b
, where:
y
is the predicted responsex
is the concentrationm
is the slopeb
is the y-intercept
Interpreting the Linear Regression: Unraveling the Insights
The linear regression provides valuable insights into the relationship between the concentration of your samples and their responses. By discerning the slope, y-intercept, and correlation coefficient, you can glean essential information about this relationship.
Slope: The Rate of Change
The slope, denoted by “m,” represents the rate at which the response changes as the concentration increases. A positive slope indicates that as concentration rises, the response also increases. Conversely, a negative slope suggests that the response decreases with increasing concentration. The magnitude of the slope quantifies this rate of change, giving you a numerical understanding of how responsive your system is to changes in concentration.
y-Intercept: The Baseline Response
The y-intercept, “b,” is the response when the concentration is zero. This value represents the baseline response of your system, independent of any concentration effects. If the y-intercept is significant, it indicates that your system has a non-zero response even in the absence of the measured analyte.
Correlation Coefficient (R-squared): A Measure of Linearity
The correlation coefficient, often denoted as “R^2,” quantifies the strength of the linear relationship between concentration and response. It ranges from 0 to 1, with higher values indicating a stronger linear correlation. A value close to 1 suggests that the data points conform closely to a straight line, while a value near 0 implies a weak or non-linear relationship. This metric assists in assessing the reliability of your standard curve for predicting sample concentrations.
Creating a Standard Curve: Your Guide to Unlocking Concentration Secrets with Excel
In the realm of scientific research and analytical chemistry, understanding sample concentrations is paramount. Enter the standard curve, a powerful tool that helps you determine the concentration of unknown samples. It’s like having a trusty roadmap that leads you to the precise answer.
Unveiling the Standard Curve
A standard curve is a graphical representation of the relationship between known concentrations of a substance and their corresponding responses, usually measured using an instrument like a spectrophotometer. This relationship is often linear, forming a straight line.
Crafting a Standard Curve in Excel
-
Data Preparation: Measure sample responses and record the corresponding concentrations in Excel. This is the foundation of your curve.
-
Plotting the Canvas: Create a scatter plot with concentration on the x-axis and response on the y-axis. This visualization will help you see the pattern.
-
Linear Regression: The Guiding Light: Add a linear regression trendline to the scatter plot. This line will be your guide, showing you the overall trend.
Interpreting the Linear Regression
-
Slope: The Rate of Change: The slope of the trendline represents the change in response with increasing concentration.
-
y-Intercept: The Starting Point: The y-intercept indicates the response when the concentration is zero, a crucial piece of information.
-
Correlation Coefficient (R-squared): The Strength of the Relationship: This value measures the strength of the linear relationship between concentration and response. A higher R-squared value indicates a stronger correlation.
Using the Standard Curve: Unveiling the Unknown
With your standard curve in place, you’re ready to unlock the secrets of unknown samples. Simply measure the response of an unknown sample and use the trendline equation to calculate its concentration.
A Word of Caution: Stay Within the Standard Curve’s Embrace
It’s essential to ensure that the unknown sample’s concentration falls within the range of the standard curve. This ensures that the calculated concentration is reliable. If the unknown sample’s concentration is outside the curve’s range, you may need to prepare a new standard curve with a wider concentration range.
Troubleshooting Residuals: Identifying Anomalies
Residuals are the differences between the observed responses and those predicted by the trendline. Large residuals may indicate outliers or errors in your data. If you encounter significant residuals, investigate potential error sources and make necessary corrections.
Troubleshooting Residuals: Pinpoint Errors in Your Standard Curve
After creating your standard curve, it’s crucial to examine the residuals—the difference between observed and predicted responses. These can reveal discrepancies in the data and help you troubleshoot potential errors.
Residuals as Red Flags
Large residuals indicate data deviations from the trendline. They can signal outliers—data points that significantly differ from the curve’s pattern. Outliers may stem from measurement errors, sample contamination, or other experimental variables.
Investigating the Cause
If you encounter large residuals, investigate the underlying causes. Conduct a thorough review of your data entry, verify your experimental setup, and consider potential sources of contamination or error.
Corrective Measures
If errors are identified, correct the data or exclude the affected points. This ensures that your standard curve is based on accurate and reliable measurements.
By carefully examining residuals, you can enhance the precision of your standard curve and minimize the impact of experimental errors. This will lead to more accurate concentration calculations for your unknown samples.