Anova Hypothesis Testing: Unraveling Group Differences With Statistical Significance
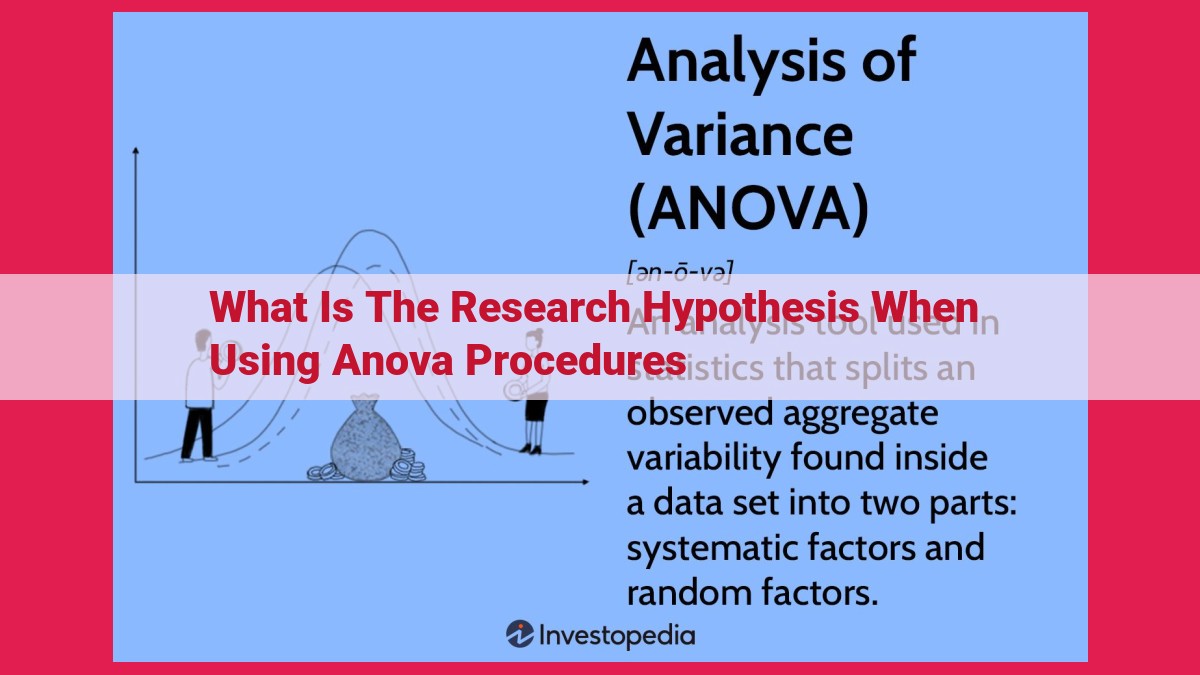
In ANOVA procedures, the research hypothesis is a statement about the significance of the difference between the means of two or more groups. The null hypothesis states that there is no significant difference, while the alternative hypothesis states that there is a significant difference. The hypothesis testing involves comparing the observed F-statistic with the critical F-value to determine whether the null hypothesis should be rejected in favor of the alternative hypothesis.
Understanding Research Hypotheses: A Comprehensive Guide
In the realm of research, hypotheses serve as the foundation upon which we test our theories and draw conclusions. They are informed predictions about the outcome of our studies and guide our data collection and analysis.
Definition of Research Hypothesis
A research hypothesis is a precise statement that predicts the relationship between two or more variables. It is an educated guess that researchers formulate based on existing knowledge and theories.
Types of Research Hypotheses
There are two main types of research hypotheses:
- Null Hypothesis (H0): States that there is no significant difference or relationship between the variables being studied.
- Alternative Hypothesis (Ha): States that there is a significant difference or relationship between the variables being studied.
Importance of Research Hypotheses
Hypotheses are crucial for several reasons:
- They establish a clear goal for the research and focus the investigation.
- They prevent bias by forcing researchers to make specific predictions before collecting data.
- They provide a benchmark against which to evaluate the results of the study.
By carefully formulating and testing hypotheses, researchers can draw informed conclusions about the phenomena they are investigating and contribute to the advancement of knowledge.
Hypothesis Testing in ANOVA: Uncovering Hidden Truths
In the realm of research, hypothesis testing is like a detective solving a mystery. In the case of ANOVA (Analysis of Variance), the detective is investigating whether a significant difference exists among multiple groups. Here’s how this intriguing process unfolds:
Null Hypothesis: Stating a Lack of Difference
The null hypothesis (H0) is the default assumption, stating that there is no significant difference among the group means. It’s like the detective saying, “I suspect that all the suspects are innocent, with no significant differences in their guilt.”
Alternative Hypothesis: Asserting a Significant Difference
In contrast, the alternative hypothesis (Ha) asserts that there is a significant difference among the group means. The detective is now saying, “I believe that at least one of the suspects is guilty and stands out from the others.”
The researcher must choose between these two competing hypotheses based on the p-value, which quantifies the likelihood that the observed differences could have occurred by chance alone. A low p-value (typically below 0.05) supports the alternative hypothesis and suggests a significant difference.
By comparing the null and alternative hypotheses, ANOVA helps researchers draw informed conclusions about the presence or absence of meaningful differences among groups. It’s an essential tool for uncovering hidden truths and advancing our understanding of various phenomena.
Unveiling the Effects in ANOVA: Unraveling the Dance of Variables
In the realm of statistics, ANOVA (Analysis of Variance) plays a pivotal role in deciphering the intricate relationships between variables. When we conduct an ANOVA, we seek to understand how different factors or variables influence the outcome of an experiment. Among the key concepts to grasp are the main effect and interaction effect, which provide insights into the behavior of these variables.
Main Effect: A Solo Performance of a Variable
Imagine you are testing the effect of different fertilizers on plant growth. You have four different fertilizers (A, B, C, and D) and randomly assign them to different groups of plants. After a period of time, you measure the growth of each plant.
The main effect of fertilizer refers to the overall difference in plant growth caused by the different fertilizers. By comparing the average growth of plants in each fertilizer group, you can determine which fertilizer is most effective on average.
Interaction Effect: A Variable’s Influence in Harmony or Conflict
But what if the effect of fertilizer is not consistent across different types of plants? This is where the interaction effect comes into play.
Suppose you have two types of plants: Type X and Type Y. You discover that Fertilizer A promotes growth in Type X plants but hinders growth in Type Y plants, while Fertilizer B has the opposite effect. This is an example of an interaction effect.
The interaction effect reveals that the effect of fertilizer is conditional on the type of plant. In other words, the behavior of one variable (fertilizer) is influenced by the other variable (plant type).
Unraveling the Dynamic Interplay of Variables
Main effects and interaction effects offer valuable insights into the complex interactions between variables. By understanding these concepts, researchers can better interpret the results of their experiments and uncover the underlying patterns that shape the world around us.
In the context of our plant growth experiment, the main effect of fertilizer tells us which fertilizer is most effective overall. However, the interaction effect reveals that the effectiveness of a fertilizer can vary depending on the specific plant type. This knowledge is crucial for making informed decisions about which fertilizers to use for different crops.
So, next time you encounter ANOVA, remember to pay attention not only to the main effects but also to the interaction effects. These two concepts will guide you towards a deeper understanding of the intricate relationships that shape the world of statistics.
Omnibus and Post Hoc Tests: Delving into Statistical Precision
When conducting an analysis of variance (ANOVA), two types of tests play crucial roles in pinpointing the underlying patterns in your data: omnibus tests and post hoc tests.
Omnibus Tests: The Overall Picture
Think of an omnibus test as the first pass through your data. It provides a comprehensive assessment, determining whether there are any statistically significant differences among the means of your groups. If the omnibus test yields a significant result, it indicates that at least one of your groups is different from the others.
Post Hoc Tests: Unearthing Specific Differences
Once you determine that there are significant differences, it’s time for the detective work. Post hoc tests delve into your data to pinpoint the exact groups that differ from one another. These tests help you identify which variables and interactions are responsible for the observed differences.
Omnibus vs. Post Hoc: Combining Power and Refinement
Omnibus tests provide a broad overview, while post hoc tests offer a detailed examination. Combining these tests gives you a powerful and precise understanding of your data:
- Omnibus tests rule out random chance as the explanation for significant differences.
- Post hoc tests identify the specific sources of these differences.
Together, omnibus and post hoc tests provide a comprehensive statistical picture, helping you draw valid conclusions about the relationships within your data.
Understanding the Power and Effect Size in Statistical Analysis
In the realm of statistical analysis, two important concepts that guide the interpretation of results are power and effect size. These metrics provide valuable insights into the strength and significance of observed differences in research data.
Power: Assessing the Likelihood of Detecting Differences
Power refers to the probability of correctly rejecting a null hypothesis when it is actually false. In other words, it measures the ability of a statistical test to detect a real difference between groups or conditions.
A high power value indicates that the test is more likely to find a significant difference when one exists. This is crucial because it helps researchers avoid false negatives, or the failure to detect a difference that is truly present.
Effect Size: Quantifying the Magnitude of Differences
Effect size measures the strength or magnitude of the observed differences between groups. It indicates how large or noticeable the differences are, regardless of statistical significance.
Effect size is often expressed as a standardized measure that can be compared across different studies and contexts. Common effect size indices include Cohen’s d for comparing means and partial eta squared for comparing variances.
The Interplay of Power and Effect Size
Power and effect size are closely related concepts. A study with high power and a large effect size is more likely to produce meaningful and reliable results. Conversely, a study with low power and a small effect size may fail to detect real differences, leading to inconclusive or misleading findings.
Researchers should carefully consider both power and effect size when designing and interpreting statistical analyses. A balance between these two metrics is essential to ensure that studies are not only statistically significant but also practically meaningful.